Classifier self-assessment: active learning and active noise correction for document classification
International Conference on Document Analysis and Recognition(2015)
摘要
This paper introduces two novel techniques that improve document classification while reducing the amount of manual work by the user. The first technique applies uncertainty sampling as a metric for batch-mode active learning to suggest only the most interesting documents for the manual labeling process, resulting in a steep improvement even for small training sets. This addresses the problem of creating and improving an initial training set. The second technique focuses on cleaning an existing large set of weakly labeled documents by active noise correction. The classifier's self-assessment is used to detect mislabeled documents which are then reclassified. For active noise correction, two approaches are explored: one based on a human expert and one that automatically corrects the assigned labels.
更多查看译文
关键词
classifier self-assessment,active noise correction,document classification,uncertainty sampling,batch-mode active learning,manual labeling process,steep improvement,training sets,labeled documents,human expert
AI 理解论文
溯源树
样例
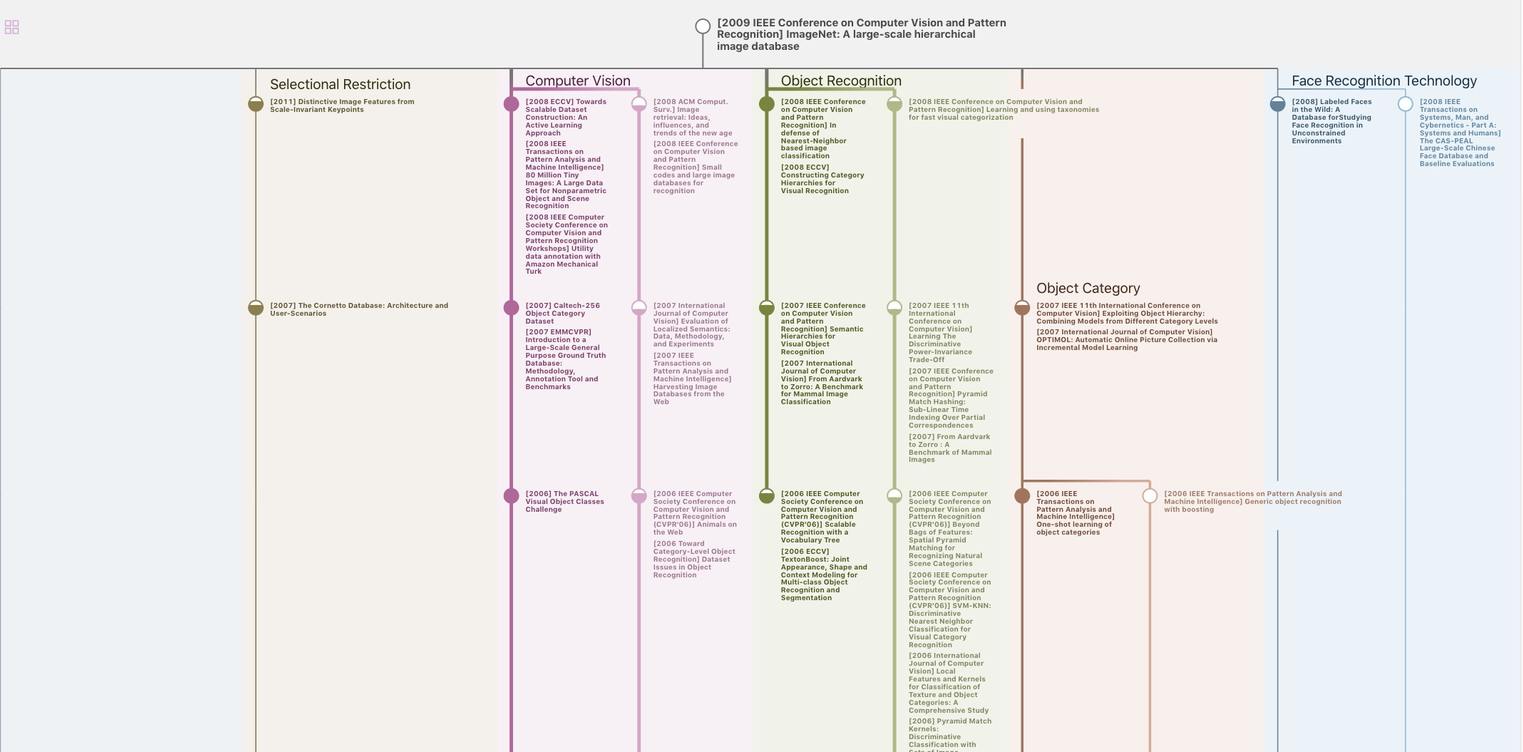
生成溯源树,研究论文发展脉络
Chat Paper
正在生成论文摘要