Using Latent Features To Measure The Diversity Of Recommendation Lists
2015 38th International Convention on Information and Communication Technology, Electronics and Microelectronics (MIPRO)(2015)
摘要
One of the well-known issues with content recommender system is that they tend to become overspecialized, which often has a negative influence on user experience. This can be solved by diversification of the recommendation list, a process that implements a tradeoff between accuracy and diversity of recommended items. Normally, item metadata is used in the diversity measure. In certain cases however, the item metadata may not be available thus a different approach to measure diversity is required. The aim of this preliminary study is to determine whether latent features can be used to measure the diversity of recommended items. In order to resolve this we generated recommendation lists for 43 different users using the LDOS-CoMoDa dataset. We then evaluated the diversity of these lists using the standard intra-list diversity measure. In addition we calculated the diversity of each list by comparing the latent features (calculated using the matrix factorization approach) of each item on the list. The comparison of both value sets showed that they show similar characteristics which implies that latent feature space offers an alternative method of evaluating item diversity when no metadata is present.
更多查看译文
关键词
recommendation list diversity measurement,content recommender system,user experience,recommended item accuracy,item metadata,LDOS-CoMoDa dataset,standard intralist diversity measure,matrix factorization approach,value sets,latent feature space,item diversity evaluation
AI 理解论文
溯源树
样例
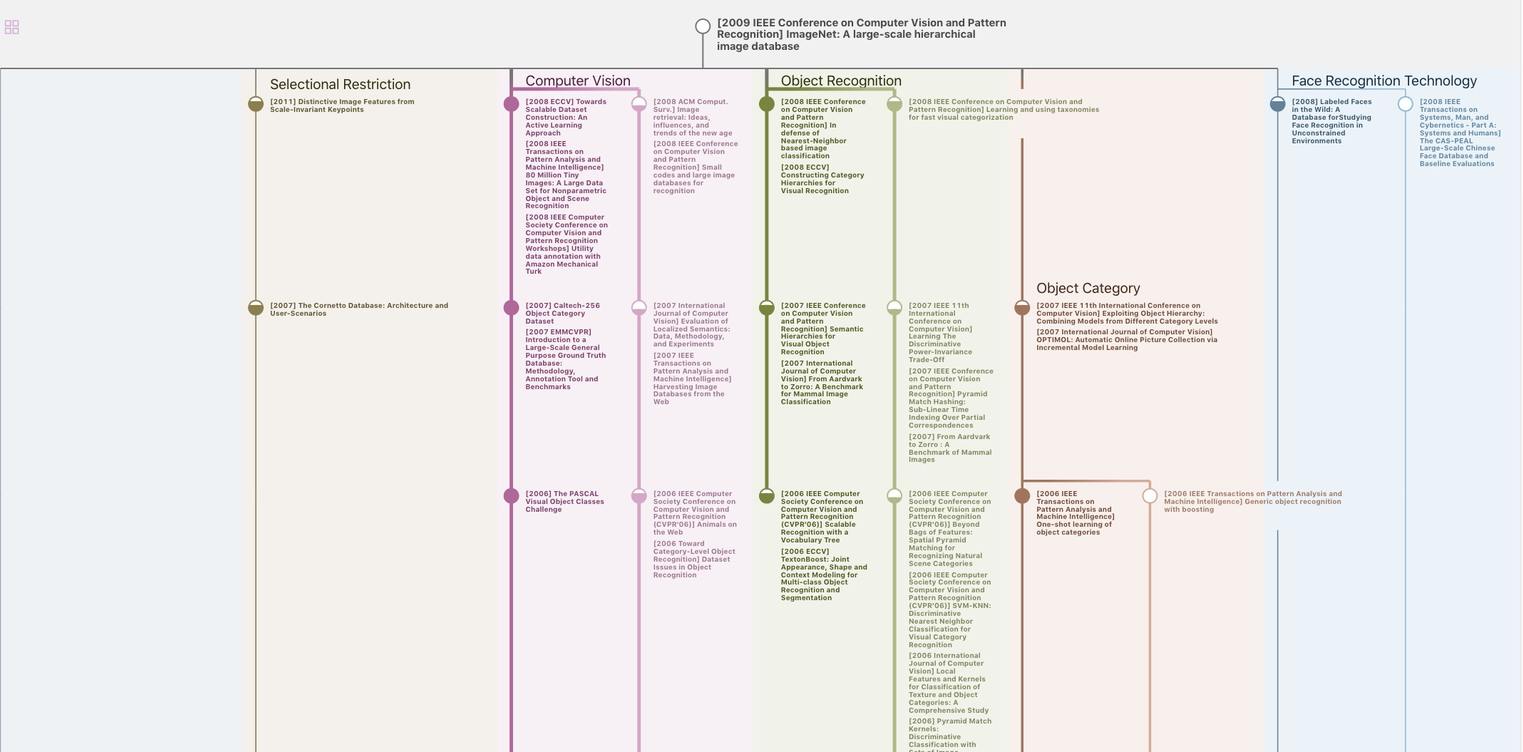
生成溯源树,研究论文发展脉络
Chat Paper
正在生成论文摘要