Citation Prediction Using Diverse Features.
ICDM Workshops(2015)
摘要
Using a large database of nearly 8 million bibliographic entries spanning over 3 million unique authors, we build predictive models to classify a paper based on its citation count. Our approach involves considering a diverse array of features including the interdisciplinarity of authors, which we quantify using Shannon entropy and Jensen-Shannon divergence. Rather than rely on subject codes, we model the disciplinary preferences of each author by estimating the author's journal distribution. We conduct an exploratory data analysis on the relationship between these interdisciplinarity variables and citation counts. In addition, we model the effects of (1) each author's influence in coauthorship graphs, and (2) words in the title of the paper. We then build classifiers for two-and three-class classification problems that correspond to predicting the interval in which a paper's citation count will lie. We use cross-validation and a true test set to tune model parameters and assess model performance. The best model we build, a classification tree, yields test set accuracies of 0.87 and 0.66, respectively. Using this model, we also provide rankings of attribute importance, for the three-class problem, these rankings indicate the importance of our interdisciplinarity metrics in predicting citation counts.
更多查看译文
关键词
citation prediction,interdisciplinarity,large-scale bibliometrics
AI 理解论文
溯源树
样例
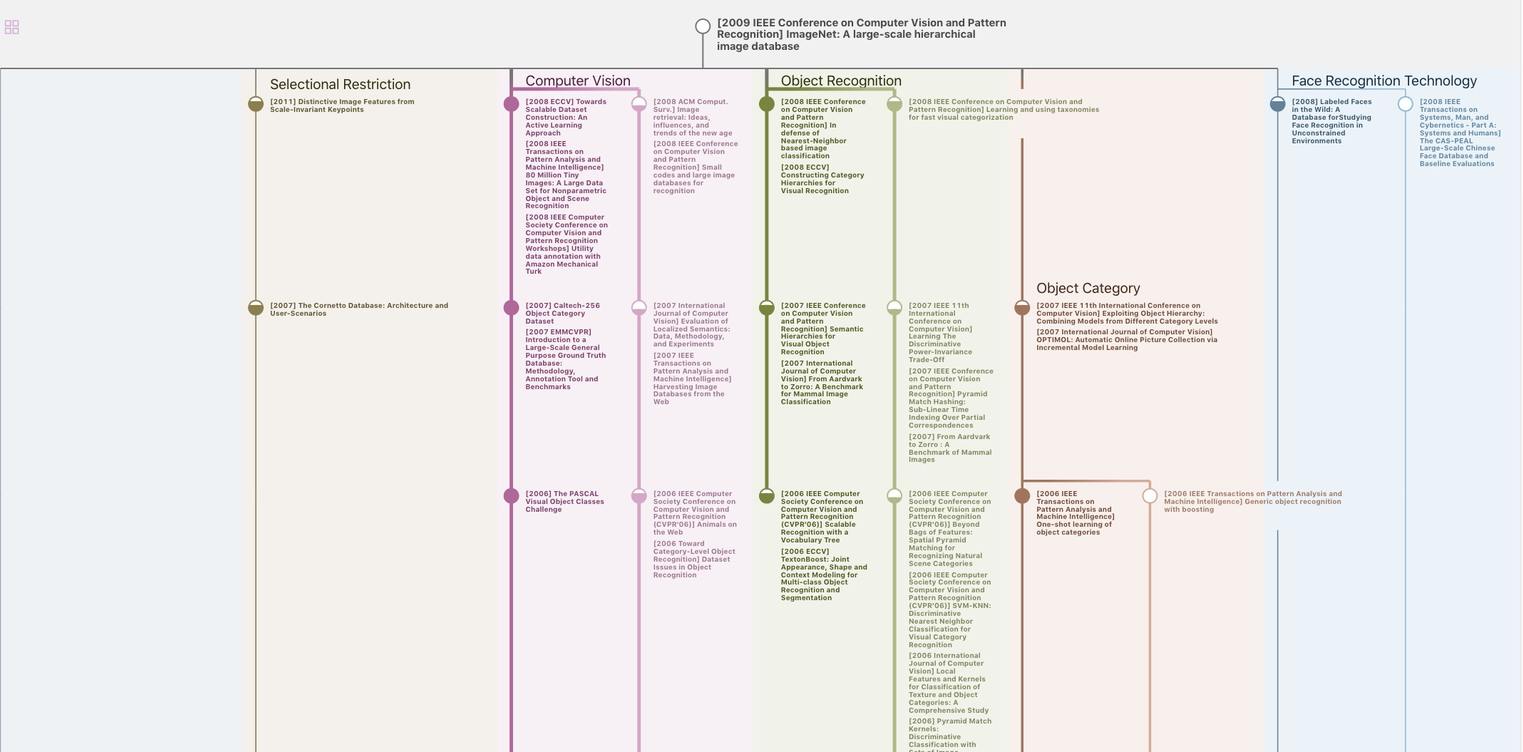
生成溯源树,研究论文发展脉络
Chat Paper
正在生成论文摘要