Source-Target-Source Classification Using Stacked Denoising Autoencoders
PATTERN RECOGNITION AND IMAGE ANALYSIS (IBPRIA 2015)(2015)
摘要
Deep Transfer Learning (DTL) emerged as a new paradigm in machine learning in which a deep model is trained on a source task and the knowledge acquired is then totally or partially transferred to help in solving a target task. Even though DTL offers a greater flexibility in extracting high-level features and enabling feature transference from a source to a target task, the DTL solution might get stuck at local minima leading to performance degradation-negative transference-, similar to what happens in the classical machine learning approach. In this paper, we propose the Source-Target-Source (STS) methodology to reduce the impact of negative transference, by iteratively switching between source and target tasks in the training process. The results show the effectiveness of such approach.
更多查看译文
关键词
Deep neural network, Transfer learning, Optimization
AI 理解论文
溯源树
样例
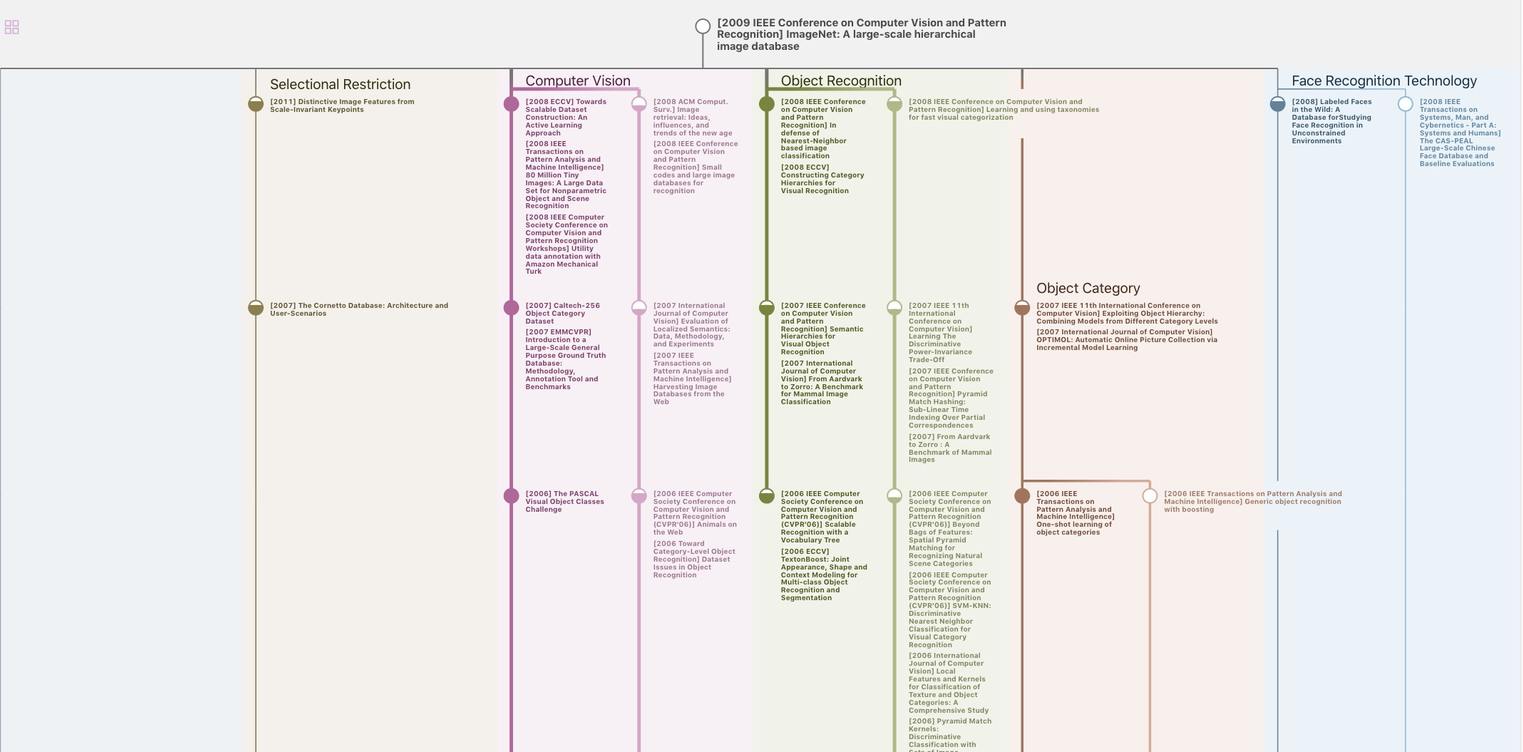
生成溯源树,研究论文发展脉络
Chat Paper
正在生成论文摘要