A learning based mobile user traffic characterization for efficient resource management in cellular networks
CCNC(2015)
摘要
With the evolution of various new types of application services for mobile devices, cellular operators have started providing multiple subscription plans to the mobile users. The plan subscribed determines the Quality of Service (QoS) to be provided to the user, and operators distinguish users as priority users and non-priority users, accordingly. To ensure better QoS to the priority users, necessary resources must be reserved at the base station. This demands analyzing the network traffic to predict future traffic pattern. This paper pioneers the use of machine learning methods to forecast mobile user traffic pattern for providing better QoS to the priority users. We analyze two different supervised learning methods, Naive Bayes Classifier and Logistic Regression, used for prediction of probable times when a priority user would be active. The prediction results are applied to the user scheduling strategies for efficient bandwidth management, thus improving system capacity and reduce blocking. Simulations on multiple real-life datasets validate the model and predict the mobile user pattern with very high accuracy, along with significant reduction in priority user service blocking ratio and improvement in their capacity.
更多查看译文
关键词
Admission Control, Resource Management, User Modeling, Priority Scheduling, Traffic Forecasting
AI 理解论文
溯源树
样例
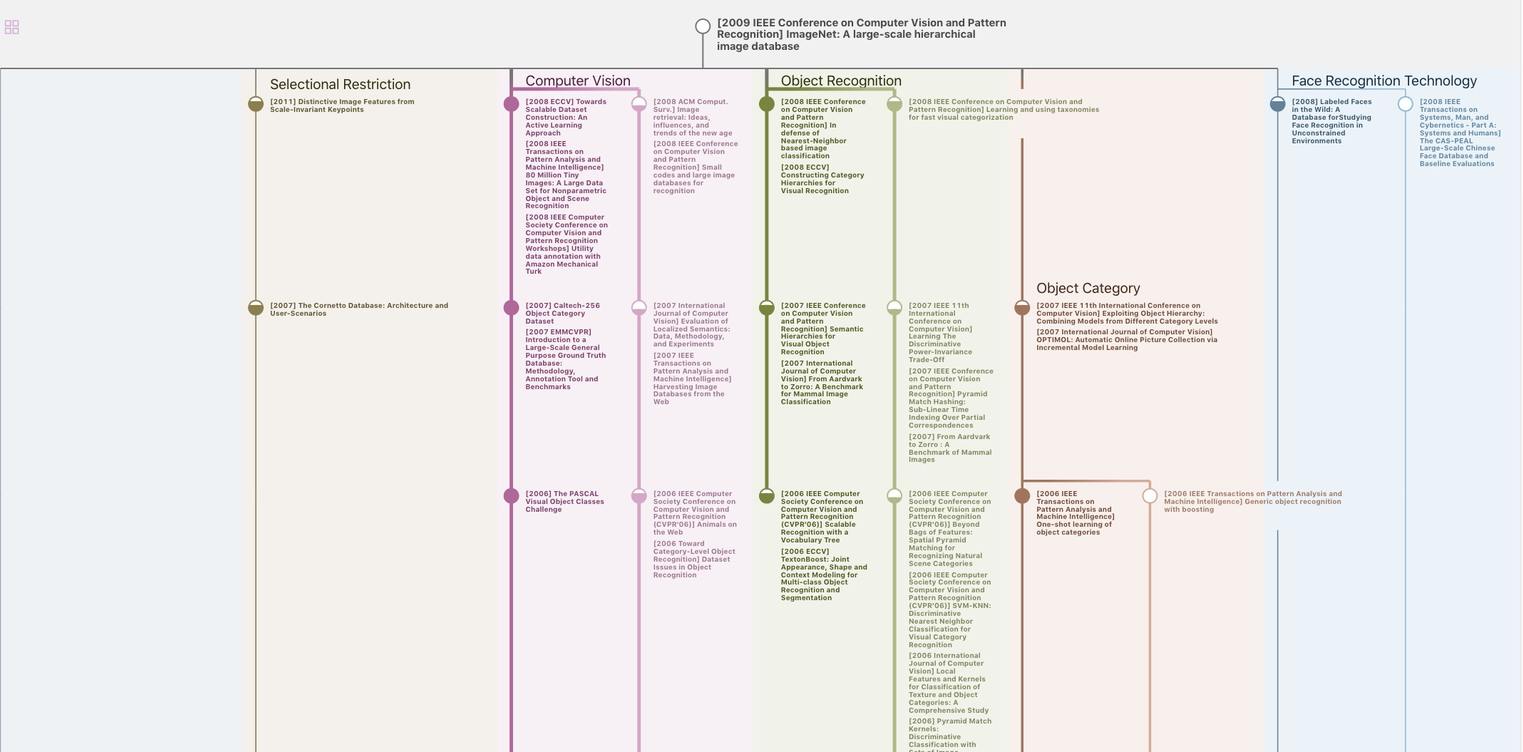
生成溯源树,研究论文发展脉络
Chat Paper
正在生成论文摘要