Surface defect detection in low-contrast images using basis image representation
2015 14th IAPR International Conference on Machine Vision Applications (MVA)(2015)
摘要
In this paper, we propose a machine vision approach for detecting local irregular brightness in low-contrast surface images and, especially, focus on mura (brightness non-uniformity) defects in Liquid Crystal Display (LCD) panels. A mura defect embedded in a low-contrast surface image shows no distinct intensity from its surrounding region, and even worse, the sensed image may also present uneven illumination on the surface. All these make the mura defect detection in low-contrast surface images extremely difficult. A set of basis images derived from defect-free surface images are used to represent the general appearance of a clear surface. An image to be inspected is then constructed as a linear combination of the basis images, and the coefficients of the combination form the feature vector for discriminating mura defects from clear surfaces. In order to find minimum number of basis images for efficient and effective representation, the basis images are designed such that they are both statistically independent and spatially exclusive. An independent component analysis-based model that finds both the maximum negentropy for statistical independency and minimum spatial correlation for spatial redundancy is proposed to extract the representative basis images. Experimental results have shown that the proposed method can effectively detect various mura defects in low-contrast LCD panel images.
更多查看译文
关键词
surface defect detection,basis image representation,machine vision approach,low-contrast surface images,liquid crystal display panels,mura defect,feature vector,independent component analysis-based model,maximum negentropy,statistical independency,minimum spatial correlation,spatial redundancy,LCD panel images
AI 理解论文
溯源树
样例
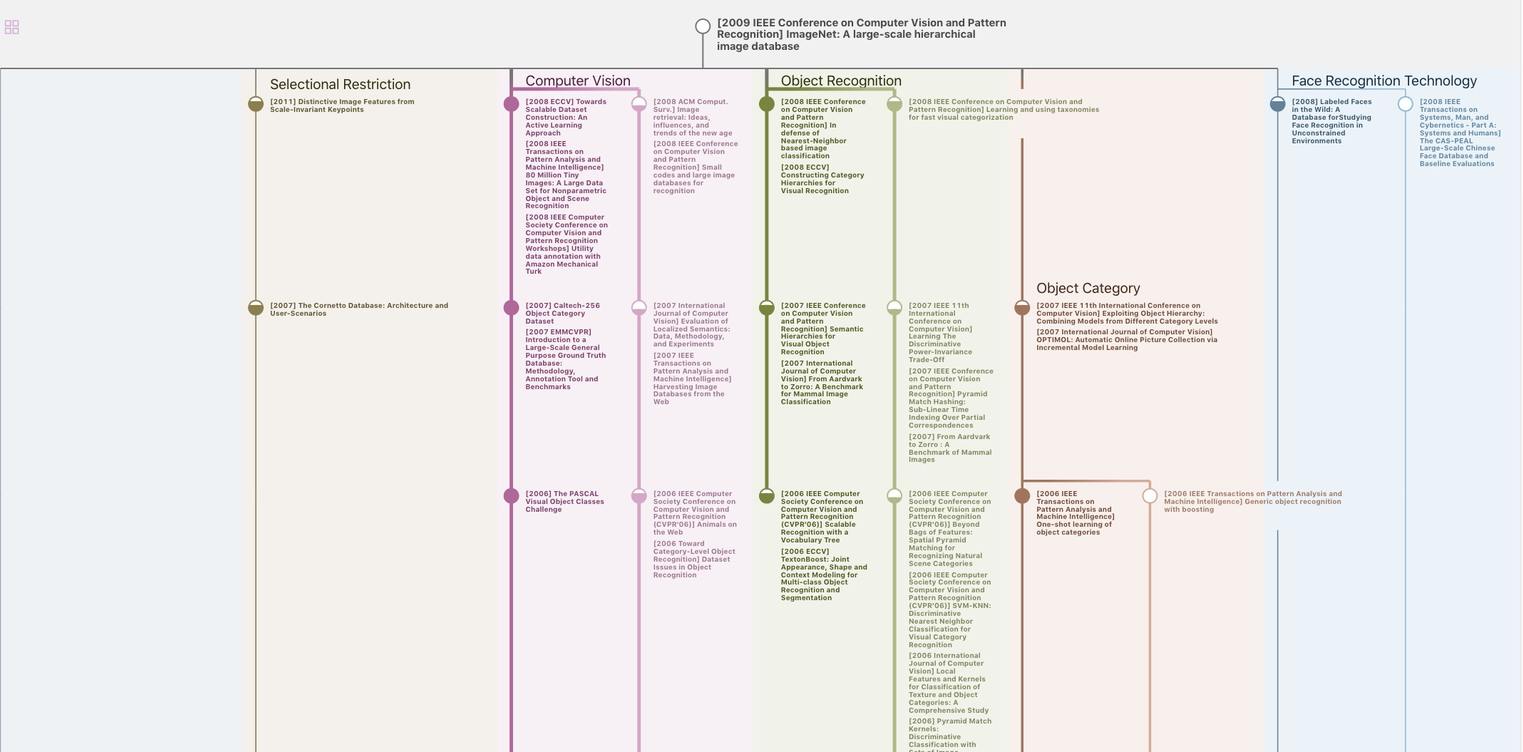
生成溯源树,研究论文发展脉络
Chat Paper
正在生成论文摘要