Investigating boredom and engagement during writing using multiple sources of information: the essay, the writer, and keystrokes.
LAK(2016)
摘要
Writing training systems have been developed to provide students with instruction and deliberate practice on their writing. Although generally successful in providing accurate scores, a common criticism of these systems is their lack of personalization and adaptive instruction. In particular, these systems tend to place the strongest emphasis on delivering accurate scores, and therefore, tend to overlook additional indices that may contribute to students' success, such as their affective states during writing practice. This study takes an initial step toward addressing this gap by building a predictive model of students' affect using information that can potentially be collected by computer systems. We used individual difference measures, text indices, and keystroke analyses to predict engagement and boredom in 132 writing sessions. The results suggest that these three categories of indices were successful in modeling students' affective states during writing. Taken together, indices related to students' academic abilities, text properties, and keystroke logs were able classify high and low engagement and boredom in writing sessions with accuracies between 76.5% and 77.3%. These results suggest that information readily available in writing training systems can inform affect detectors and ultimately improve student models within intelligent tutoring systems.
更多查看译文
关键词
Intelligent Tutoring Systems,Natural Language Processing,stealth assessment,corpus linguistics,writing
AI 理解论文
溯源树
样例
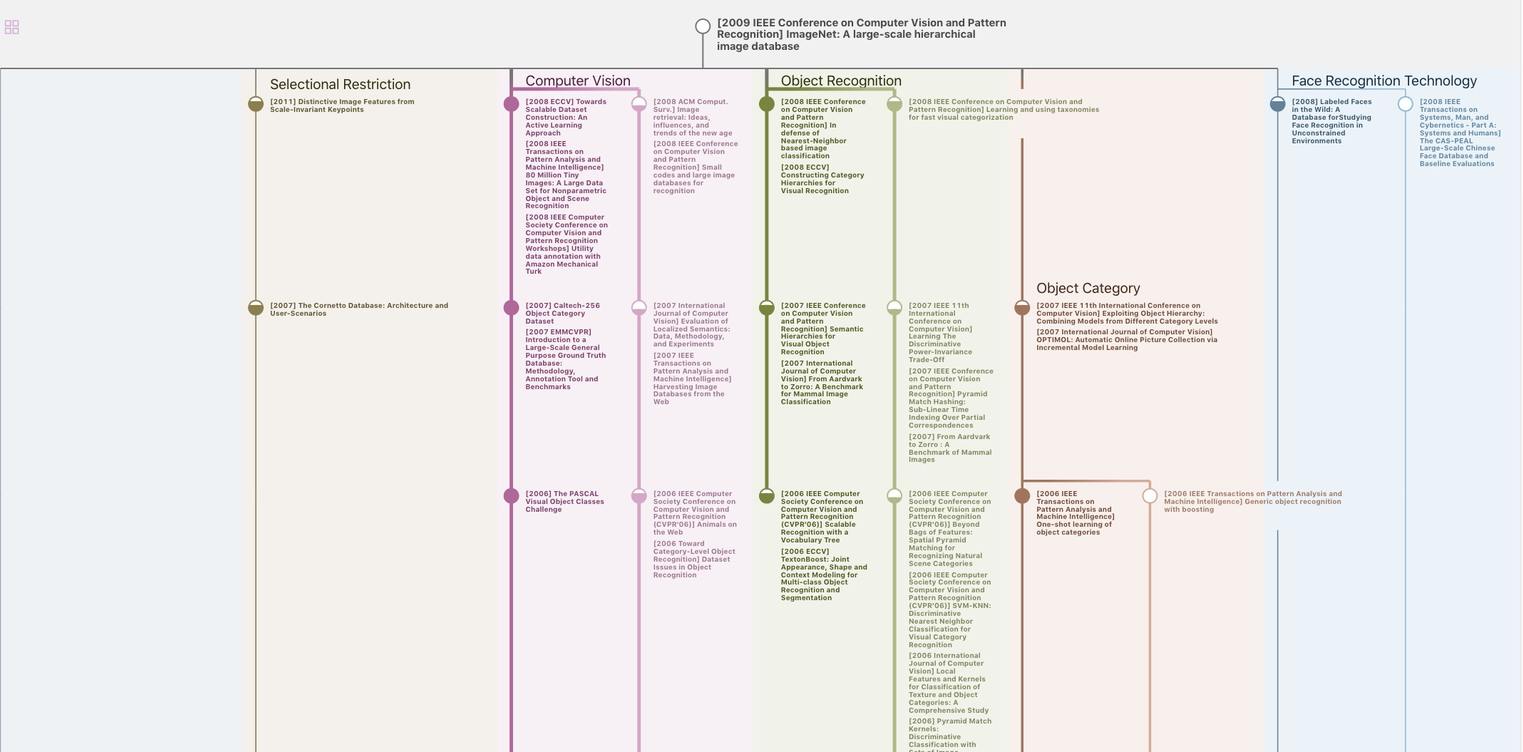
生成溯源树,研究论文发展脉络
Chat Paper
正在生成论文摘要