Efficient Aspect Object Models Using Pre-Trained Convolutional Neural Networks
2015 IEEE-RAS 15th International Conference on Humanoid Robots (Humanoids)(2015)
摘要
We study the problem of object recognition on robotic platforms where large image collections of target objects are unavailable and where new models of previously unseen objects must be added dynamically. This situation is common in robotics, where task related objects can require recognition over multiple viewpoints and training examples are sparse. The proposed framework uses pre-trained convolutional neural network layers to support aspect object models while emphasizing a minimal computational footprint. In this paper, we maintain an object model database consisting of aspect and class descriptors computed from images of target objects at varying view points. By querying the model database we show how to recognize objects with respect to previously seen exemplars. We investigate the effectiveness of different dimensionality reduction techniques for key generation on query efficiency and accuracy. We also demonstrate a working system with a small collection of objects including classes that do not appear in the network's pre-training data set.
更多查看译文
关键词
object recognition,robotic platforms,image collections,pretrained convolutional neural network layers,aspect object models,minimal computational footprint,object model database,class descriptors,dimensionality reduction,query efficiency,query accuracy
AI 理解论文
溯源树
样例
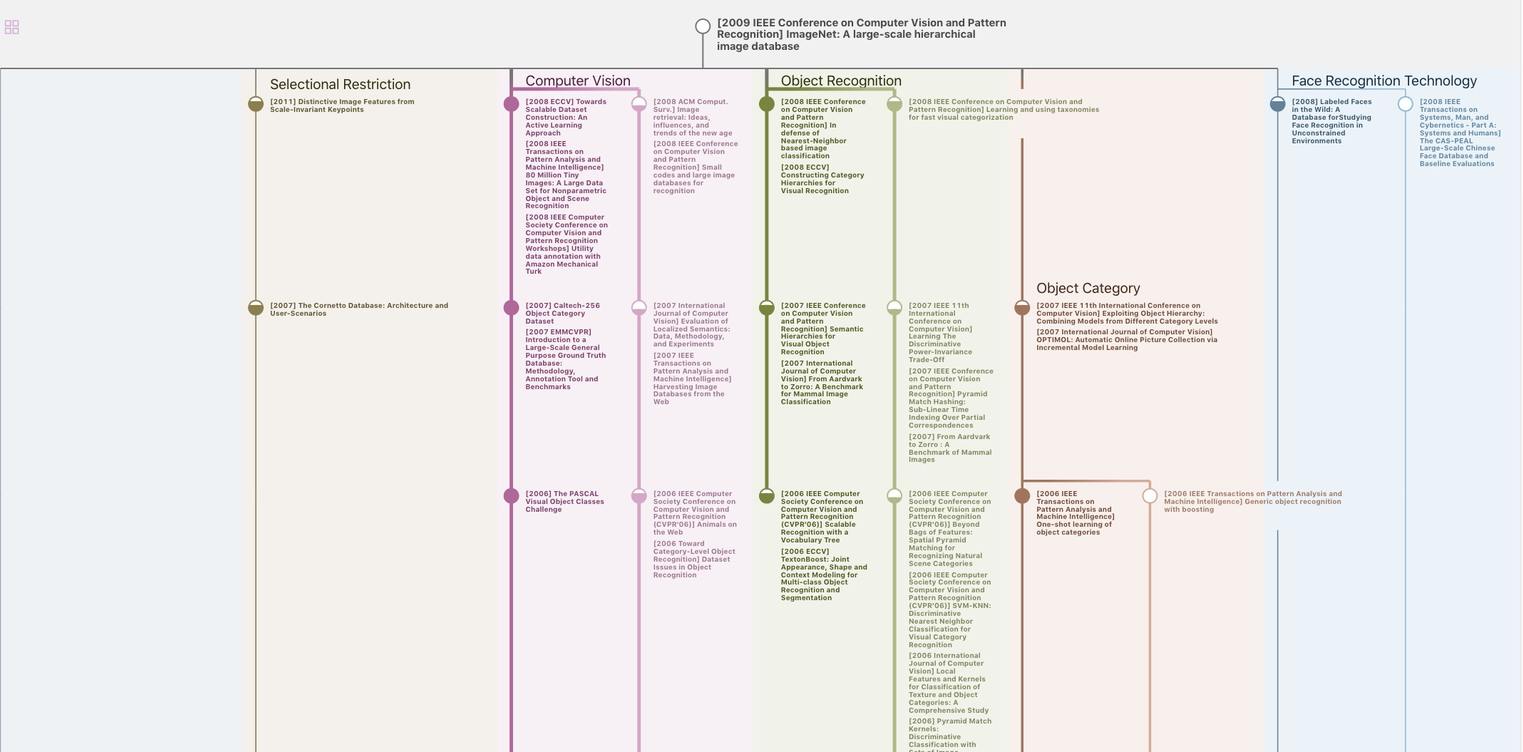
生成溯源树,研究论文发展脉络
Chat Paper
正在生成论文摘要