Factorization threshold models for scale-free networks generation.
Signal-Image Technology and Internet-Based Systems(2016)
摘要
Many real networks such as the World Wide Web, financial, biological, citation and social networkshave a power-law degree distribution. Networks with this feature are also called scale-free. Several models for producing scale-free networks have been obtained by now and most of them are based on the preferential attachment approach. We will offer the model with another scale-free property explanation. The main idea is to approximate the network's adjacency matrix by multiplication of the matrices V and VT, where V is the matrix of vertices' latent features. This approach is called matrix factorization and is successfully used in the link prediction problem. To create a generative model of scale-free networks we will sample latent features V from some probabilistic distribution and try to generate a network's adjacency matrix. Entries in the generated matrix are dot products of latent features which are real numbers. In order to create an adjacency matrix, we approximate entries with the Boolean domain {0, 1}. We have incorporated the threshold parameter θ into the model for discretization of a dot product. Actually, we have been influenced by the geographical threshold models which were recently proven to have good results in a scale-free networks generation. The overview of our results is the following. First, we will describe our model formally. Second, we will tune the threshold θ in order to generate sparse growing networks. Finally, we will show that our model produces scale-free networks with the fixed power-law exponent which equals two. In order to generate oriented networks with tunable power-law exponents and to obtain other model properties, we will offer different modifications of our model. Some of our results will be demonstrated using computer simulation.
更多查看译文
关键词
Matrix factorization,Scale-free networks,Threshold models
AI 理解论文
溯源树
样例
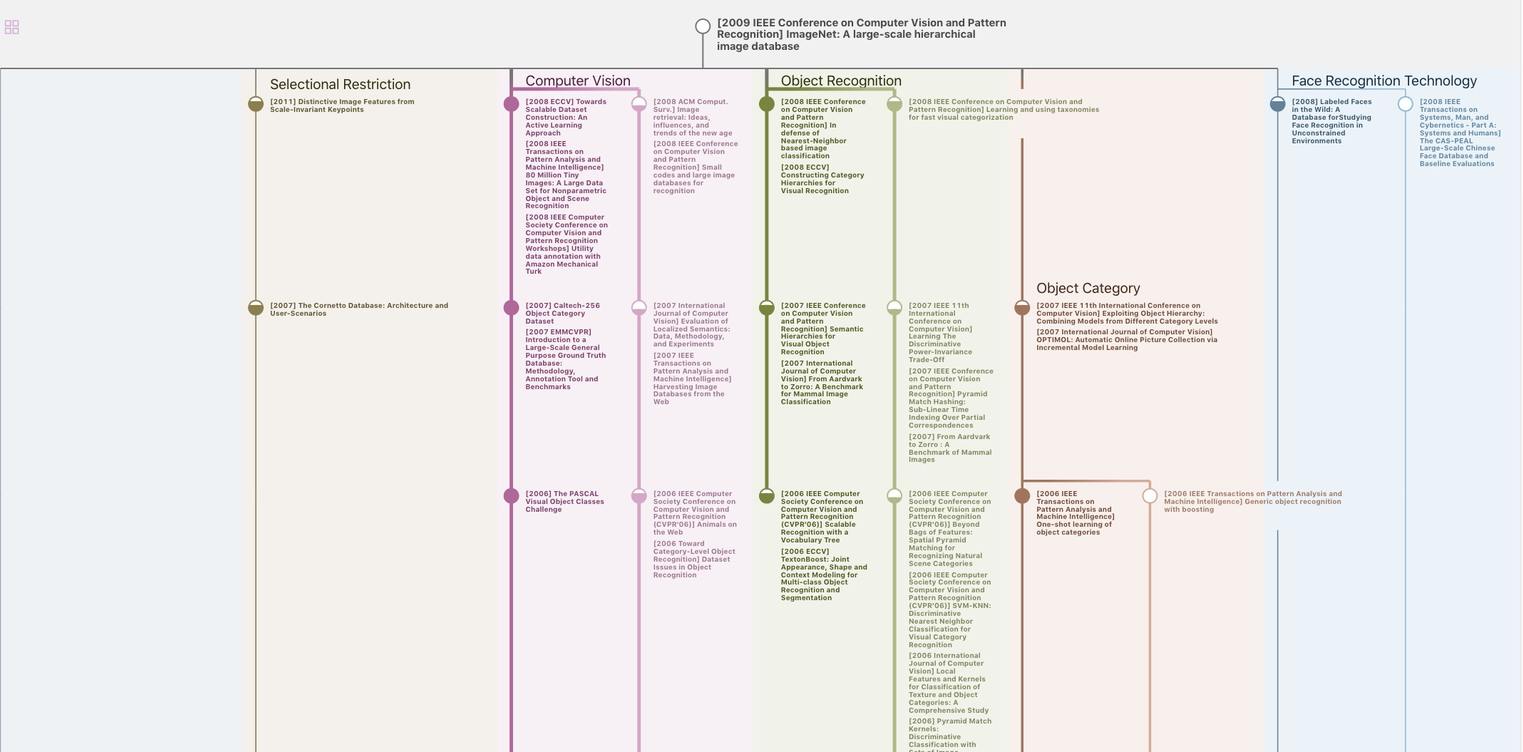
生成溯源树,研究论文发展脉络
Chat Paper
正在生成论文摘要