Privacy-Preserving Dual Splitting Distributed Optimization With Application To Load Flattening In California
2015 54TH IEEE CONFERENCE ON DECISION AND CONTROL (CDC)(2015)
摘要
This article presents a dual splitting technique for a class of strongly convex optimization problems whose constraints are block-wise independent. The average-based input in the objective is the only binding element. A dual splitting strategy enables the design of distributed and privacy preserving algorithms. Theoretical convergence bounds and numerical experiments show this method successfully applies to the problem of charging electric devices so as to even out the daily energy demand in California. The solution we provide is a privacy enforced algorithm readily implementable in a network of smart electric vehicle chargers. It can reach any arbitrary precision for the common optimization goal while relying on randomly perturbed information at the agent level. We show that, provided the community is large enough, an averaging effect enables the group to learn its global optimum faster than individual information is leaked. A limited number of messages are sent out in the distributed implementation which prevents adversary statisticians from having low theoretical Mean Square Errors for their estimates.
更多查看译文
关键词
Privacy,Gradient methods,Convergence,Algorithm design and analysis,Stochastic processes,Minimization
AI 理解论文
溯源树
样例
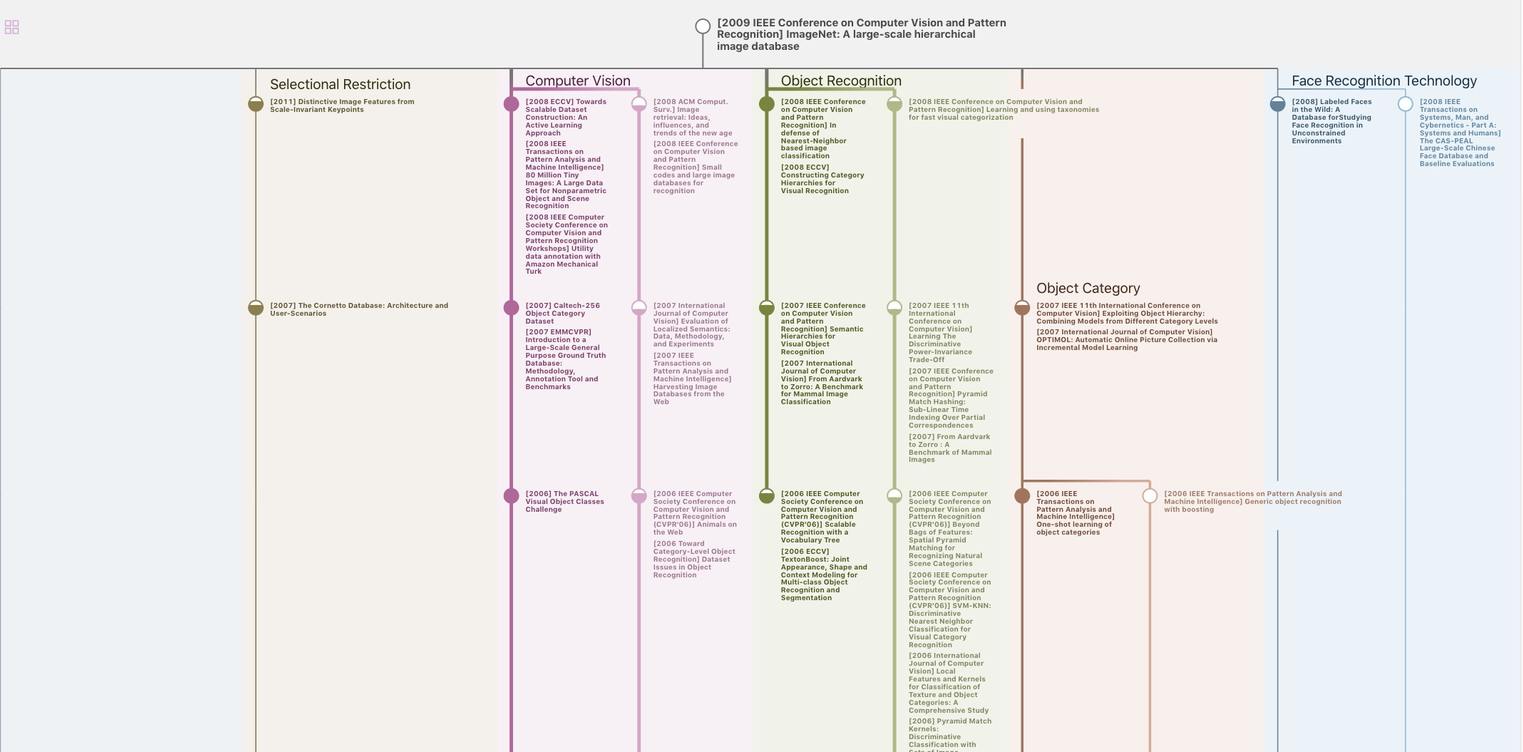
生成溯源树,研究论文发展脉络
Chat Paper
正在生成论文摘要