Mining Semantic Affordances Of Visual Object Categories
2015 IEEE Conference on Computer Vision and Pattern Recognition (CVPR)(2015)
摘要
Affordances are fundamental attributes of objects. Affordances reveal the functionalities of objects and the possible actions that can be performed on them. Understanding affordances is crucial for recognizing human activities in visual data and for robots to interact with the world. In this paper we introduce the new problem of mining the knowledge of semantic affordance: given an object, determining whether an action can be performed on it. This is equivalent to connecting verb nodes and noun nodes in Word Net, or filling an affordance matrix encoding the plausibility of each action-object pair. We introduce a new benchmark with crowdsourced ground truth affordances on 20 PASCAL VOC object classes and 957 action classes. We explore a number of approaches including text mining, visual mining, and collaborative filtering. Our analyses yield a number of significant insights that reveal the most effective ways of collecting knowledge of semantic affordances.
更多查看译文
关键词
semantic affordance mining,visual object categories,human activity recognition,robots,knowledge mining,verb nodes,noun nodes,WordNet,affordance matrix,crowdsourced ground truth affordances,PASCAL VOC object classes,action classes,text mining,visual mining,collaborative filtering
AI 理解论文
溯源树
样例
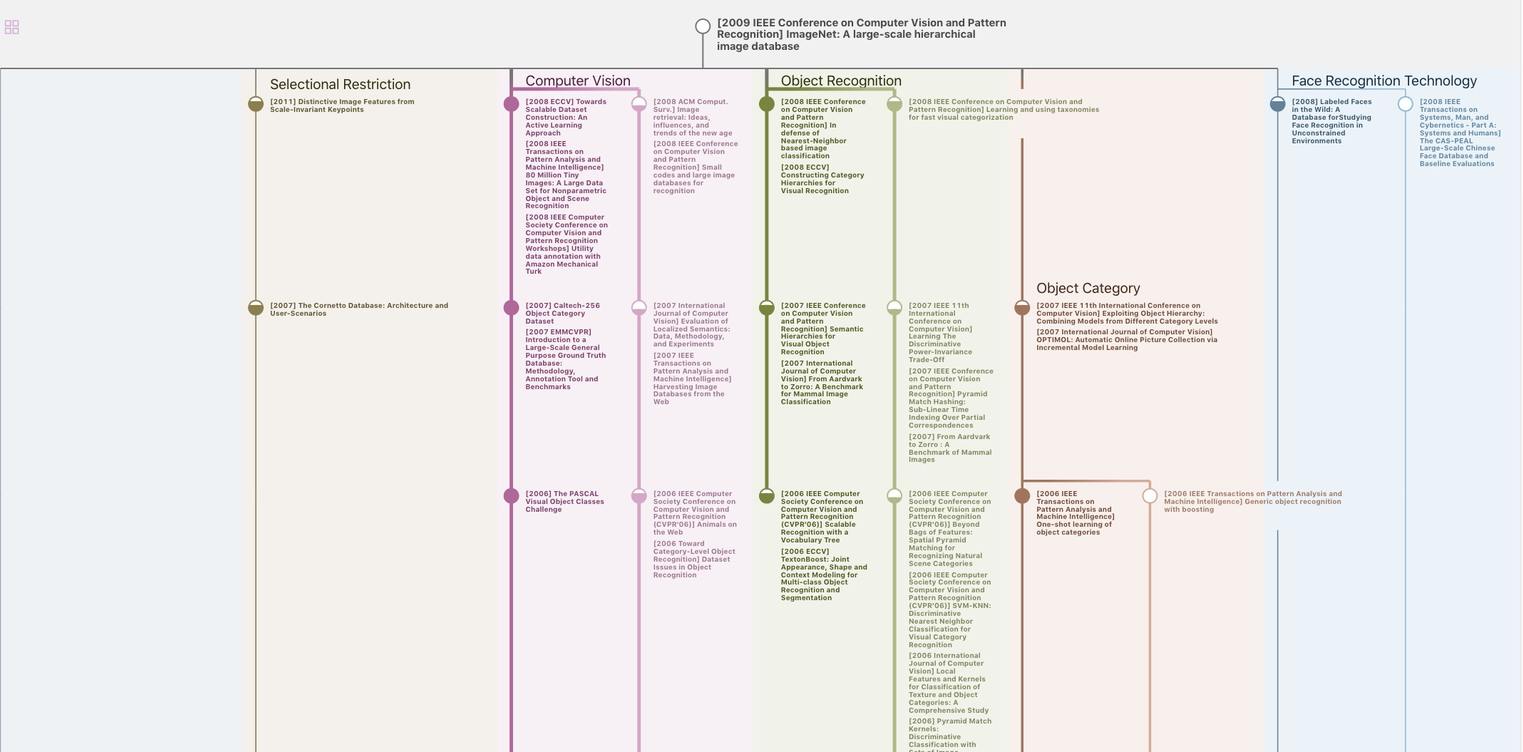
生成溯源树,研究论文发展脉络
Chat Paper
正在生成论文摘要