Constrained Convolutional Neural Networks for Weakly Supervised Segmentation
2015 IEEE International Conference on Computer Vision (ICCV)(2015)
摘要
We present an approach to learn a dense pixel-wise labeling from image-level tags. Each image-level tag imposes constraints on the output labeling of a Convolutional Neural Network (CNN) classifier. We propose Constrained CNN (CCNN), a method which uses a novel loss function to optimize for any set of linear constraints on the output space (i.e. predicted label distribution) of a CNN. Our loss formulation is easy to optimize and can be incorporated directly into standard stochastic gradient descent optimization. The key idea is to phrase the training objective as a biconvex optimization for linear models, which we then relax to nonlinear deep networks. Extensive experiments demonstrate the generality of our new learning framework. The constrained loss yields state-of-the-art results on weakly supervised semantic image segmentation. We further demonstrate that adding slightly more supervision can greatly improve the performance of the learning algorithm.
更多查看译文
关键词
constrained convolutional neural networks,dense pixel-wise labeling,image-level tags,CNN classifier,loss formulation,stochastic gradient descent optimization,learning framework,weakly supervised semantic image segmentation
AI 理解论文
溯源树
样例
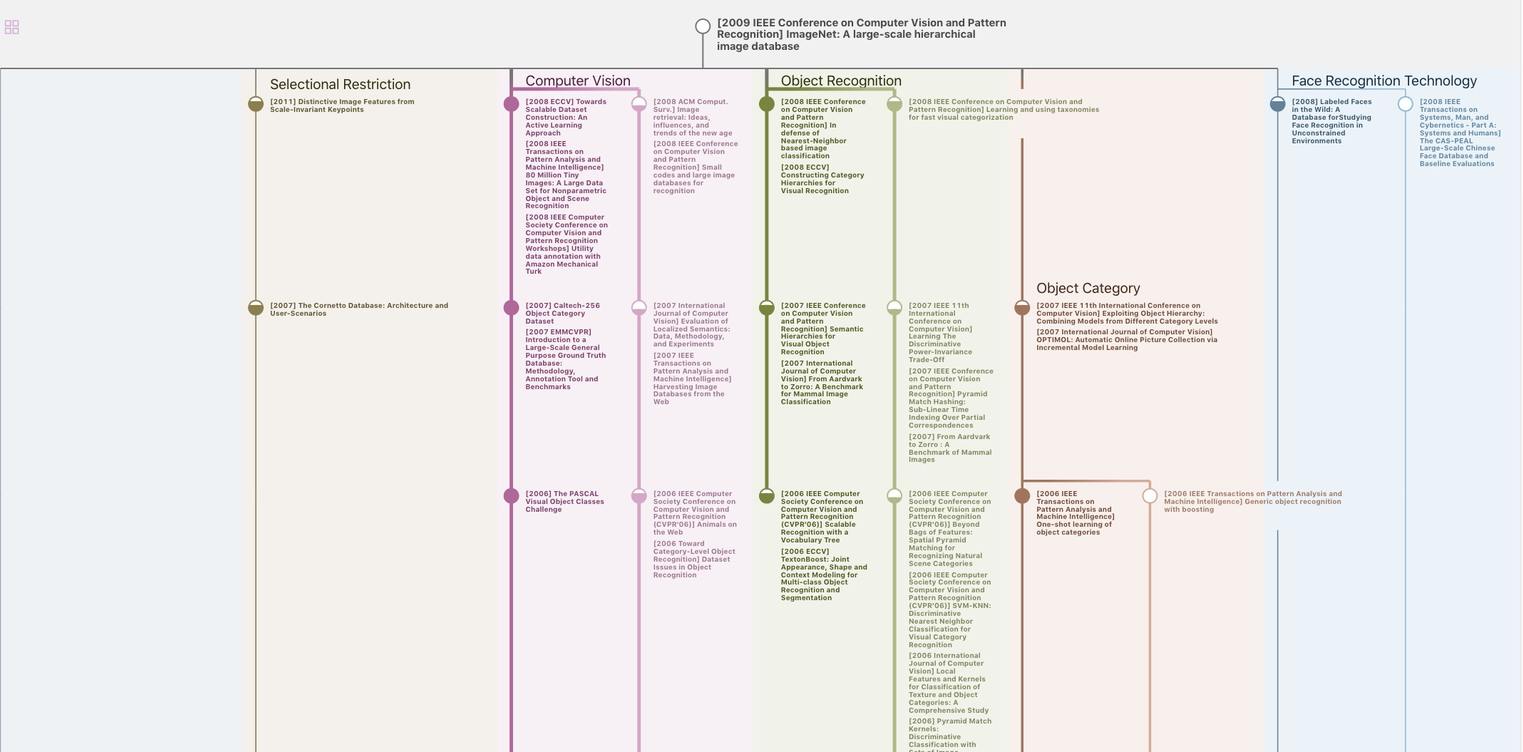
生成溯源树,研究论文发展脉络
Chat Paper
正在生成论文摘要