Influence Maximization In Human-Intervened Social Networks
SocInf'15: Proceedings of the 1st International Conference on Social Influence Analysis - Volume 1398(2015)
摘要
Recently there has been tremendous research on influence analysis in social networks: how to find initial topics or users to maximize the word-of-mouth effect that may be significant for advertising, viral marketing and other applications. Many researchers focus on the problem of influence maximization on the static structure of the network and find a subset of early adopters which activate the influence diffusion across the network. Despite the progress in modeling and techniques, how the incentives improve the network structure to enlarge the influence diffusion has been largely overlooked. In this paper, we introduce a novel problem which extends the influence maximization to the situation that the network structure can be varied in case of some incentives such as fans trading by compensating the web users to be fans in social networks. Providing that the presented problem is NP-hard, we propose two approximate approaches to solve the problem of influence maximization in dynamic networks. The first is a two-stage approach which separates the problem into two sub problems and solves them respectively. The second is a joint influence diffusion algorithm so as to repair the network structure and find the corresponding initial subset of the individuals in the repaired social network simultaneously to maximize the influence. We performed experiments on social network data to provide evidence of the effectiveness of the proposed methods.
更多查看译文
AI 理解论文
溯源树
样例
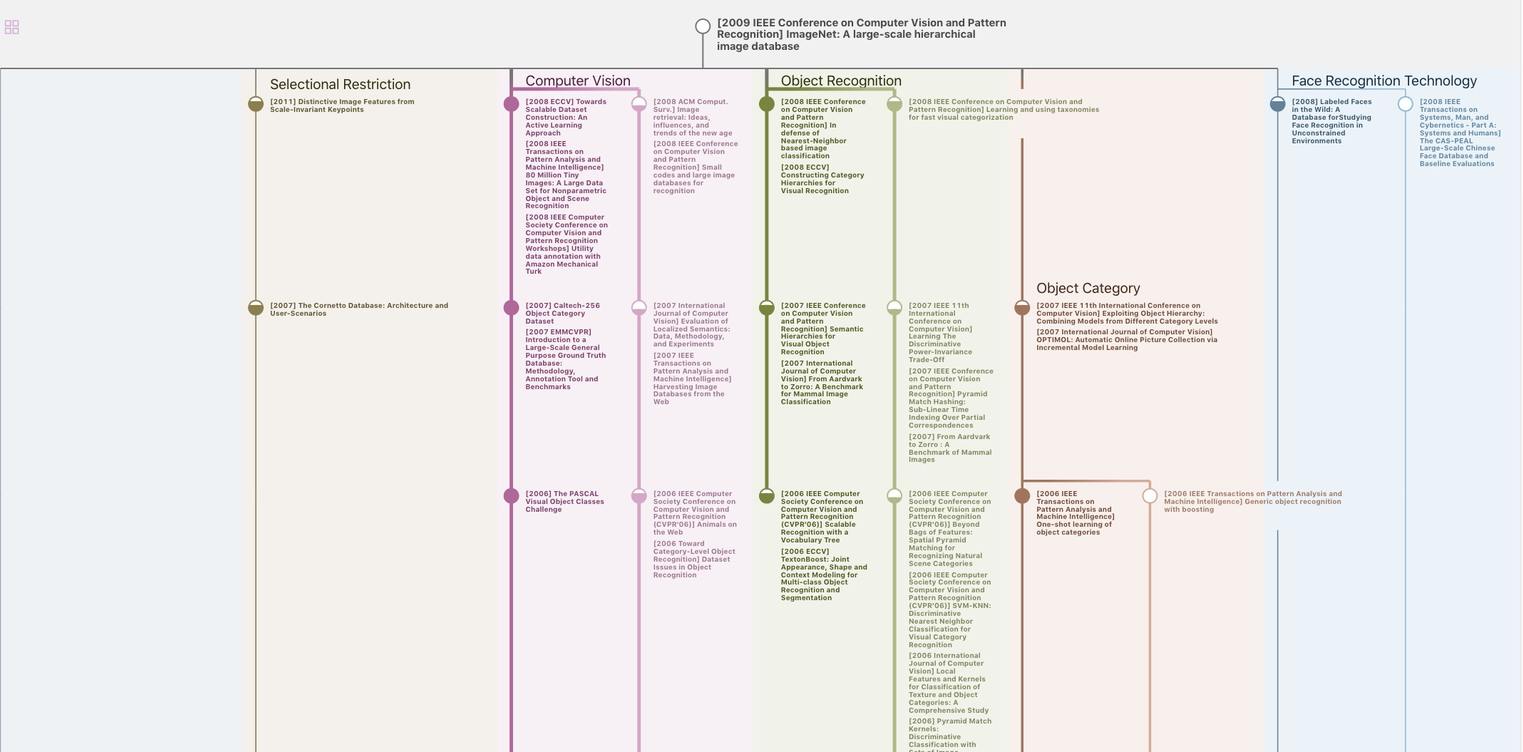
生成溯源树,研究论文发展脉络
Chat Paper
正在生成论文摘要