Predicting Multiple Structured Visual Interpretations
2015 IEEE International Conference on Computer Vision (ICCV)(2015)
摘要
We present a simple approach for producing a small number of structured visual outputs which have high recall, for a variety of tasks including monocular pose estimation and semantic scene segmentation. Current state-of-the-art approaches learn a single model and modify inference procedures to produce a small number of diverse predictions. We take the alternate route of modifying the learning procedure to directly optimize for good, high recall sequences of structured-output predictors. Our approach introduces no new parameters, naturally learns diverse predictions and is not tied to any specific structured learning or inference procedure. We leverage recent advances in the contextual submodular maximization literature to learn a sequence of predictors and empirically demonstrate the simplicity and performance of our approach on multiple challenging vision tasks including achieving state-of-the-art results on multiple predictions for monocular pose-estimation and image foreground/background segmentation.
更多查看译文
关键词
multiple structured visual interpretation,structured visual output,monocular pose estimation,semantic scene segmentation,inference procedure,learning procedure,structured-output predictor,diverse prediction,structured learning,submodular maximization literature,vision task,monocular pose-estimation,image foreground/background segmentation
AI 理解论文
溯源树
样例
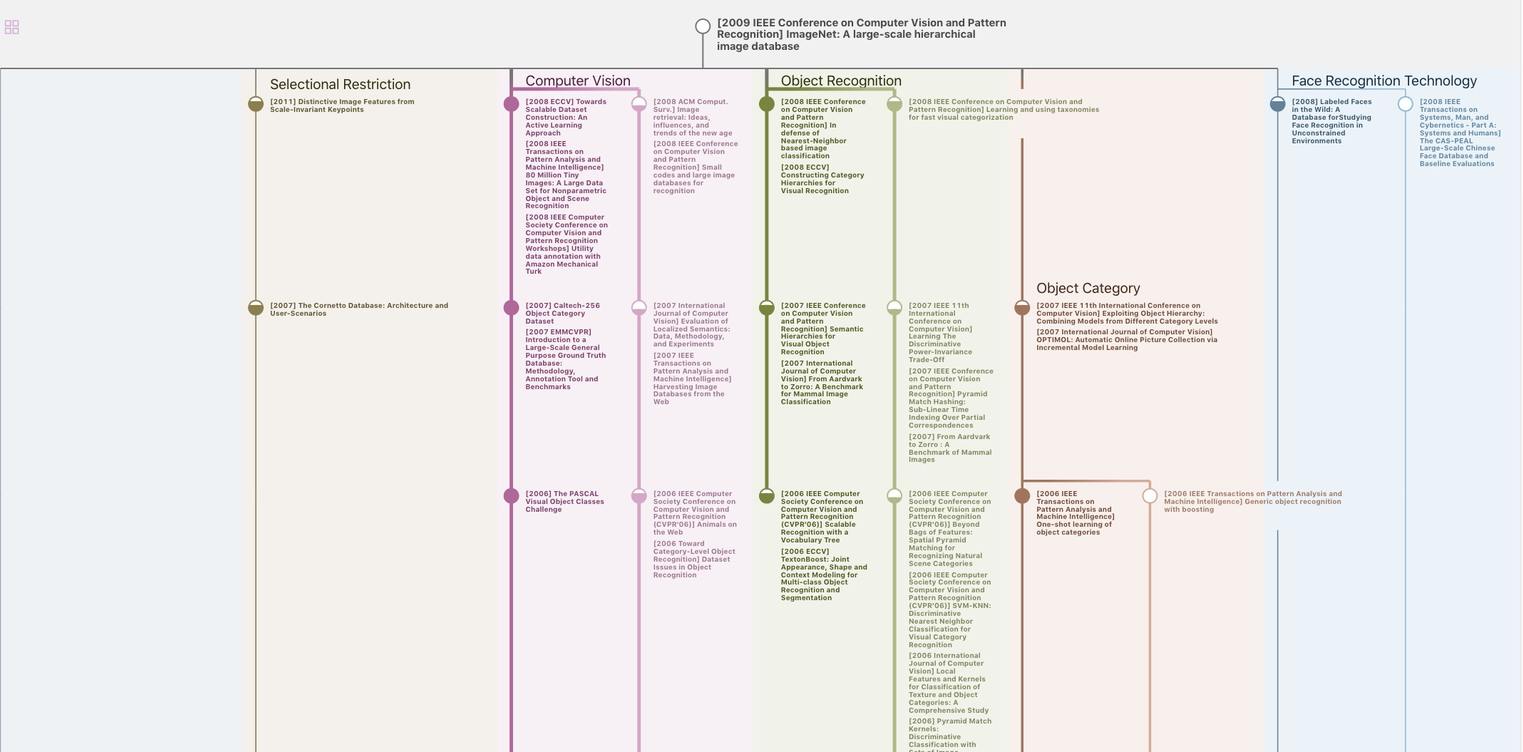
生成溯源树,研究论文发展脉络
Chat Paper
正在生成论文摘要