Managing Monotonicity In Classification By A Pruned Random Forest
INTELLIGENT DATA ENGINEERING AND AUTOMATED LEARNING - IDEAL 2015(2015)
摘要
In ordinal monotonic classification problems, the class variable should increase according to a subset of explanatory variables. Standard classifiers do not guarantee to produce model that satisfy the monotonicity constraints. Some algorithms have been developed to manage this issue, such as decision trees which have modified the growing and pruning mechanisms. In this contribution we study the suitability of using these mechanisms in the generation of Random Forests. We introduce a simple ensemble pruning mechanism based on the degree of monotonicity. After an exhaustive experimental analysis, we deduce that a Random Forest applied over these problems is able to achieve a slightly better predictive performance than standard algorithms.
更多查看译文
关键词
Monotonic classification,Decision tree induction,Random forest,Ensemble pruning
AI 理解论文
溯源树
样例
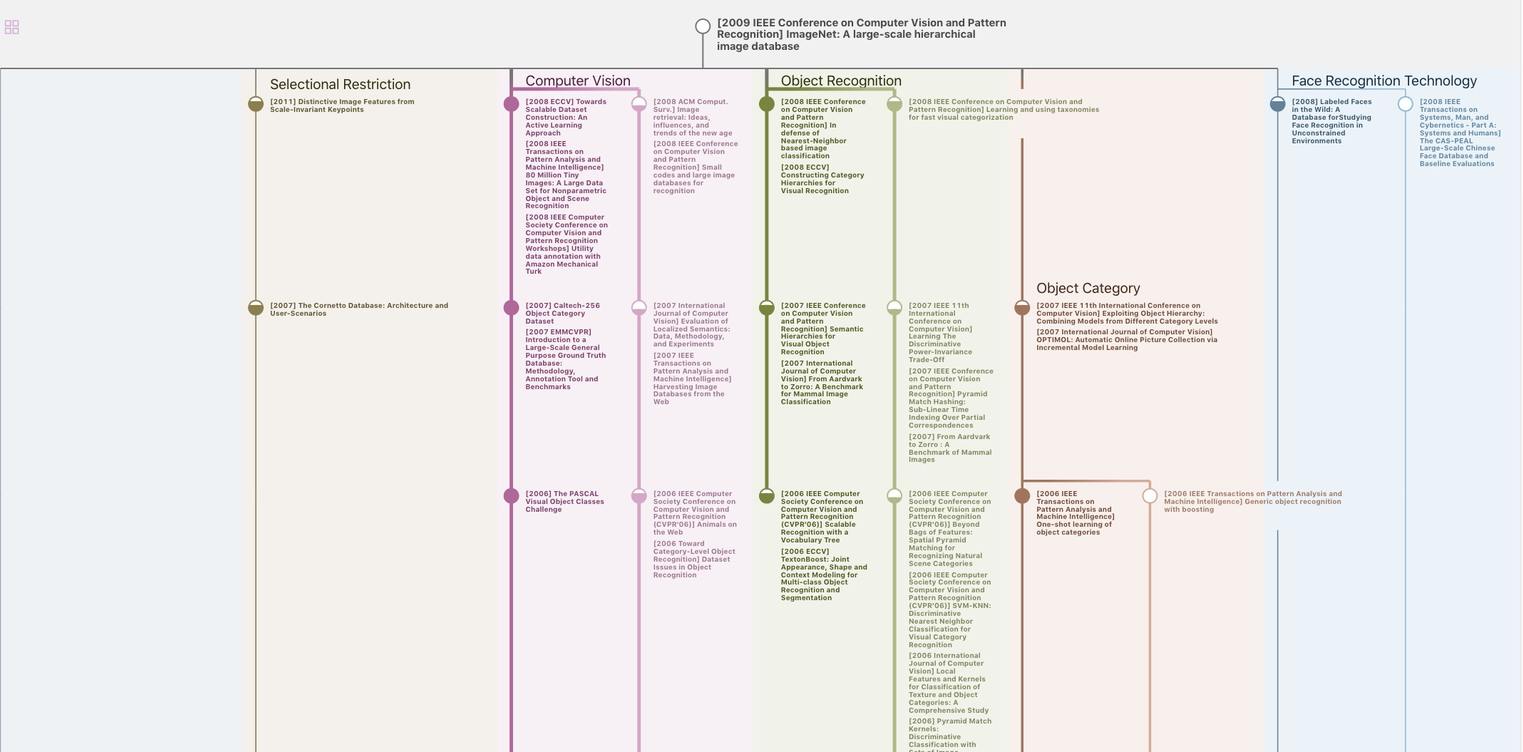
生成溯源树,研究论文发展脉络
Chat Paper
正在生成论文摘要