A Supervised Low-Rank Method For Learning Invariant Subspaces
2015 IEEE International Conference on Computer Vision (ICCV)(2015)
摘要
Sparse representation and low-rank matrix decomposition approaches have been successfully applied to several computer vision problems. They build a generative representation of the data, which often requires complex training as well as testing to be robust against data variations induced by nuisance factors. We introduce the invariant components, a discriminative representation invariant to nuisance factors, because it spans subspaces orthogonal to the space where nuisance factors are defined. This allows developing a framework based on geometry that ensures a uniform inter-class separation, and a very efficient and robust classification based on simple nearest neighbor. In addition, we show how the approach is equivalent to a local metric learning, where the local metrics (one for each class) are learned jointly, rather than independently, thus avoiding the risk of overfitting without the need for additional regularization. We evaluated the approach for face recognition with highly corrupted training and testing data, obtaining very promising results.
更多查看译文
关键词
supervised low-rank method,invariant subspace learning,sparse representation,low-rank matrix decomposition approaches,computer vision problems,uniform interclass separation,robust classification,simple nearest neighbor approach,local metric learning,face recognition
AI 理解论文
溯源树
样例
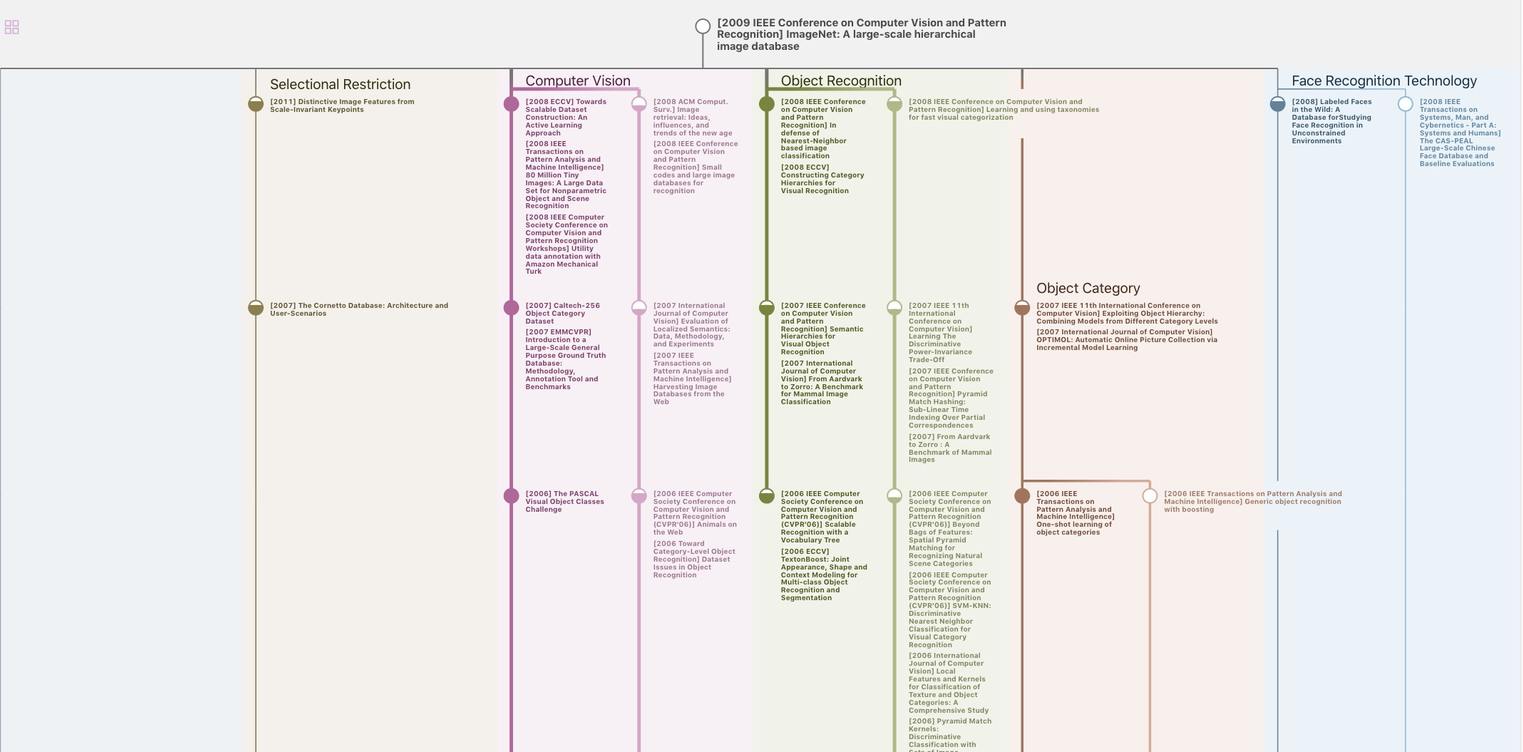
生成溯源树,研究论文发展脉络
Chat Paper
正在生成论文摘要