Separation Matrix Optimization Using Associative Memory Model For Blind Source Separation
2015 23rd European Signal Processing Conference (EUSIPCO)(2015)
摘要
A source signalis estimated using an associative memory model (AMM) and used for separation matrix optimization in linear blind source separation (BSS) to yield high quality and less distorted speech. Linear-filtering-based BSS, such as independent vector analysis (IVA), has been shown to he effective in sound source separation while avoiding non-linear signal distortion. This technique, however, requires several assumptions of sound sources being independent and generated from non-Gaussian distribution. We propose a method for estimating a linear separation matrix without, any assumptions about the sources by repeating the following two steps: estimating non-distorted reference signals by using an AMM and optimizing the separation matrix to minimize an error between the estimated signal and reference signal. Experimental comparisons carried out in simultaneous speech separation suggest that the proposed method can reduce the residual distortion caused by IVA.
更多查看译文
关键词
convolutional neural network,denoising autoencoder,associative memory model,linear filtering,blind source separation
AI 理解论文
溯源树
样例
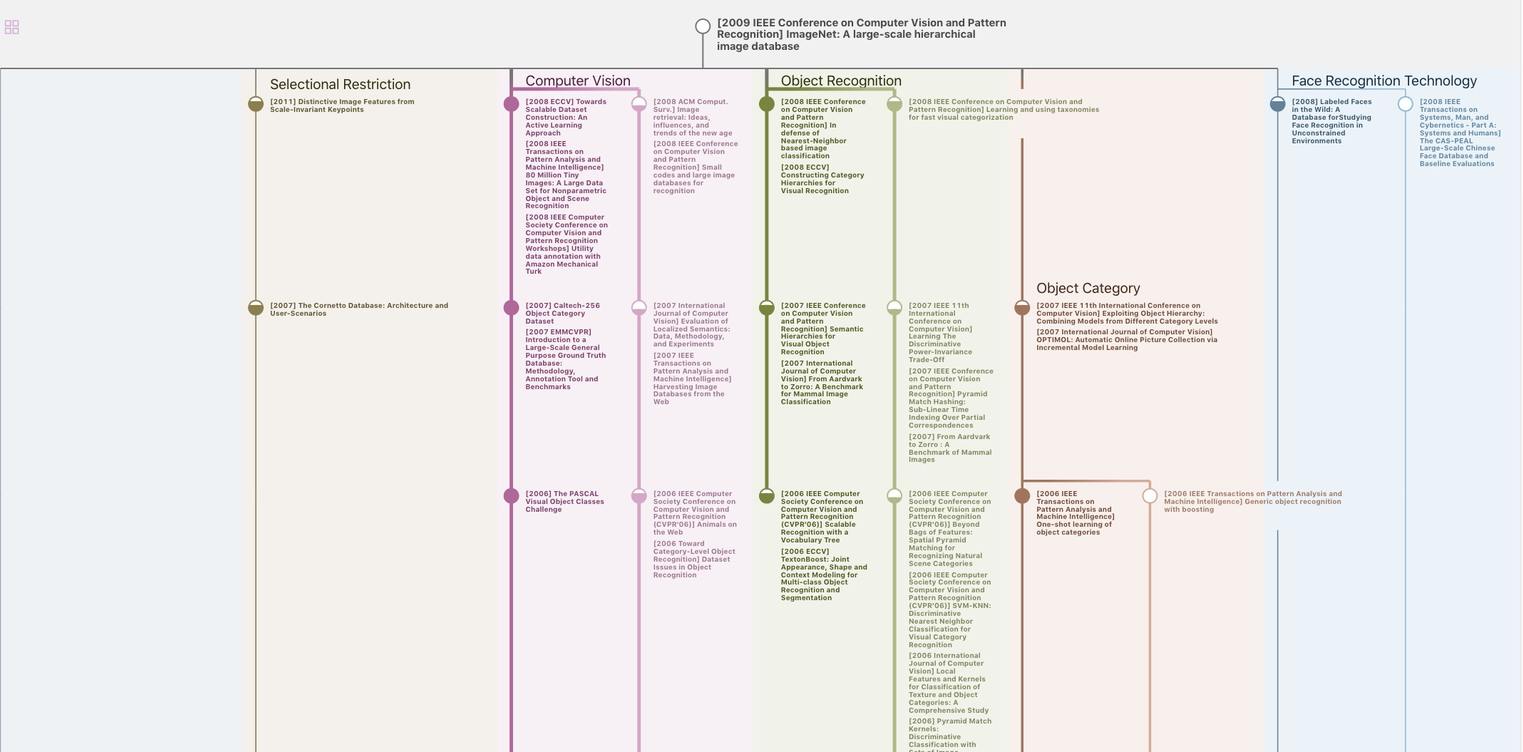
生成溯源树,研究论文发展脉络
Chat Paper
正在生成论文摘要