Predicting Continuous Probability Distribution Of Image Emotions In Valence-Arousal Space
MM '15: ACM Multimedia Conference Brisbane Australia October, 2015(2015)
摘要
Previous works on image emotion analysis mainly focused on assigning a dominated emotion category or the average dimension values to an image for affective image classification and regression. However, this is often insufficient in many applications, as the emotions that are evoked in viewers by an image are highly subjective and different. In this paper, we propose to predict the continuous probability distribution of dimensional image emotions represented in valence-arousal space. By the statistical analysis on the constructed Image-Emotion-Social-Net dataset, we represent the emotion distribution as a Gaussian mixture model (GMM), which is estimated by the EM algorithm. Then we extract commonly used features of different levels for each image. Finally, we formulize the emotion distribution prediction as a multi-task shared sparse regression (MTSSR) problem, which is optimized by iteratively reweighted least squares. Besides, we introduce three baseline algorithms. Experiments conducted on the Image-Emotion-Social Net dataset demonstrate the superiority of the proposed method, as compared to some state-of-the-art approaches.
更多查看译文
关键词
Image Emotion Distribution,Valence-Arousal,Gaussian Mixture Model,Sparse Regression
AI 理解论文
溯源树
样例
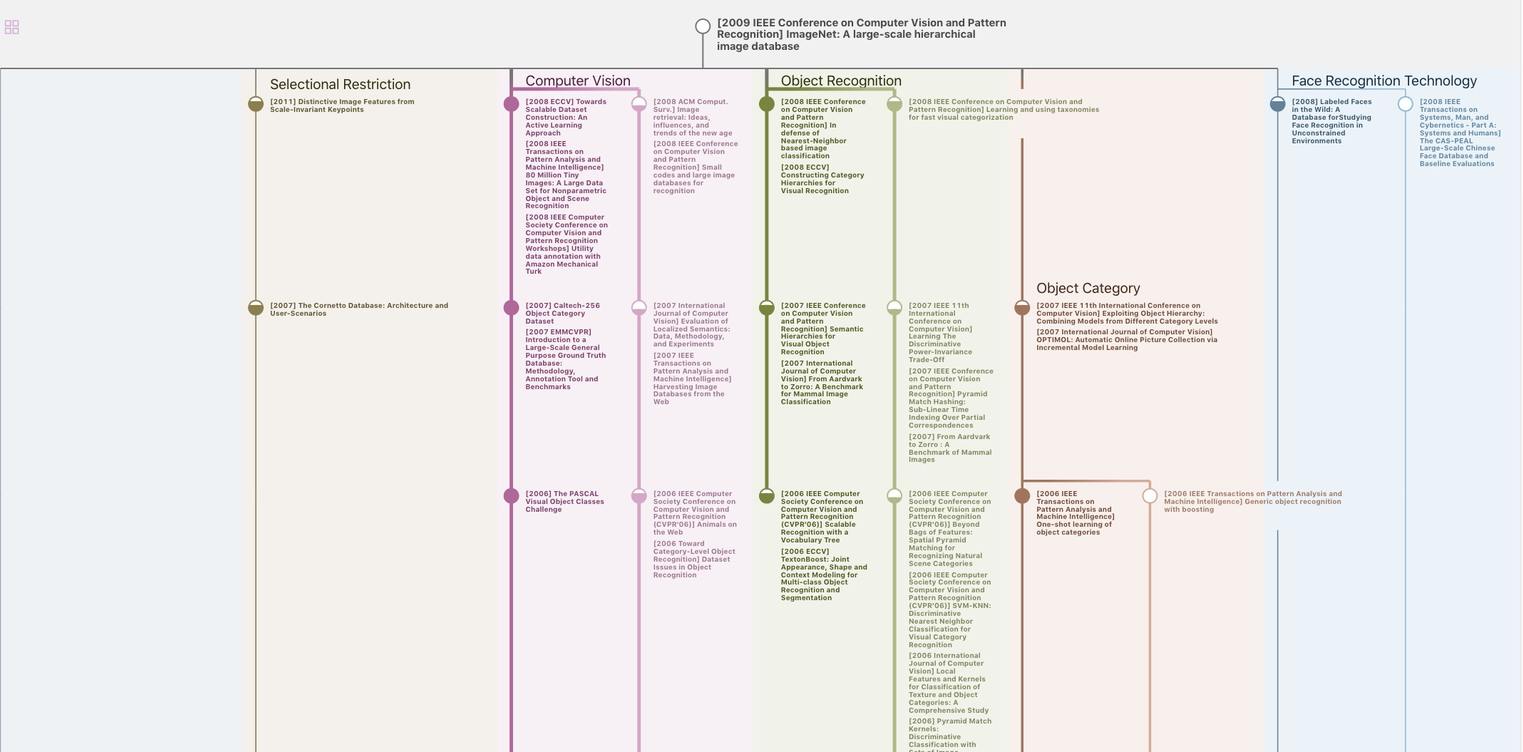
生成溯源树,研究论文发展脉络
Chat Paper
正在生成论文摘要