Clustering Dynamic Spatio-Temporal Patterns In The Presence Of Noise And Missing Data
IJCAI'15: Proceedings of the 24th International Conference on Artificial Intelligence(2015)
摘要
Clustering has gained widespread use, especially for static data. However, the rapid growth of spatio-temporal data from numerous instruments, such as earth-orbiting satellites, has created a need for spatio-temporal clustering methods to extract and monitor dynamic clusters. Dynamic spatio-temporal clustering faces two major challenges: First, the clusters are dynamic and may change in size, shape, and statistical properties over time. Second, numerous spatio-temporal data are incomplete, noisy, heterogeneous, and highly variable (over space and time). We propose a new spatio-temporal data mining paradigm, to autonomously identify dynamic spatio-temporal clusters in the presence of noise and missing data. Our proposed approach is more robust than traditional clustering and image segmentation techniques in the case of dynamic patterns, non-stationary, heterogeneity, and missing data. We demonstrate our method's performance on a real-world application of monitoring in-land water bodies on a global scale.
更多查看译文
AI 理解论文
溯源树
样例
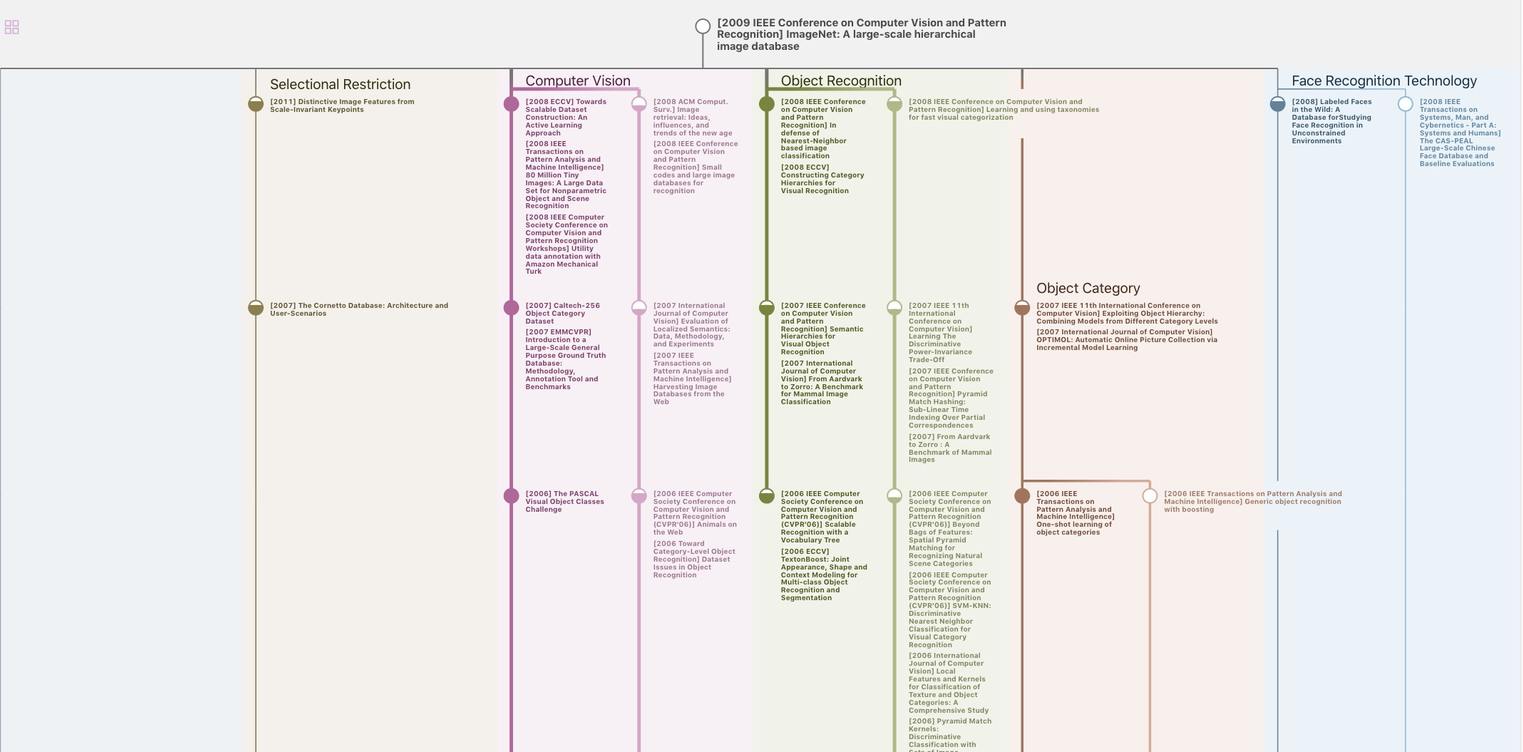
生成溯源树,研究论文发展脉络
Chat Paper
正在生成论文摘要