Low memory-cost scramble methods for constructing deterministic CS matrix
2015 IEEE Workshop on Signal Processing Systems (SiPS)(2015)
摘要
Compressed sensing (CS) is an emerging field in data acquisition that measures signal below the Nyquist rate through sensing matrix. Random matrices have been regarded as optimal sensing matrices in CS but cost too much memory space. Structured matrices are then proposed to decrease memory cost by deterministic sensing. Nevertheless, the recovery suffers from severe degradation when using these structured matrices as sensing matrices for signals which are sparse under specific domain. In this paper, we propose two scramble methods to compensate the performance loss of structured matrices under alternative region of interest. The proposed predefined scramble matrix (PSM) method is able to match the guarantee region of recovery of Gaussian random matrices. The PSM method requires as much memory space as the structured matrices and has sub-optimal recovery performance. On the other hand, the proposed built-in location selection (BLS) method is able to approximate the whole region of recovery of Gaussian random matrices. The BLS method can achieve optimal recovery performance with some extra memory and logic cost than PSM method. Compared to 128 × 512 Gaussian sensing matrix, the PSM method and the BLS method can reduce 98.4% and 96% of the memory cost, respectively.
更多查看译文
关键词
compressed sensing(CS),structured matrix,deterministic sensing matrix
AI 理解论文
溯源树
样例
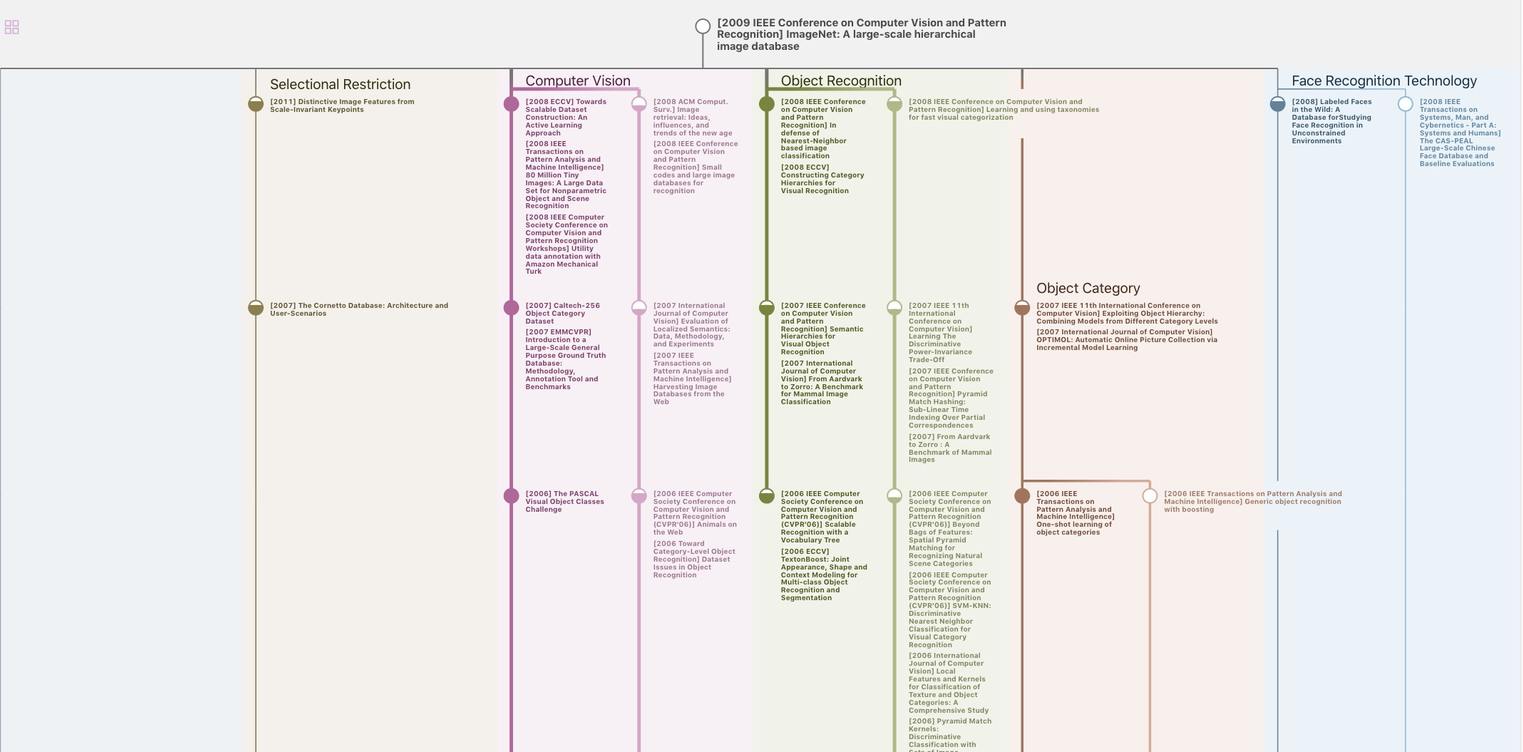
生成溯源树,研究论文发展脉络
Chat Paper
正在生成论文摘要