A stochastic density matrix approach to approximation of probability distributions and its application to nonlinear systems
2015 IEEE Conference on Control Applications (CCA)(2015)
摘要
This paper outlines an approach to the approximation of probability density functions by quadratic forms of weighted orthonormal basis functions with positive semi-definite Hermitian matrices of unit trace. Such matrices are called stochastic density matrices in order to reflect an analogy with the quantum mechanical density matrices. The SDM approximation of a PDF satisfies the normalization condition and is nonnegative everywhere in contrast to the truncated Gram-Charlier and Edgeworth expansions. For bases with an algebraic structure, such as the Hermite polynomial and Fourier bases, the SDM approximation can be chosen so as to satisfy given moment specifications and can be optimized using a quadratic proximity criterion. We apply the SDM approach to the Fokker-Planck-Kolmogorov PDF dynamics of Markov diffusion processes governed by nonlinear stochastic differential equations. This leads to an ordinary differential equation for the SDM dynamics of the approximating PDF. As an example, we consider the Smoluchowski SDE on a multidimensional torus.
更多查看译文
关键词
multidimensional torus,Smoluchowski SDE,ordinary differential equation,nonlinear stochastic differential equations,Markov diffusion processes,Fokker-Planck-Kolmogorov PDF dynamics,quadratic proximity criterion,moment specifications,Fourier bases,Hermite polynomial,algebraic structure,normalization condition,SDM approximation,stochastic density matrices,positive semidefinite Hermitian matrices,weighted orthonormal basis functions,quadratic forms,probability density function approximation,nonlinear systems,probability distributions,stochastic density matrix approach
AI 理解论文
溯源树
样例
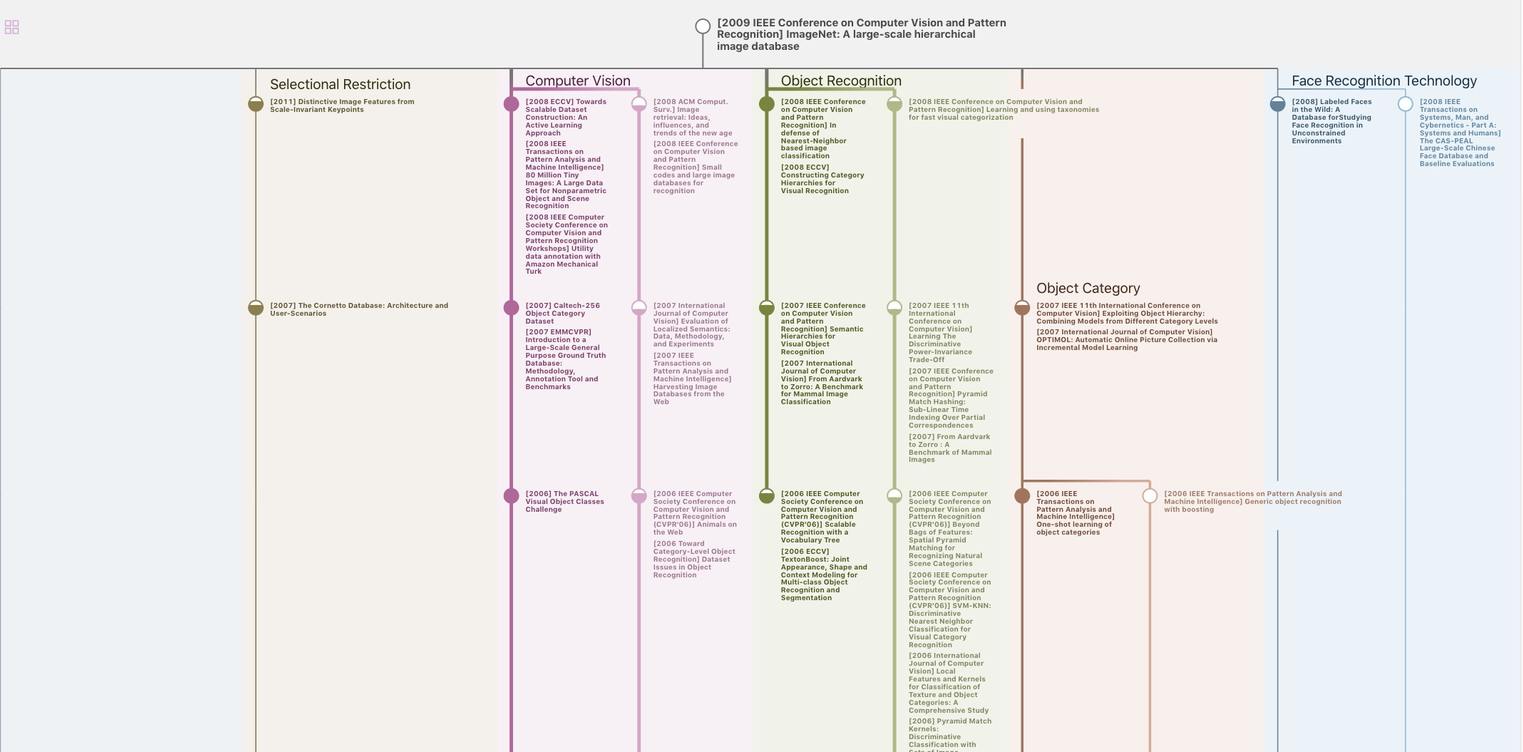
生成溯源树,研究论文发展脉络
Chat Paper
正在生成论文摘要