Towards automatic prediction of student performance in STEM undergraduate degree programs
SAC 2015: Symposium on Applied Computing Salamanca Spain April, 2015(2015)
摘要
STEM is defined as learning in the fields of Science, Technology, Engineering and Mathematics. In Brazil, many students leave the educational system before achieving a tertiary degree in these fields. Poor academic performance in STEM undergraduate courses is an issue faced by many universities, both in developed and emerging countries. Although these universities store large amounts of data, there are few studies about educational data mining (EDM) software tools designed to aid educational managers in analyzing student learning and improving the quality of undergraduate degree programs. Our approach may assist managers in supervising students at the end of each academic term, thus enabling them to identify the students in difficulty of fulfilling the academic requirements toward a degree. This paper shows quantitative experimental studies using a large dataset of real data from five traditional STEM undergraduate courses of one of the largest public Brazilian universities. Finally, the results show that data mining algorithms can establish effective prediction models from existing student data.
更多查看译文
关键词
educational data mining (EDM), STEM, prediction model, algorithm
AI 理解论文
溯源树
样例
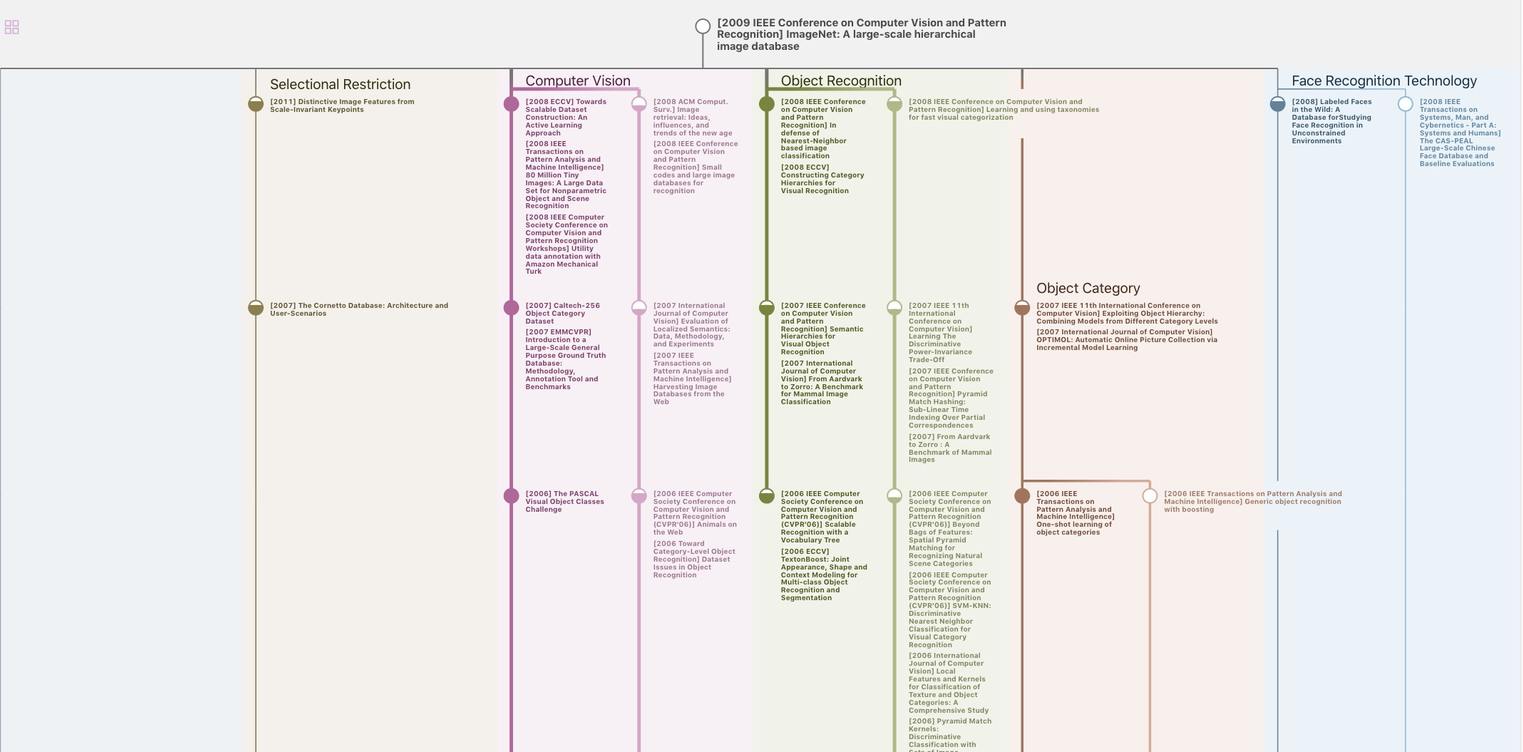
生成溯源树,研究论文发展脉络
Chat Paper
正在生成论文摘要