A Graph-Based Approach to Topic Clustering for Online Comments to News.
ECIR(2016)
摘要
This paper investigates graph-based approaches to labeled topic clustering of reader comments in online news. For graph-based clustering we propose a linear regression model of similarity between the graph nodes (comments) based on similarity features and weights trained using automatically derived training data. To label the clusters our graph-based approach makes use of DBPedia to abstract topics extracted from the clusters. We evaluate the clustering approach against gold standard data created by human annotators and compare its results against LDA – currently reported as the best method for the news comment clustering task. Evaluation of cluster labelling is set up as a retrieval task, where human annotators are asked to identify the best cluster given a cluster label. Our clustering approach significantly outperforms the LDA baseline and our evaluation of abstract cluster labels shows that graph-based approaches are a promising method of creating labeled clusters of news comments, although we still find cases where the automatically generated abstractive labels are insufficient to allow humans to correctly associate a label with its cluster.
更多查看译文
关键词
Comment Cluster, Latent Dirichlet Allocation, News Article, Cluster Label, Topic Cluster
AI 理解论文
溯源树
样例
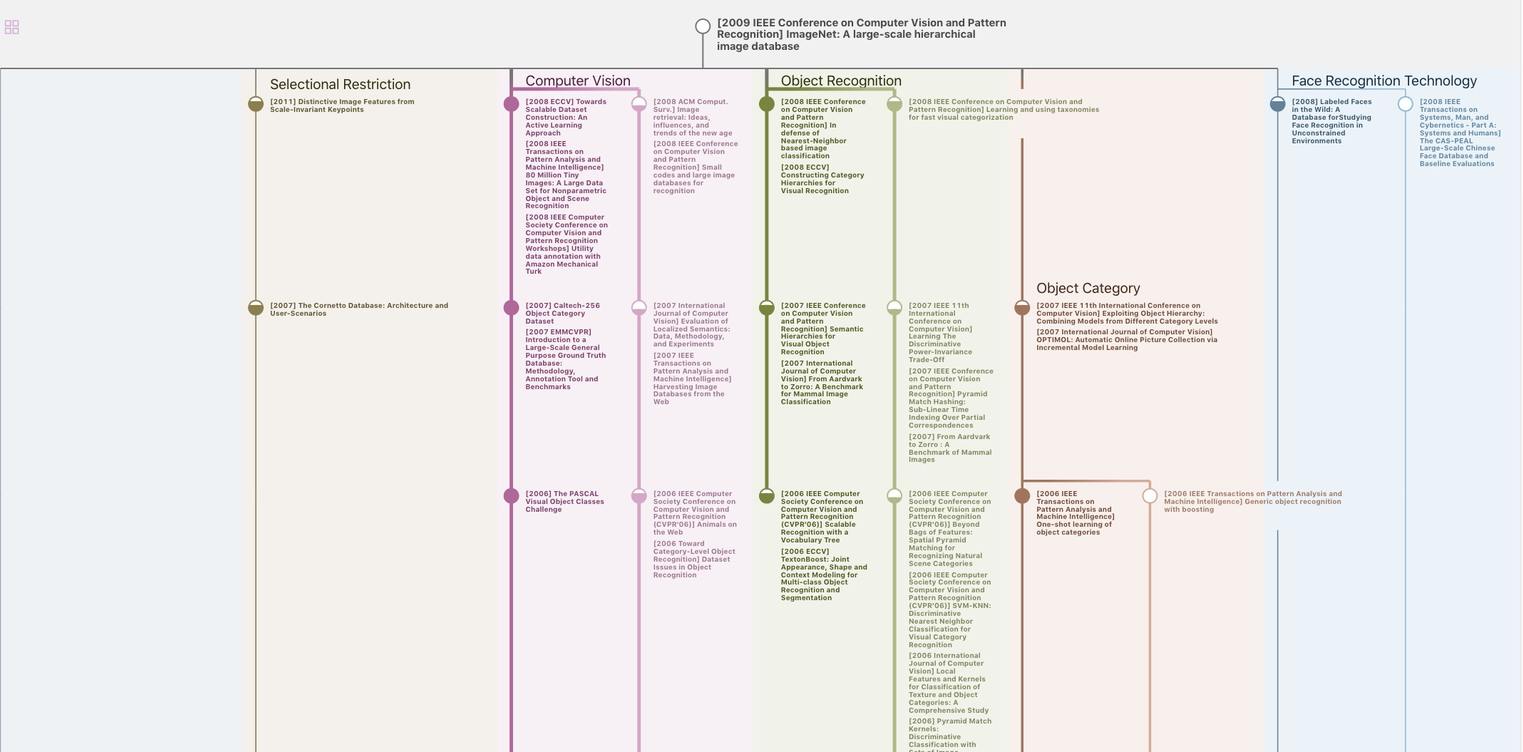
生成溯源树,研究论文发展脉络
Chat Paper
正在生成论文摘要