Ambient Occlusion Via Compressive Visibility Estimation
2015 IEEE Conference on Computer Vision and Pattern Recognition (CVPR)(2015)
摘要
There has been emerging interest on recovering traditionally challenging intrinsic scene properties. In this paper, we present a novel computational imaging solution for recovering the ambient occlusion (AO) map of an object. AO measures how much light from all different directions can reach a surface point without being blocked by self-occlusions. Previous approaches either require obtaining highly accurate surface geometry or acquiring a large number of images. We adopt a compressive sensing framework that captures the object under strategically coded lighting directions. We show that this incident illumination field exhibits some unique properties suitable for AO recovery: every ray's contribution to the visibility function is binary while their distribution for AO measurement is sparse. This enables a sparsity-prior based solution for iteratively recovering the surface normal, the surface albedo, and the visibility function from a small number of images. To physically implement the scheme, we construct an encodable directional light source using the light field probe. Experiments on synthetic and real scenes show that our approach is both reliable and accurate with significantly reduced size of input.
更多查看译文
关键词
ambient occlusion,compressive visibility estimation,intrinsic scene property recovery,computational imaging solution,compressive sensing framework,strategically coded lighting directions,sparsity-prior based solution,encodable directional light source,light field probe
AI 理解论文
溯源树
样例
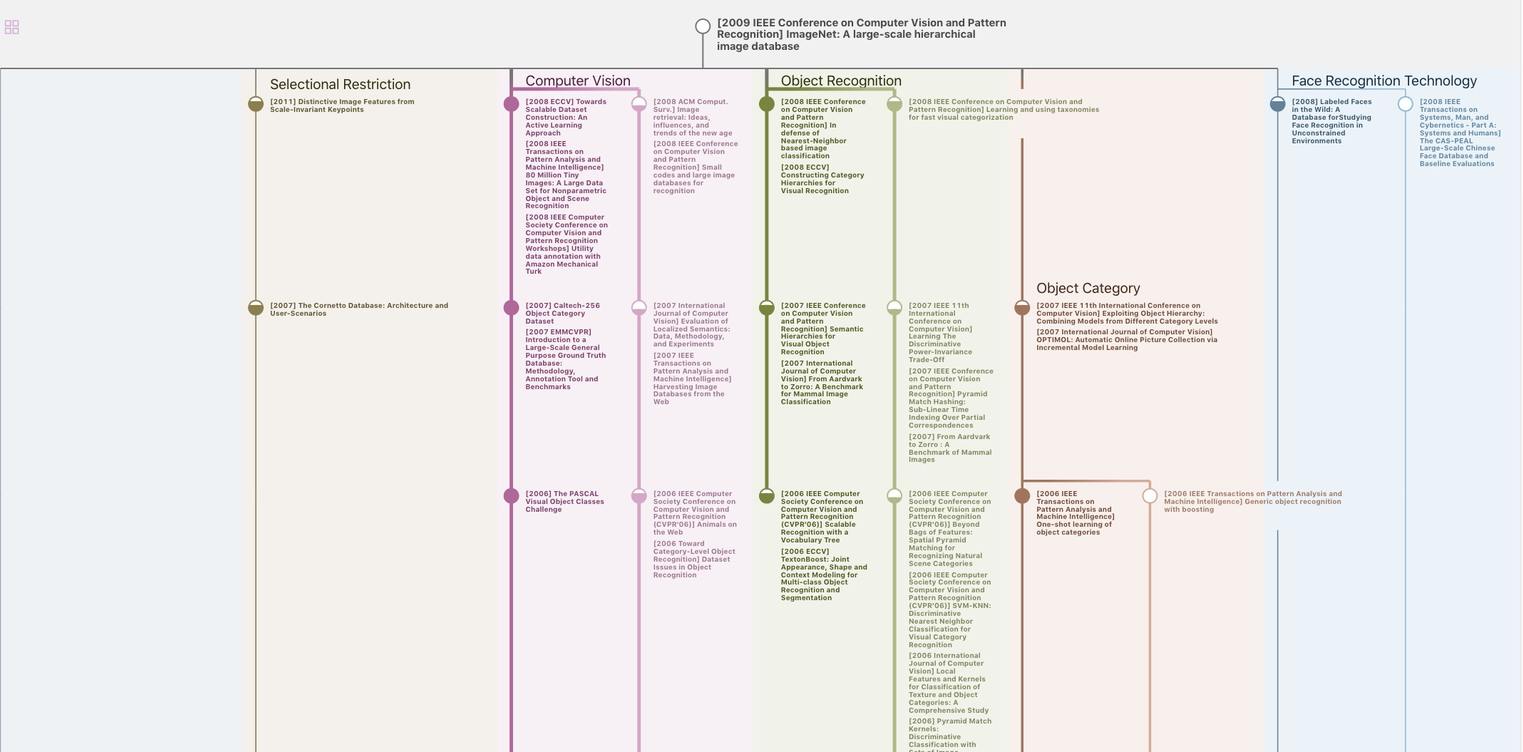
生成溯源树,研究论文发展脉络
Chat Paper
正在生成论文摘要