A Piggyback System For Joint Entity Mention Detection And Linking In Web Queries
WWW '16: 25th International World Wide Web Conference Montréal Québec Canada April, 2016(2016)
摘要
In this paper we study the problem of linking open-domain web-search queries towards entities drawn from the full entity inventory of Wikipedia articles. We introduce SMAPH2, a second-order approach that, by piggybacking on a web search engine, alleviates the noise and irregularities that characterize the language of queries and puts queries in a larger context in which it is easier to make sense of them. The key algorithmic idea underlying SMAPH-2 is to first discover a candidate set of entities and then link-back those entities to their mentions occurring in the input query. This allows us to confine the possible concepts pertinent to the query to only the ones really mentioned in it. The link-back is implemented via a collective disambiguation step based upon a supervised ranking model that makes one joint prediction for the annotation of the complete query optimizing directly the F1 measure. We evaluate both known features, such as word embeddings and semantic relatedness among entities, and several novel features such as an approximate distance between mentions and entities (which can handle spelling errors). We demonstrate that SMAPH-2 achieves state-of-the-art performance on the ERD@SIGIR2014 benchmark. We also publish GERDAQ (General Entity Recognition, Disambiguation and Annotation in Queries), a novel, public dataset built specifically for web-query entity linking via a crowdsourcing effort. SMAPH-2 outperforms the benchmarks by comparable margins also on GERDAQ.
更多查看译文
关键词
Entity linking,query annotation,ERD,piggyback
AI 理解论文
溯源树
样例
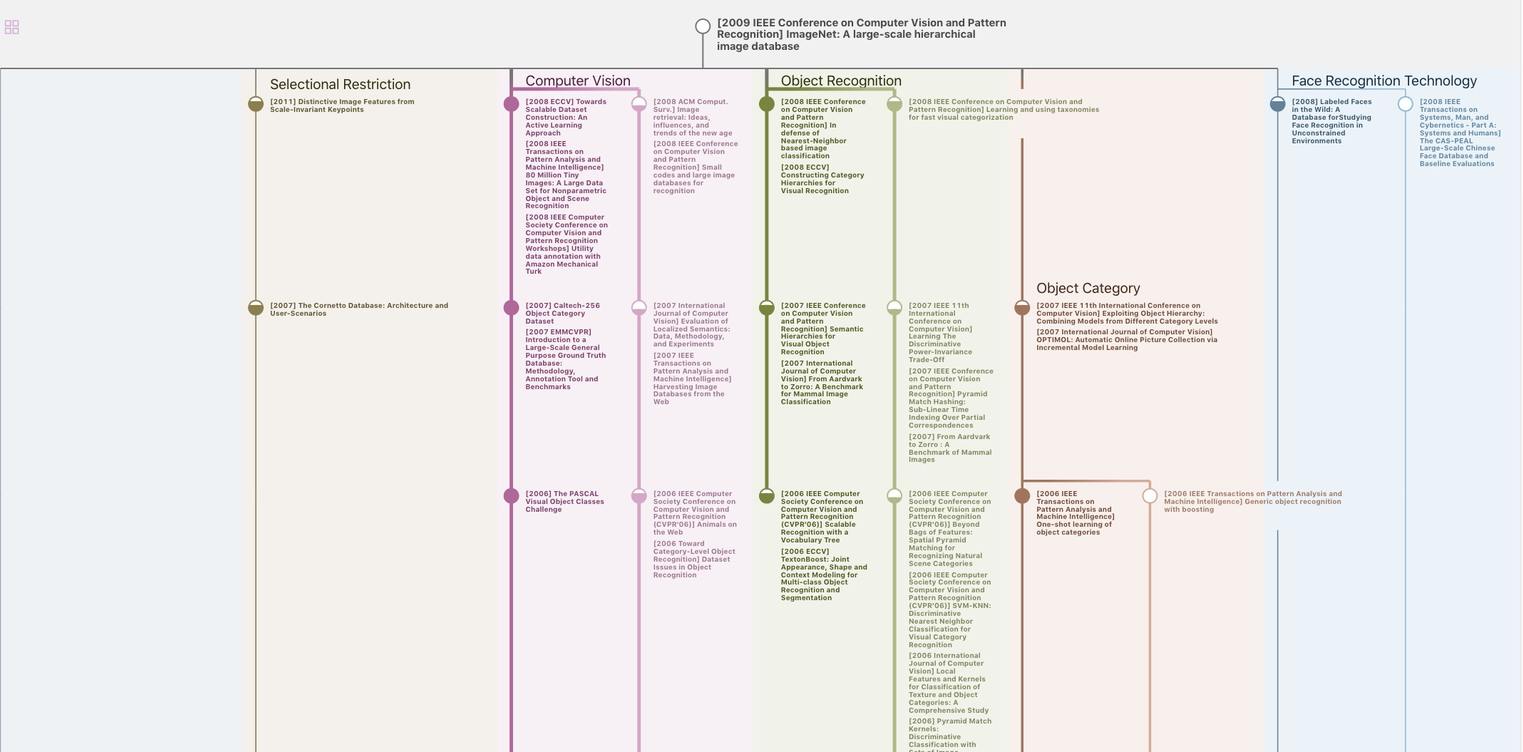
生成溯源树,研究论文发展脉络
Chat Paper
正在生成论文摘要