Sensor Fusion For Semantic Segmentation Of Urban Scenes
2015 IEEE International Conference on Robotics and Automation (ICRA)(2015)
摘要
Semantic understanding of environments is an important problem in robotics in general and intelligent autonomous systems in particular. In this paper, we propose a semantic segmentation algorithm which effectively fuses information from images and 3D point clouds. The proposed method incorporates information from multiple scales in an intuitive and effective manner. A late-fusion architecture is proposed to maximally leverage the training data in each modality. Finally, a pairwise Conditional Random Field (CRF) is used as a post-processing step to enforce spatial consistency in the structured prediction. The proposed algorithm is evaluated on the publicly available KITTI dataset [1] [2], augmented with additional pixel and point-wise semantic labels for building, sky, road, vegetation, sidewalk, car, pedestrian, cyclist, sign/ pole, and fence regions. A per-pixel accuracy of 89.3% and average class accuracy of 65.4% is achieved, well above current state-of-the-art [3].
更多查看译文
关键词
sensor fusion,urban scenes semantic segmentation,late-fusion architecture,conditional random field,pairwise CRF,KITTI dataset,point-wise semantic labels,3D point clouds
AI 理解论文
溯源树
样例
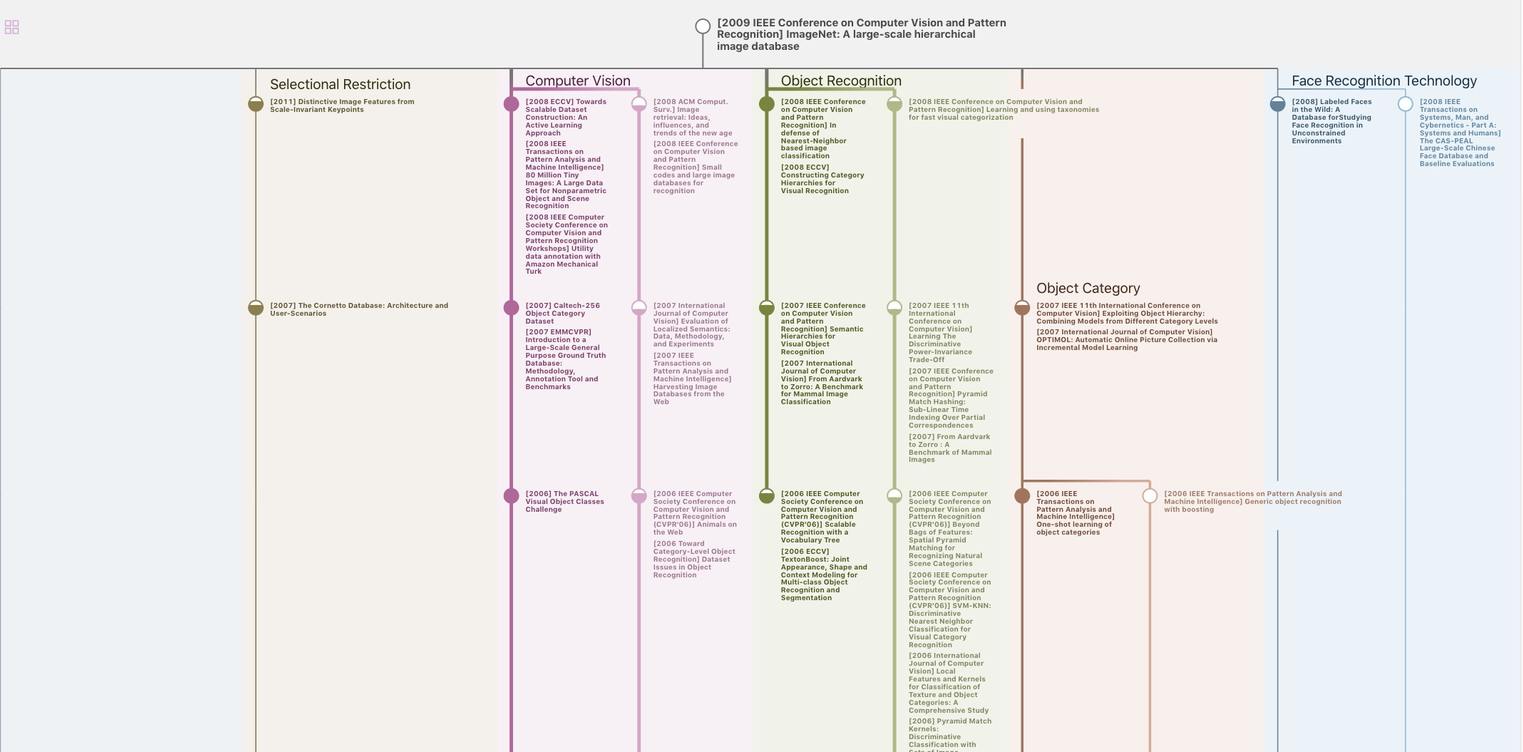
生成溯源树,研究论文发展脉络
Chat Paper
正在生成论文摘要