An End-To-End Approach To Language Identification In Short Utterances Using Convolutional Neural Networks
16TH ANNUAL CONFERENCE OF THE INTERNATIONAL SPEECH COMMUNICATION ASSOCIATION (INTERSPEECH 2015), VOLS 1-5(2015)
摘要
In this work, we propose an end-to-end approach to the language identification (LID) problem based on Convolutional Deep Neural Networks (CDNNs). The use of CDNNs is mainly motivated by the ability they have shown when modeling speech signals, and their relatively low-cost with respect to other deep architectures in terms of number of free parameters. We evaluate different configurations in a subset of 8 languages within the NIST Language Recognition Evaluation 2009 Voice of America (VOA) dataset, for the task of short test durations (segments up to 3 seconds of speech). The proposed CDNN-based systems achieve comparable performances to our baseline i-vector system, while reducing drastically the number of parameters to tune (at least 100 times fewer parameters). Then, we combine these CDNN-based systems and the i-vector baseline with a simple fusion at score level. This combination outperforms our best standalone system (up to 11% of relative improvement in terms of EER).
更多查看译文
AI 理解论文
溯源树
样例
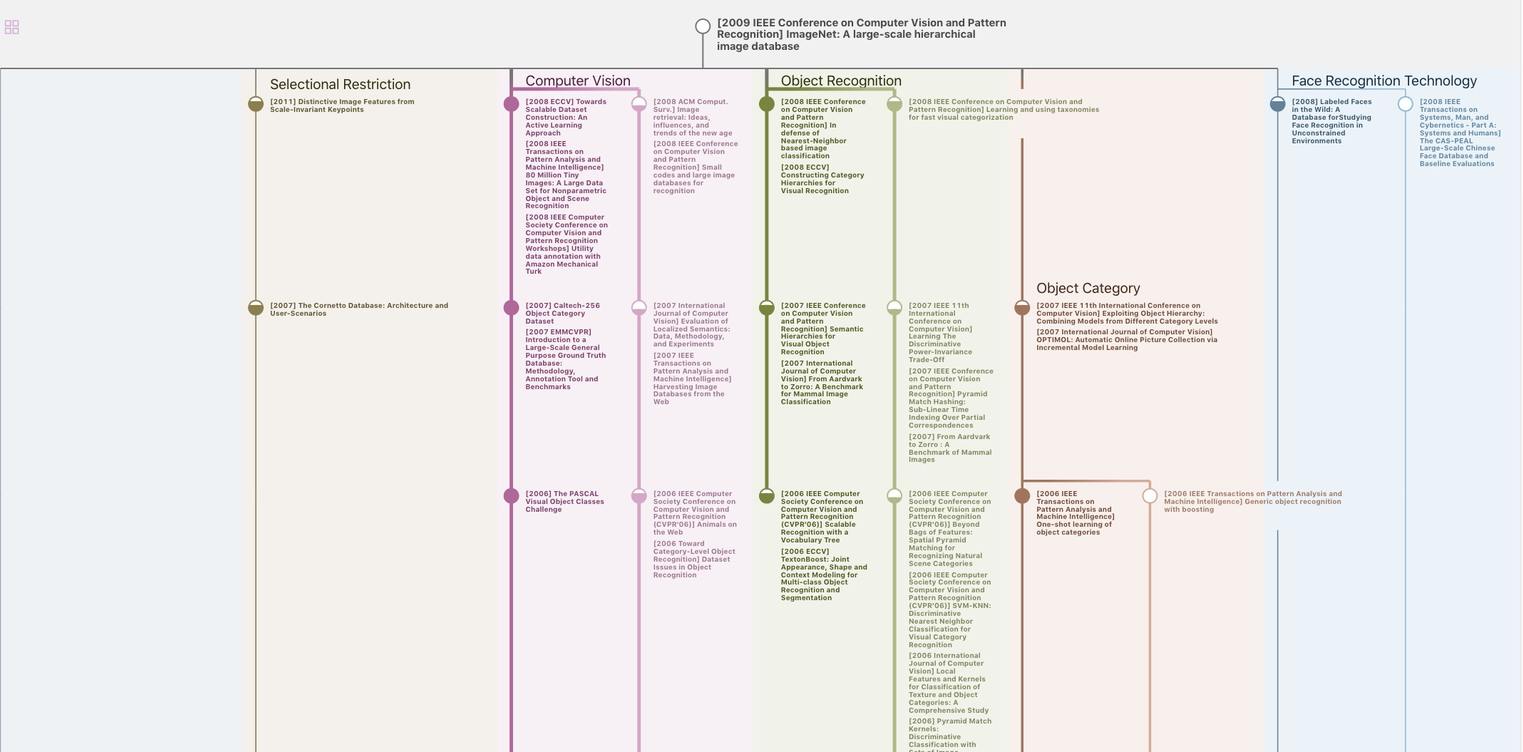
生成溯源树,研究论文发展脉络
Chat Paper
正在生成论文摘要