Click-Through Prediction For Advertising In Twitter Timeline
KDD(2015)
摘要
We present the problem of click-through prediction for advertising in Twitter timeline, which displays a stream of Tweets from accounts a user choose to follow. Traditional computational advertising usually appears in two forms: sponsored search that places ads onto the search result page when a query is issued to a search engine, and contextual advertising that places ads onto a regular, usually static Web page. Compared with these two paradigms, placing ads into a Tweet stream is particularly challenging given the nature of the data stream: the context into which an ad can be placed updates dynamically and never replicates. Every ad is therefore placed into a unique context. This makes the information available for training a machine learning model extremely sparse. In this study, we propose a learning-to-rank method which not only addresses the sparsity of training signals but also can be trained and updated online. The proposed method is evaluated using both offline experiments and online AB tests, which involve very large collections of Twitter data and real Twitter users. Results of the experiments prove the effectiveness and efficiency of our solution, and its superiority over the current production model adopted by Twitter.
更多查看译文
关键词
Online Advertising,Social Media Stream,Click-through Prediction
AI 理解论文
溯源树
样例
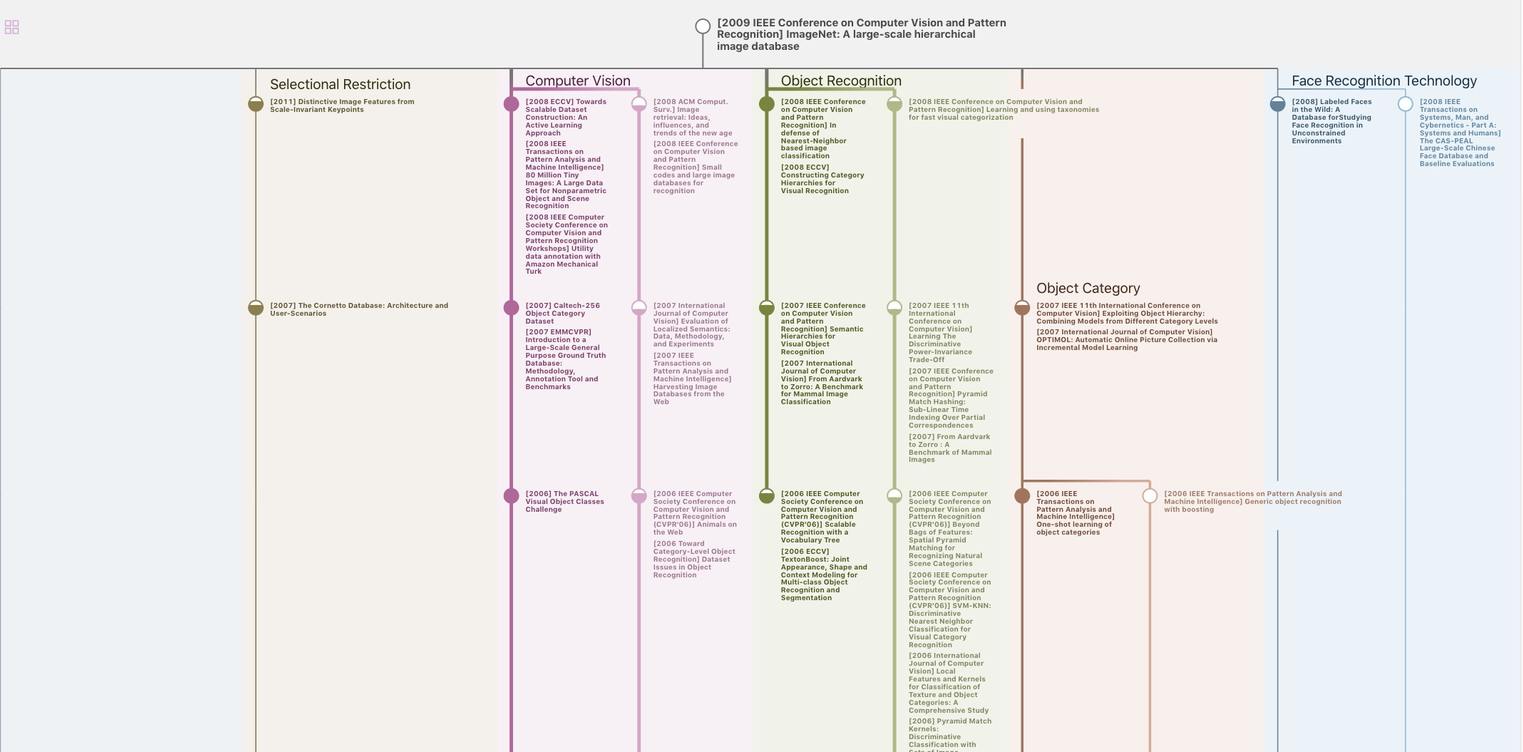
生成溯源树,研究论文发展脉络
Chat Paper
正在生成论文摘要