Patch Group Based Nonlocal Self-Similarity Prior Learning for Image Denoising
ICCV(2015)
摘要
Patch based image modeling has achieved a great success in low level vision such as image denoising. In particular, the use of image nonlocal self-similarity (NSS) prior, which refers to the fact that a local patch often has many nonlocal similar patches to it across the image, has significantly enhanced the denoising performance. However, in most existing methods only the NSS of input degraded image is exploited, while how to utilize the NSS of clean natural images is still an open problem. In this paper, we propose a patch group (PG) based NSS prior learning scheme to learn explicit NSS models from natural images for high performance denoising. PGs are extracted from training images by putting nonlocal similar patches into groups, and a PG based Gaussian Mixture Model (PG-GMM) learning algorithm is developed to learn the NSS prior. We demonstrate that, owe to the learned PG-GMM, a simple weighted sparse coding model, which has a closed-form solution, can be used to perform image denoising effectively, resulting in high PSNR measure, fast speed, and particularly the best visual quality among all competing methods.
更多查看译文
关键词
image denoising,image NSS prior,image enhancement,patch group based NSS prior learning scheme,PG-based NSS prior learning scheme,nonlocal similar patch,PG-based Gaussian mixture model learning algorithm,PG-GMM learning algorithm,weighted sparse coding model,PSNR,nonlocal self-similarity prior learning
AI 理解论文
溯源树
样例
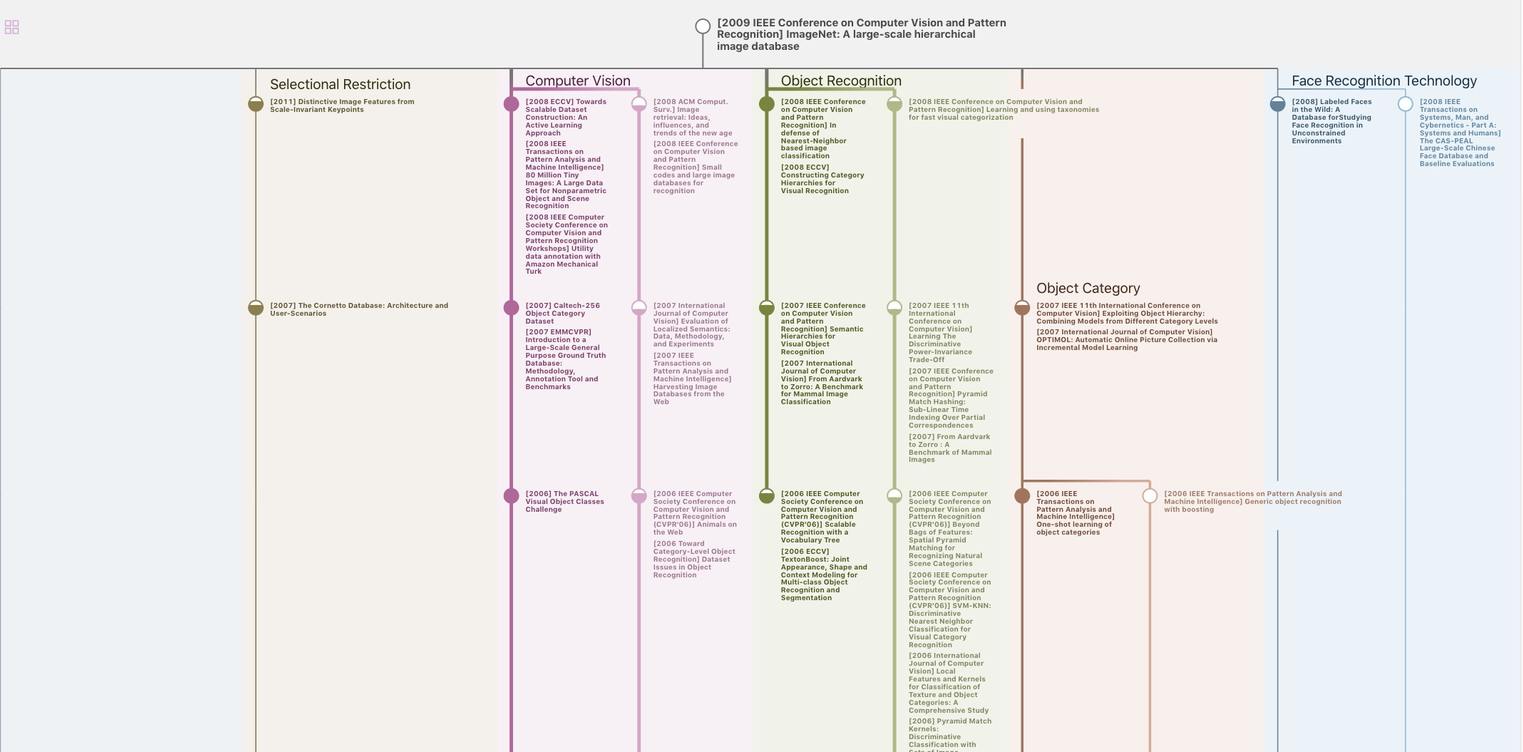
生成溯源树,研究论文发展脉络
Chat Paper
正在生成论文摘要