Large-Scale Speaker Search Using Plda On Mismatched Conditions
2015 IEEE International Conference on Acoustics, Speech and Signal Processing (ICASSP)(2015)
摘要
Recent work reported on fast speaker search over large speech data corpora has focused on using locality sensitive hashing (LSH) search with hashing functions approximating i-vector based cosine distances (CosDist) for model comparisons. Because of the superior performance of probabilistic linear discriminant analysis (PLDA) model reported on speaker identification (SID) in recent years, in this paper we focus on using PLDA for fast speaker search. It is challenging to approximate PLDA well with simple hashing functions, resulting in difficulty to combine it with LSH search. As an alternative, we adopt a clustering-based pruning strategy to speed up PLDA search. Our results show the strategy can significantly speed up search with minimal performance loss. Another focus of this work is on PLDA model adaptation to mismatched conditions under which the fast search runs. The technique we adopt to adapt the PLDA model is based on the LDA adaptation method reported in [1], primarily adapting the LDA transform. Our results show this adaptation improves PLDA performance significantly (over 25% relative) on data collected in different conditions. Our speed-up experiments running with adapted LDA show that gains from the adapted PLDA are retained after the speed-up.
更多查看译文
关键词
speaker search,I-vectors,PLDA,cosine distance
AI 理解论文
溯源树
样例
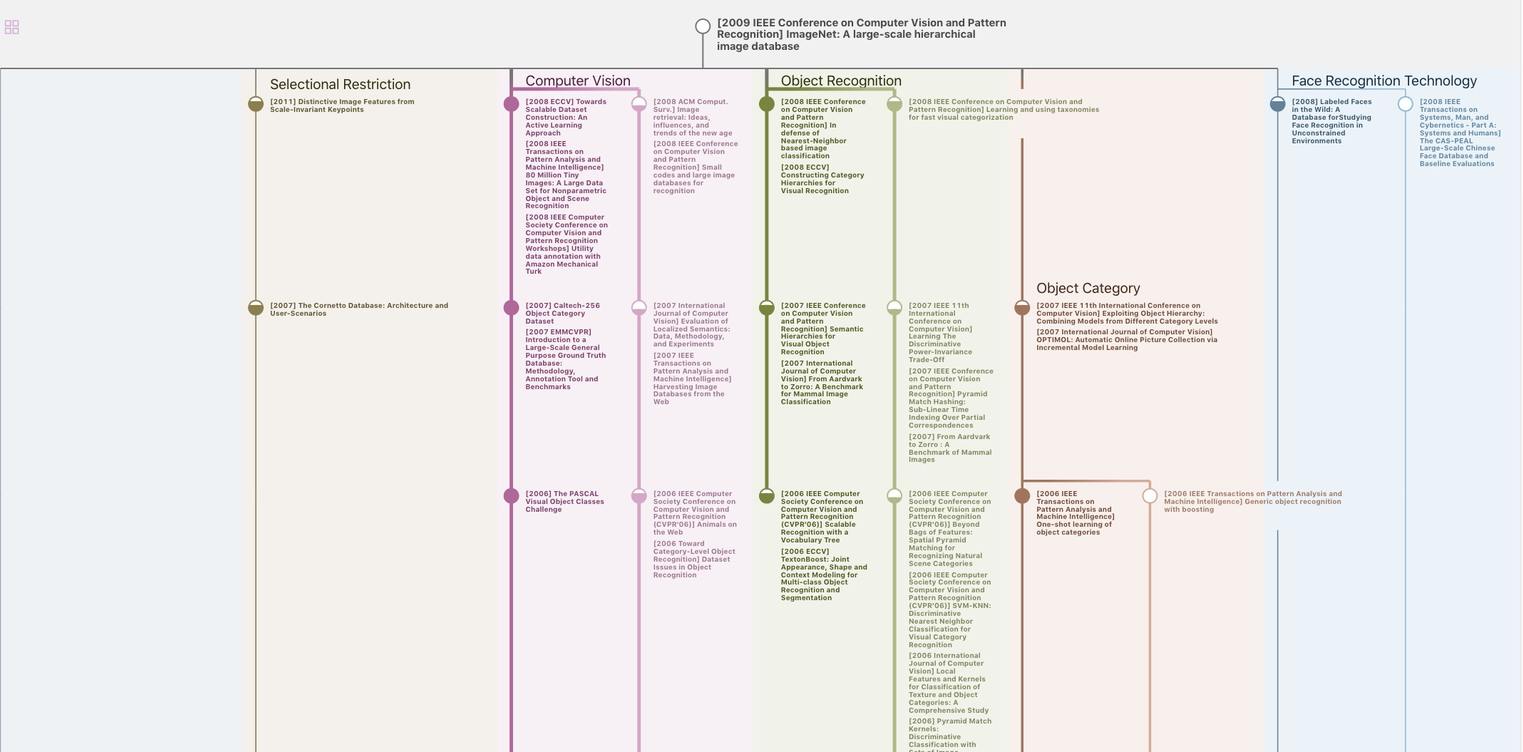
生成溯源树,研究论文发展脉络
Chat Paper
正在生成论文摘要