Combining view-based pose normalization and feature transform for cross-pose face recognition
ICB(2015)
摘要
Automatic face recognition across large pose changes is still a challenging problem. Previous solutions apply a transform in image space or feature space for normalizing the pose mismatch. For feature transform, the feature vector extracted on a probe facial image is transferred to match the gallery condition with regression models. Usually, the regression models are learned from paired gallery-probe conditions, in which pose angles are known or accurately estimated. The solution based on image transform is able to handle continuous pose changes, yet the approach suffers from warping artifacts due to misalignment and self-occlusion. In this work, we propose a novel approach, which combines the advantage of both methods. The algorithm is able to handle continuous pose mismatch in gallery and probe set, mitigating the impact of inaccurate pose estimation in feature-transform-based method. We evaluate the proposed algorithm on the FERET face database, where the pose angles are roughly annotated. Experimental results show that our proposed method is superior to solely image/feature transform methods, especially when the pose angle difference is large.
更多查看译文
关键词
face recognition,feature extraction,pose estimation,regression analysis,vectors,cross-pose face recognition,feature transform,feature vector extraction,feature-transform-based method,pose estimation,pose mismatch normalization,regression model,view-based pose normalization,
AI 理解论文
溯源树
样例
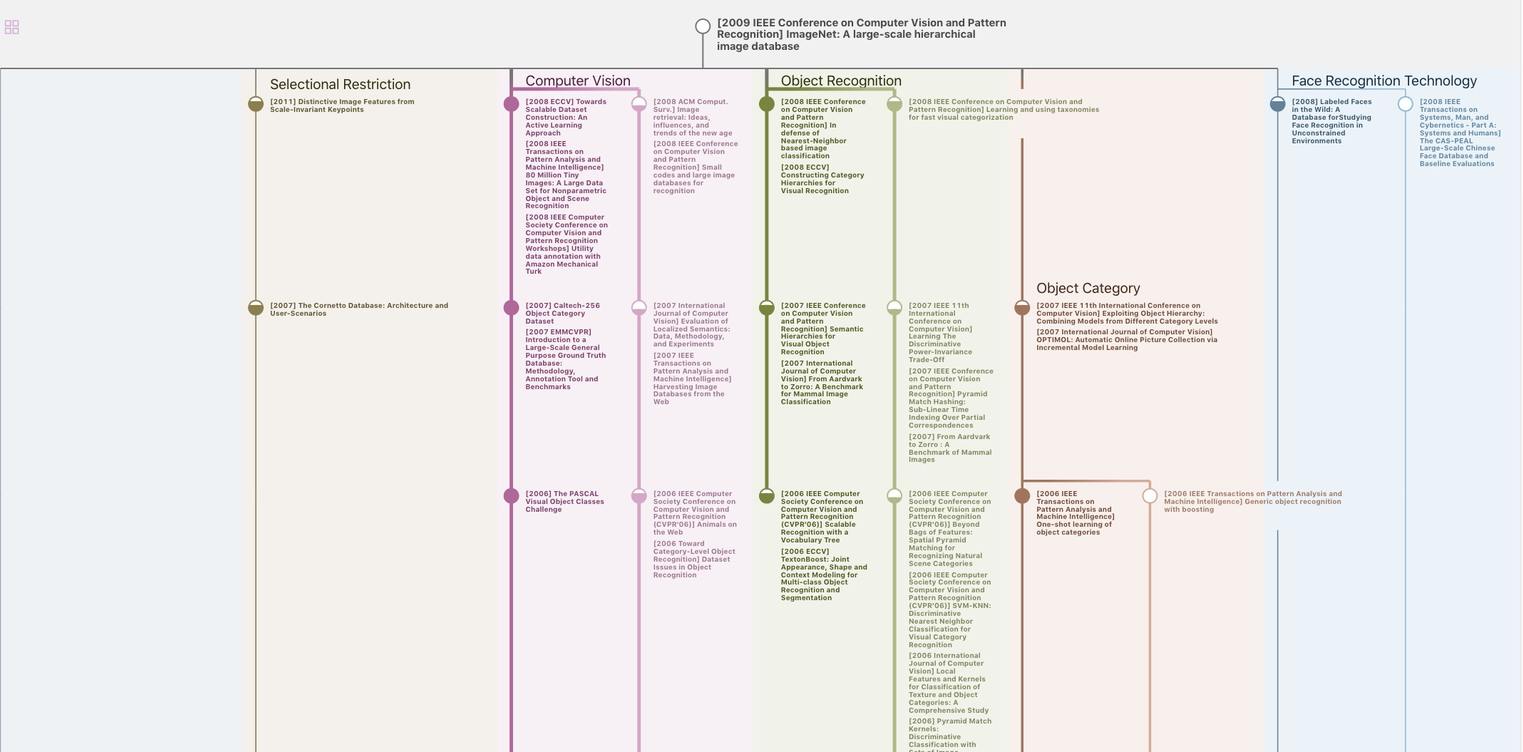
生成溯源树,研究论文发展脉络
Chat Paper
正在生成论文摘要