Contextual Spoken Language Understanding Using Recurrent Neural Networks
2015 IEEE International Conference on Acoustics, Speech and Signal Processing (ICASSP)(2015)
摘要
We present a contextual spoken language understanding (contextual SLU) method using Recurrent Neural Networks (RNNS). Previous work has shown that context information, specifically the previously estimated domain assignment, is helpful for domain identification. We further show that other context information such as the previously estimated intent and slot labels are useful for both intent classification and slot filling tasks in SLU. We propose a step-n-gram model to extract sentence-level features from RNNS, which extract sequential features. The step-n-gram model is used together with a stack of Convolution Networks for training domain/intent classification. Our method therefore exploits possible correlations among domain/intent classification and slot filling and incorporates context information from the past predictions of domain/intent and slots. The proposed method obtains new state-of-the-art results on ATIS and improved performances over baseline techniques such as conditional random fields (CRFS) on a large context-sensitive SLU dataset.
更多查看译文
关键词
Recurrent Neural Networks,Convolution Networks,Spoken Language Understanding
AI 理解论文
溯源树
样例
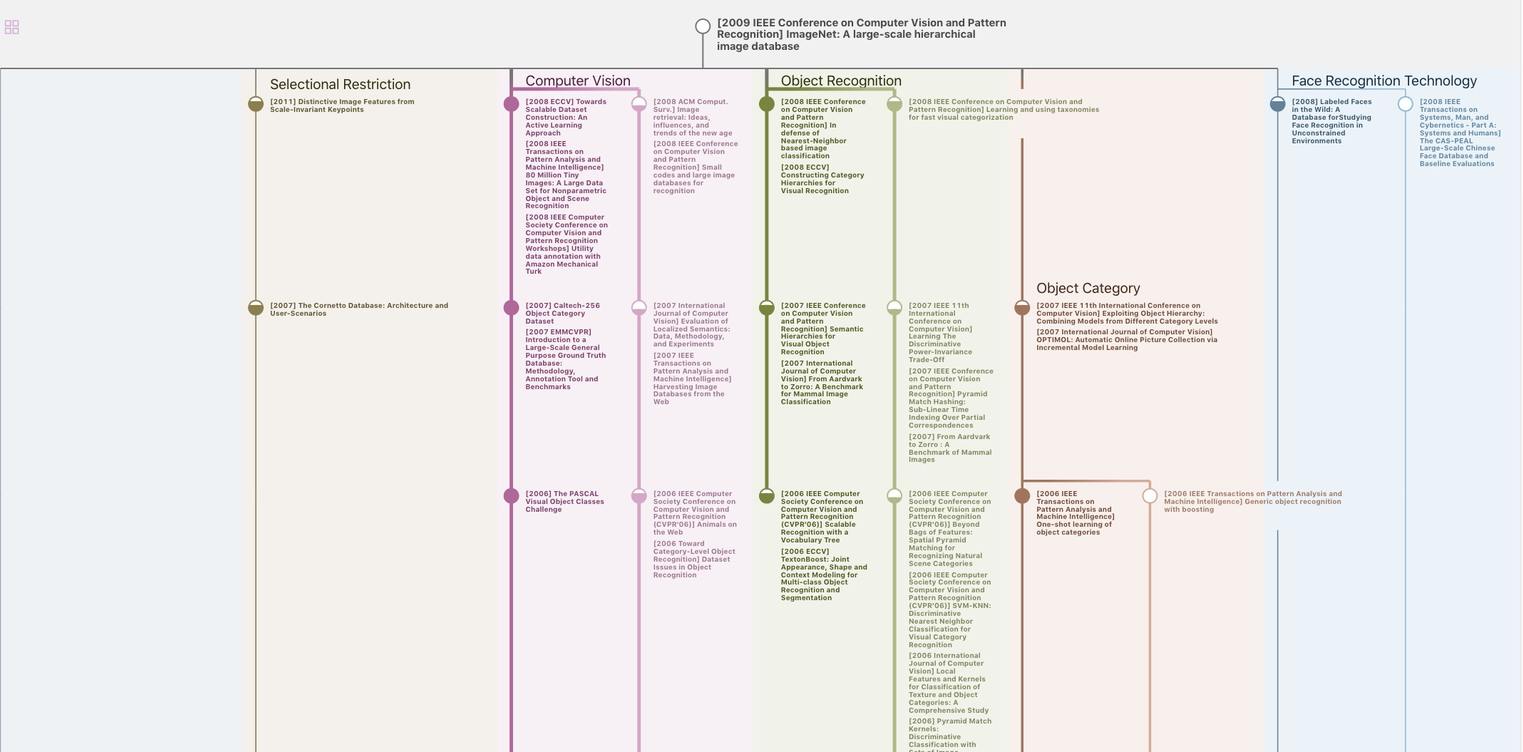
生成溯源树,研究论文发展脉络
Chat Paper
正在生成论文摘要