Semantic Segmentation Of Rgbd Images With Mutex Constraints
2015 IEEE International Conference on Computer Vision (ICCV)(2015)
摘要
In this paper, we address the problem of semantic scene segmentation of RGB-D images of indoor scenes. We propose a novel image region labeling method which augments CRF formulation with hard mutual exclusion (mutex) constraints. This way our approach can make use of rich and accurate 3D geometric structure coming from Kinect in a principled manner. The final labeling result must satisfy all mutex constraints, which allows us to eliminate configurations that violate common sense physics laws like placing a floor above a night stand. Three classes of mutex constraints are proposed: global object co-occurrence constraint, relative height relationship constraint, and local support relationship constraint. We evaluate our approach on the NYU-Depth V2 dataset, which consists of 1449 cluttered indoor scenes, and also test generalization of our model trained on NYU-Depth V2 dataset directly on a recent SUN3D dataset without any new training. The experimental results show that we significantly outperform the state-of-the-art methods in scene labeling on both datasets.
更多查看译文
关键词
semantic RGB-D image segmentation,Mutex constraints,semantic scene segmentation,image region labeling method,CRF formulation,3D geometric structure,Kinect,mutex constraints,global object cooccurrence constraint,local support relationship constraint,NYU-Depth V2 dataset,cluttered indoor scenes,test generalization,SUN3D dataset
AI 理解论文
溯源树
样例
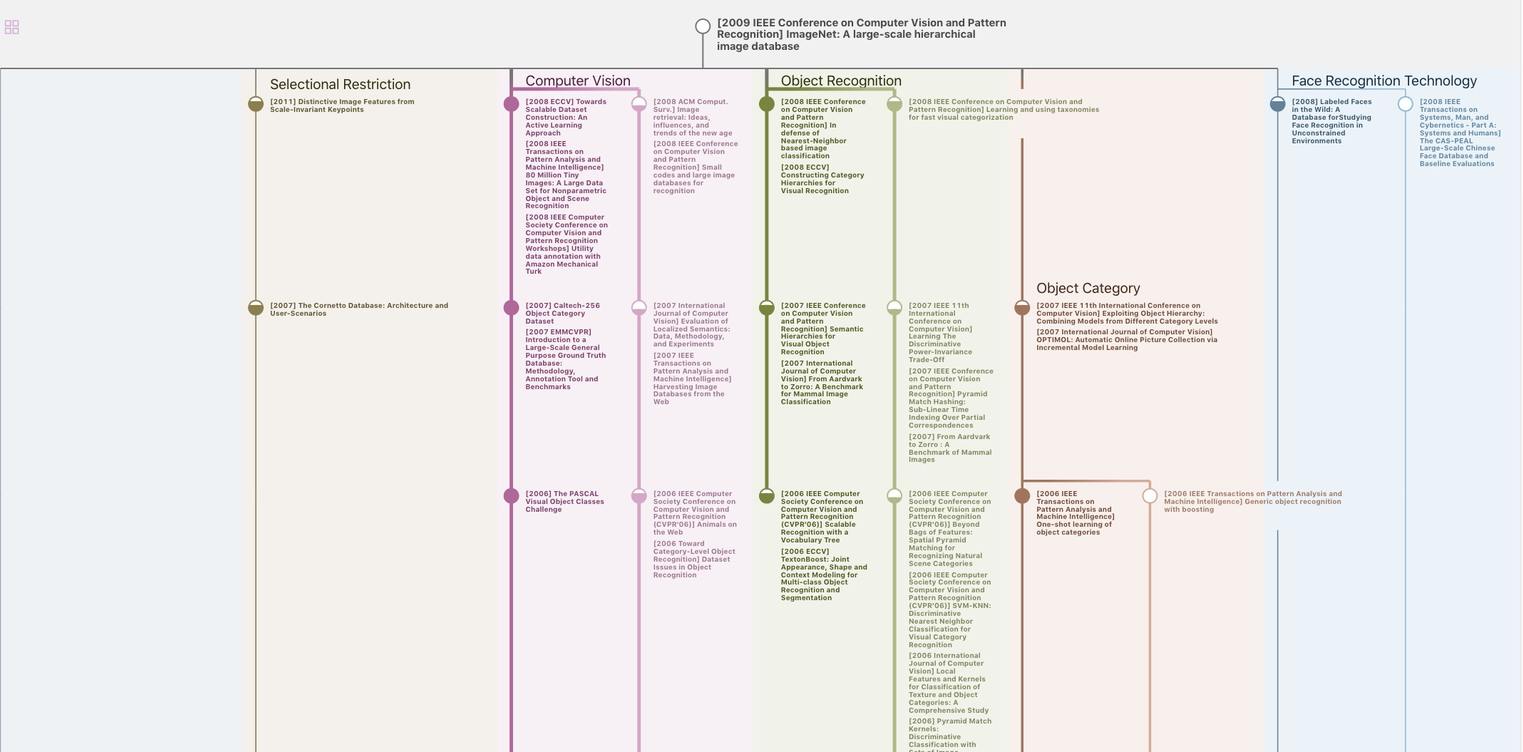
生成溯源树,研究论文发展脉络
Chat Paper
正在生成论文摘要