Wisdom of Crowds: An Empirical Study of Ensemble-Based Feature Selection Strategies
AI 2015: ADVANCES IN ARTIFICIAL INTELLIGENCE(2015)
摘要
The accuracy of feature selection methods is affected by both the nature of the underlying datasets and the actual machine learning algorithms they are combined with. The role these factors have in the final accuracy of the classifiers is generally unknown in advance. This paper presents an ensemble-based feature selection approach that addresses this uncertainty and mitigates against the variability in the generalisation of the classifiers. The study conducts extensive experiments with combinations of three feature selection methods on nine datasets, which are trained on eight different types of machine learning algorithms. The results confirm that the ensemble based approaches to feature selection tend to produce classifiers with higher accuracies, are more reliable due to decreased variances and are thus more generalisable.
更多查看译文
关键词
Ensemble feature selection,Dimensionality reduction,Machine learning,Classification,Data mining,Ensemble classifiers
AI 理解论文
溯源树
样例
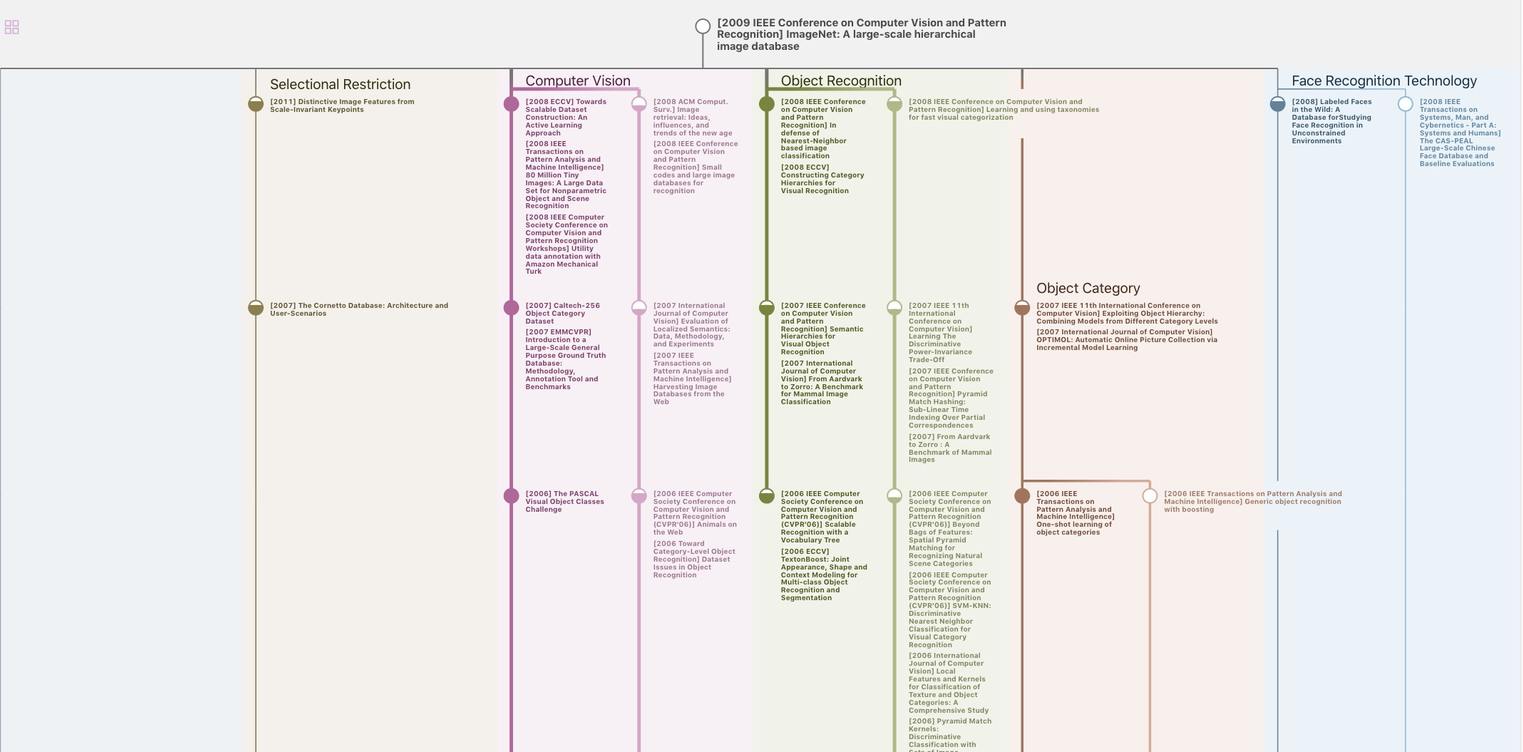
生成溯源树,研究论文发展脉络
Chat Paper
正在生成论文摘要