Time Series Forecasting with PSO-Optimized Neural Networks.
MICAI '14: Proceedings of the 2014 13th Mexican International Conference on Artificial Intelligence(2014)
摘要
In this paper, we propose a new methodology to forecast values for univariate time series datasets, based on a Feed Forward Neural Network (FFNN) ensemble. Each ensemble element is trained with the Particle Swarm Optimization (PSO) algorithm, this ensemble produces a final sequence of time series forecasts via a bootstrapping procedure. Our proposed methodology is compared against Auto-Regressive Integrated Moving Average (ARIMA) models. This experiment gives us a good idea of how effective soft computing techniques can be in the field of time series modeling. The results obtained show empirically that our proposed methodology is robust and produces useful forecast error bounds that provide a clear picture of a time series' future movements.
更多查看译文
关键词
Soft Computing,Artificial Intelligence,Time Series Forecasts,ARIMA models,Artificial Neural Networks,Committee Machines,Bootstrapping,Particle Swarm Optimization,Evolutionary Algorithms
AI 理解论文
溯源树
样例
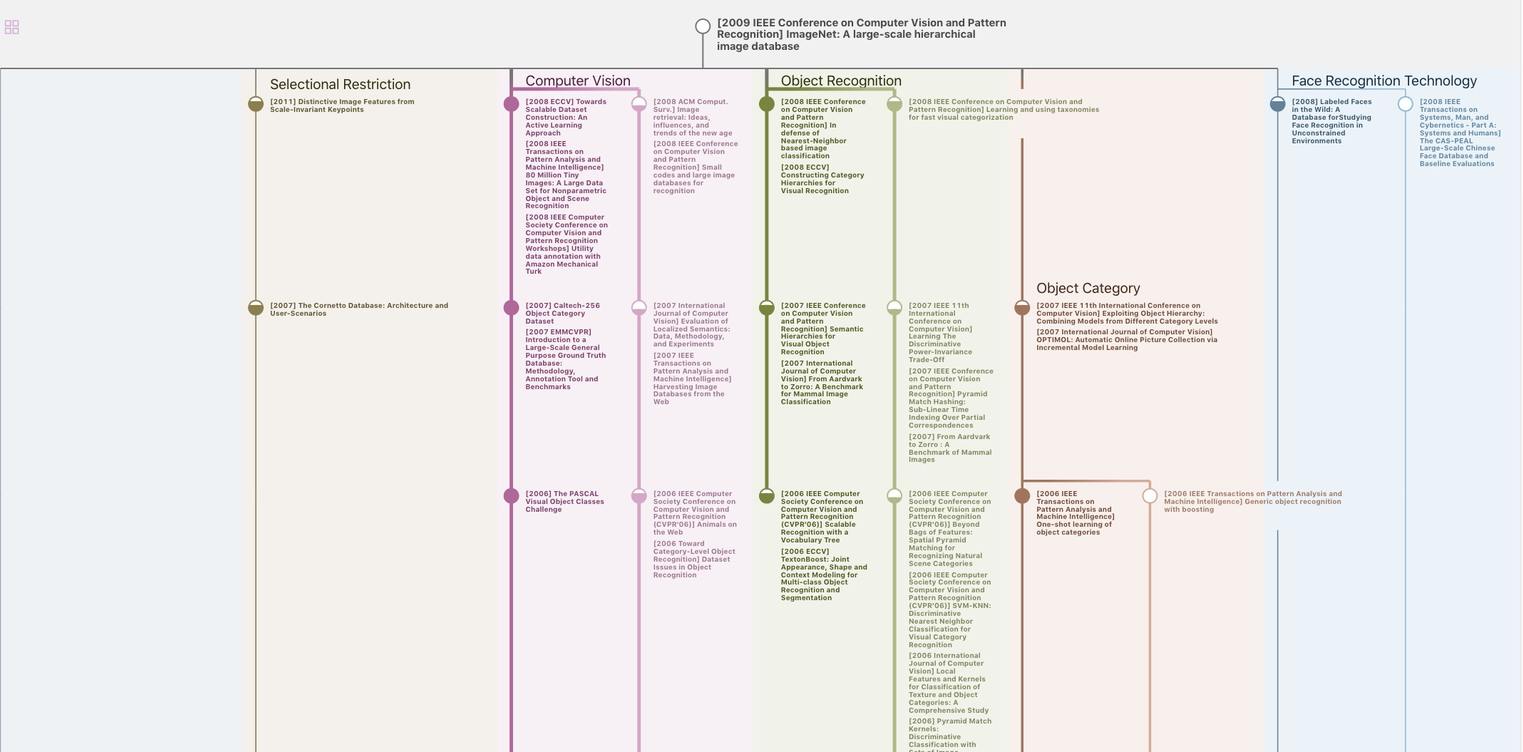
生成溯源树,研究论文发展脉络
Chat Paper
正在生成论文摘要