Conservative to confident: Treating uncertainty robustly within Learning-Based Control
2015 IEEE International Conference on Robotics and Automation (ICRA)(2015)
摘要
Robust control maintains stability and performance for a fixed amount of model uncertainty but can be conservative since the model is not updated online. Learning-based control, on the other hand, uses data to improve the model over time but is not typically guaranteed to be robust throughout the process. This paper proposes a novel combination of both ideas: a robust Min-Max Learning-Based Nonlinear Model Predictive Control (MM-LB-NMPC) algorithm. Based on an existing LB-NMPC algorithm, we present an efficient and robust extension, altering the NMPC performance objective to optimize for the worst-case scenario. The algorithm uses a simple a priori vehicle model and a learned disturbance model. Disturbances are modelled as a Gaussian Process (GP) based on experience collected during previous trials as a function of system state, input, and other relevant variables. Nominal state sequences are predicted using an Unscented Transform and worst-case scenarios are defined as sequences bounding the 3σ confidence region. Localization for the controller is provided by an on-board, vision-based mapping and navigation system enabling operation in large-scale, GPS-denied environments. The paper presents experimental results from testing on a 50 kg skid-steered robot executing a path-tracking task. The results show reductions in maximum lateral and heading path-tracking errors by up to 30% and a clear transition from robust control when the model uncertainty is high to optimal control when model uncertainty is reduced.
更多查看译文
关键词
model uncertainty,stability,robust min-max learning-based nonlinear model predictive control algorithm,MM-LB-NMPC algorithm,a priori vehicle model,learned disturbance model,LB-NMPC algorithm,Gaussian process,GP,nominal state sequences,unscented transform,3σ confidence region,on-board vision-based mapping,navigation system,large-scale GPS-denied environments,path-tracking task,path-tracking errors,optimal control,outdoor mobile robots,system state function
AI 理解论文
溯源树
样例
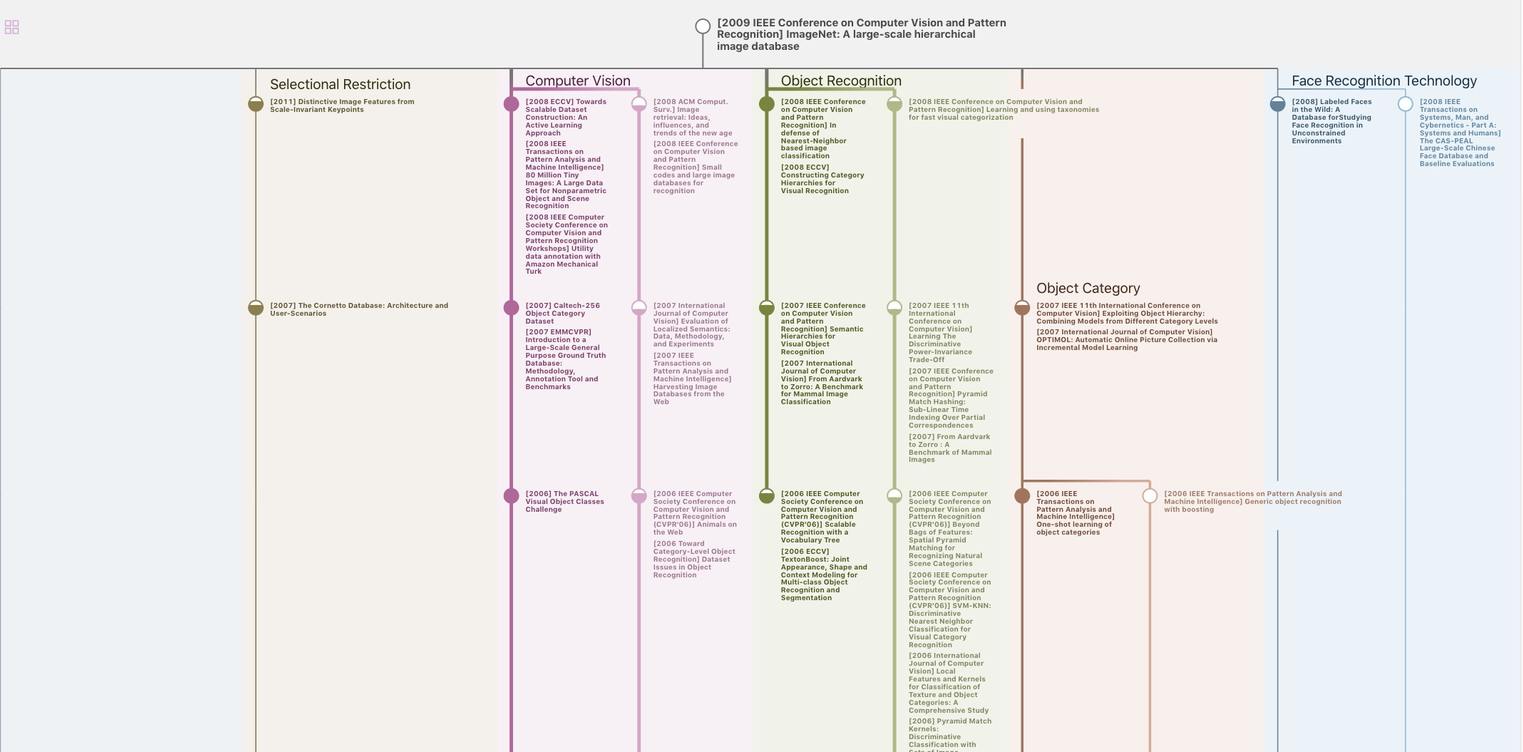
生成溯源树,研究论文发展脉络
Chat Paper
正在生成论文摘要