Multi-Modal Retrieval Via Deep Textual-Visual Correlation Learning
INTELLIGENCE SCIENCE AND BIG DATA ENGINEERING: IMAGE AND VIDEO DATA ENGINEERING, ISCIDE 2015, PT I(2015)
摘要
In this paper, we consider multi-modal retrieval from the perspective of deep textual-visual learning so as to preserve the correlations between multi-modal data. More specifically, We propose a general multi-modal retrieval algorithm to maximize the canonical correlations between multi-modal data via deep learning, which we call Deep Textual-Visual correlation learning (DTV). In DTV, given pairs of images and their describing documents, a convolutional neural network is implemented to learn the visual representation of images and a dependency-tree recursive neural network(DT-RNN) is conducted to learn compositional textual representations of documents respectively, then DTV projects the visual-textual representation into a common embedding space where each pair of multi-modal data is maximally correlated subject to being unrelated with other pairs by matrix-vector canonical correlation analysis (CCA). The experimental results indicate the effectiveness of our proposed DTV when applied to multi-modal retrieval.
更多查看译文
关键词
Multi-modal retrieval, Deep learning, CCA
AI 理解论文
溯源树
样例
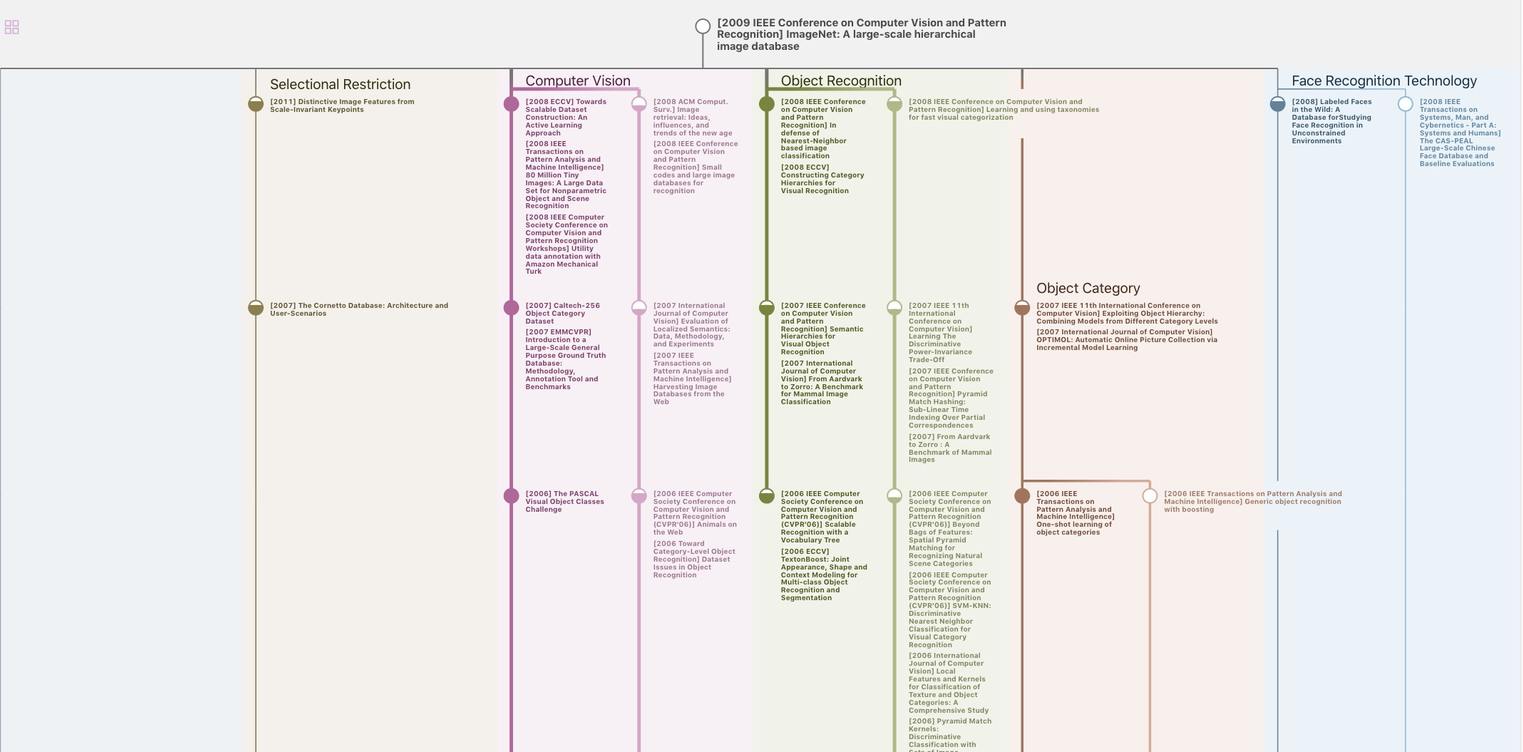
生成溯源树,研究论文发展脉络
Chat Paper
正在生成论文摘要