Learning Discriminative Reconstructions For Unsupervised Outlier Removal
2015 IEEE International Conference on Computer Vision (ICCV)(2015)
摘要
We study the problem of automatically removing outliers from noisy data, with application for removing outlier images from an image collection. We address this problem by utilizing the reconstruction errors of an autoencoder. We observe that when data are reconstructed from low-dimensional representations, the inliers and the outliers can be well separated according to their reconstruction errors. Based on this basic observation, we gradually inject discriminative information in the learning process of autoencoder to make the inliers and the outliers more separable. Experiments on a variety of image datasets validate our approach.
更多查看译文
关键词
discriminative reconstructions learning,unsupervised outlier removal,image collection,reconstruction errors,autoencoder,low-dimensional representations,image datasets
AI 理解论文
溯源树
样例
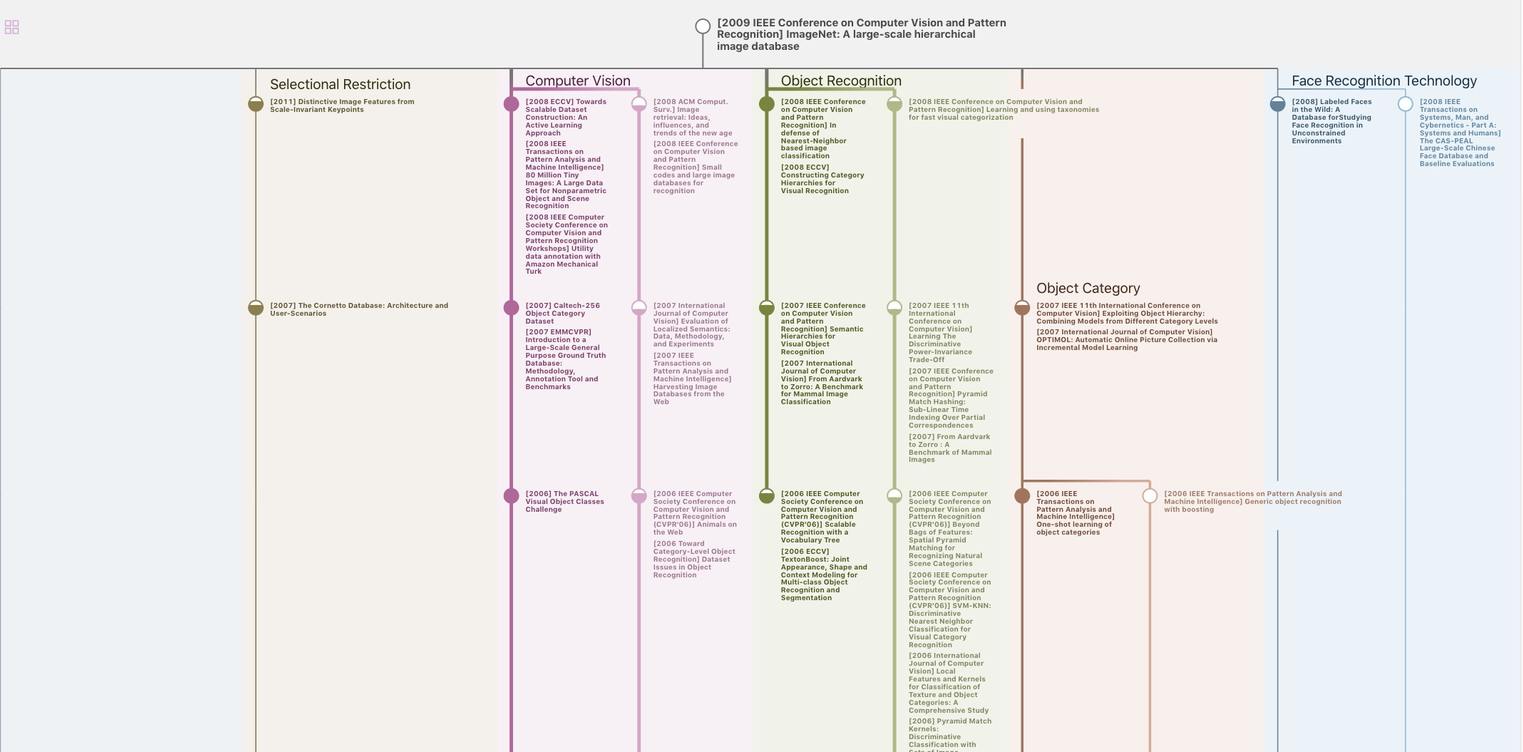
生成溯源树,研究论文发展脉络
Chat Paper
正在生成论文摘要