Effective semantic pixel labelling with convolutional networks and Conditional Random Fields
IEEE Computer Society Conference on Computer Vision and Pattern Recognition Workshops(2015)
摘要
Large amounts of available training data and increasing computing power have led to the recent success of deep convolutional neural networks (CNN) on a large number of applications. In this paper, we propose an effective semantic pixel labelling using CNN features, hand-crafted features and Conditional Random Fields (CRFs). Both CNN and hand-crafted features are applied to dense image patches to produce per-pixel class probabilities. The CRF infers a labelling that smooths regions while respecting the edges present in the imagery. The method is applied to the ISPRS 2D semantic labelling challenge dataset with competitive classification accuracy.
更多查看译文
关键词
accuracy,labeling,semantics,feature extraction,visualization
AI 理解论文
溯源树
样例
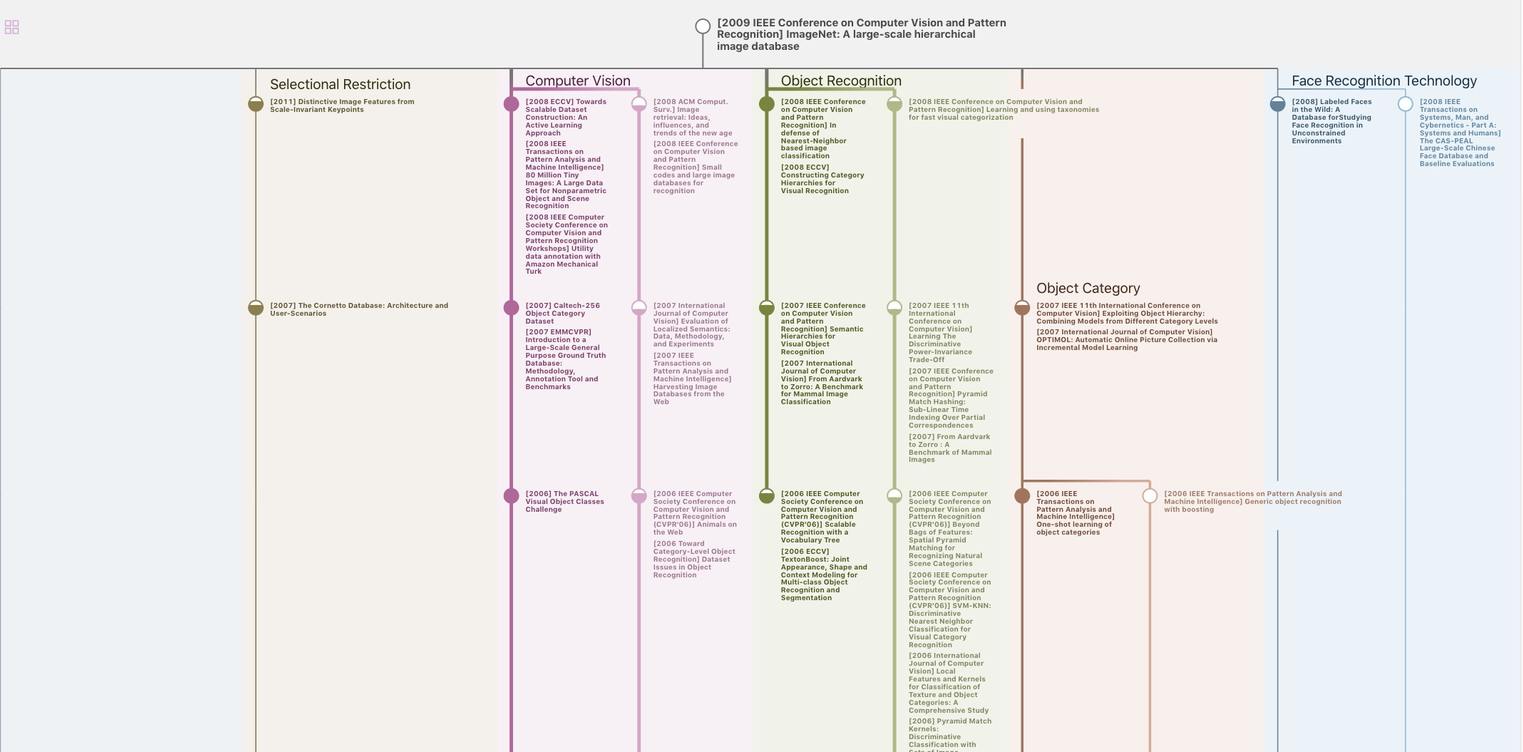
生成溯源树,研究论文发展脉络
Chat Paper
正在生成论文摘要