Labelwise versus Pairwise Decomposition in Label Ranking.
LWA(2013)
摘要
Label ranking is a specific type of preference learning problem, namely the problem of learning a model that maps instances to rankings over a finite set of predefined alternatives (labels). State-of-the-art approaches to label ranking include decomposition techniques that reduce the original problem to binary classification; ranking by pairwise comparison (RPC), for example, constructs one binary problem for each pair of alternatives. In general, each classification example refers to the pairwise comparison of two alternatives in a ranking. In this paper, we introduce a new (meta) learning technique for label ranking, which is based on a labelwise instead of a pairwise decomposition. The basic idea is to train one model per class label, namely a model that maps instances to ranks. Instead of a quadratic number of binary problems, like in RPC, this obviously gives rise to a linear number of ordinal classification problems. We propose a generalization of this approach for the practically relevant case in which the training data only contains incomplete rankings, that is, rankings of some but not all alternatives; in this case, only imprecise information about the rank of individual labels can be derived. Moreover, we provide an experimental study, in which the pairwise and the labelwise decomposition techniques are compared in a systematic way.
更多查看译文
关键词
labelwise ranking,pairwise decomposition
AI 理解论文
溯源树
样例
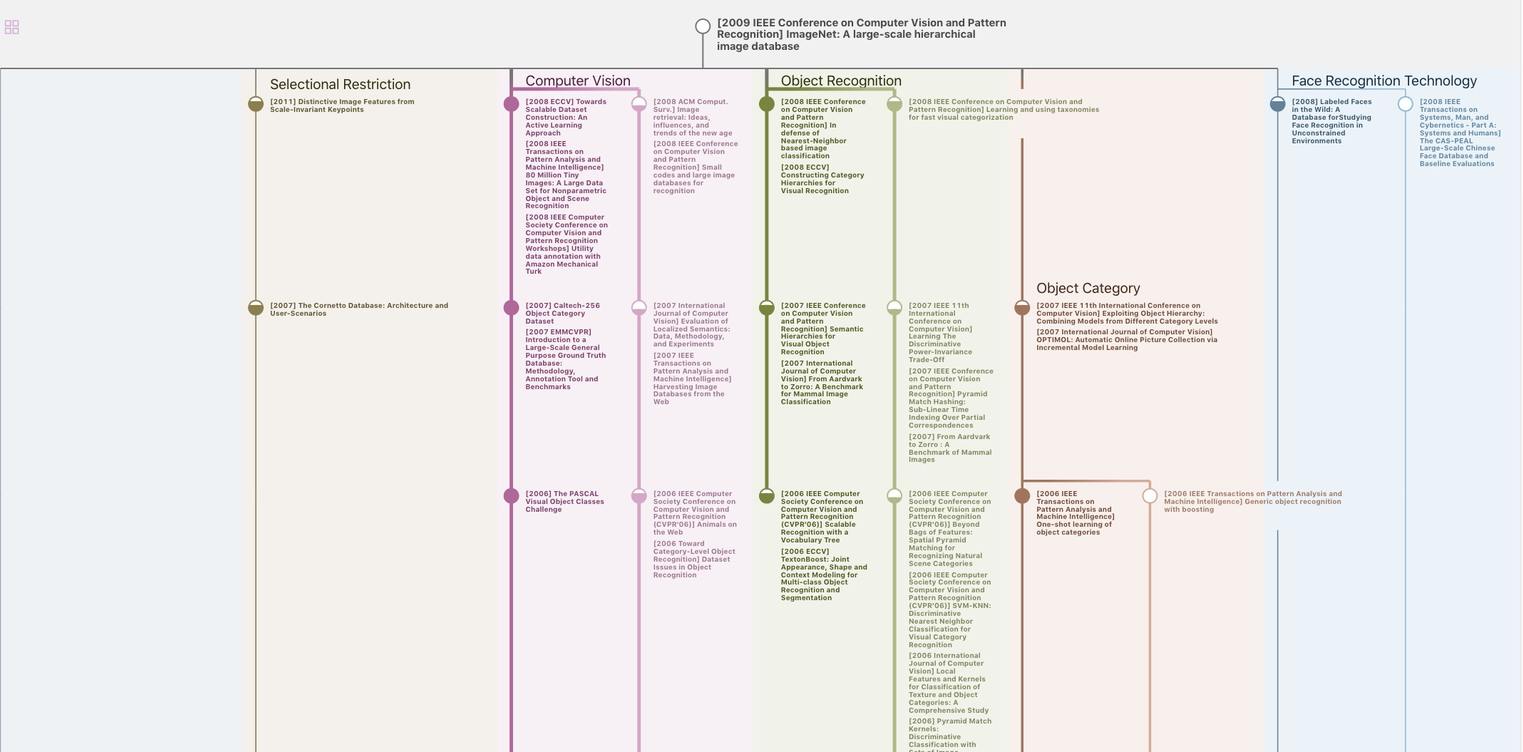
生成溯源树,研究论文发展脉络
Chat Paper
正在生成论文摘要