Deep Sparse Representation For Robust Image Registration
2015 IEEE Conference on Computer Vision and Pattern Recognition (CVPR)(2015)
摘要
The definition of the similarity measure is an essential component in image registration. In this paper, we propose a novel similarity measure for registration of two or more images. The proposed method is motivated by that the optimally registered images can be deeply sparsified in the gradient domain and frequency domain, with the separation of a sparse tensor of errors. One of the key advantages of the proposed similarity measure is its robustness to severe intensity distortions, which widely exist on medical images, remotely sensed images and natural photos due to the difference of acquisition modalities or illumination conditions. Two efficient algorithms are proposed to solve the batch image registration and pair registration problems in a unified framework. We validate our method on extensive challenging datasets. The experimental results demonstrate the robustness, accuracy and efficiency of our method over 9 traditional and state-of-the-art algorithms on synthetic images and a wide range of real-world applications.
更多查看译文
关键词
deep sparse representation,robust image registration,similarity measure,gradient domain,frequency domain,severe intensity distortion,medical image,remotely sensed image,natural photo,acquisition modality,illumination condition,batch image registration,pair registration problem,computer vision
AI 理解论文
溯源树
样例
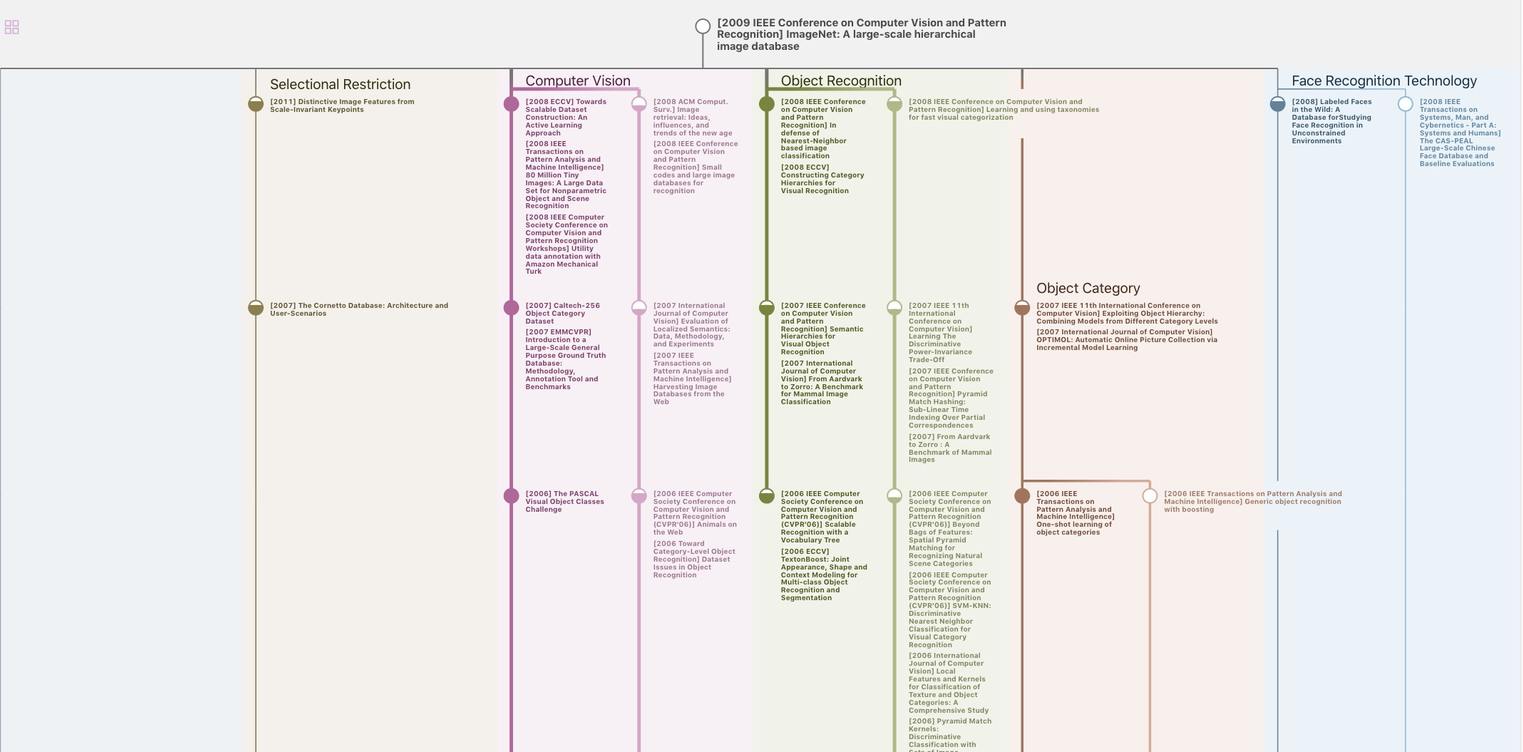
生成溯源树,研究论文发展脉络
Chat Paper
正在生成论文摘要