Multi-scale recognition with DAG-CNNs
2015 IEEE International Conference on Computer Vision (ICCV)(2015)
摘要
We explore multi-scale convolutional neural nets (CNNs) for image classification. Contemporary approaches extract features from a single output layer. By extracting features from multiple layers, one can simultaneously reason about high, mid, and low-level features during classification. The resulting multi-scale architecture can itself be seen as a feed-forward model that is structured as a directed acyclic graph (DAG-CNNs). We use DAG-CNNs to learn a set of multiscale features that can be effectively shared between coarse and fine-grained classification tasks. While fine-tuning such models helps performance, we show that even "off-the-self" multiscale features perform quite well. We present extensive analysis and demonstrate state-of-the-art classification performance on three standard scene benchmarks (SUN397, MIT67, and Scene15). In terms of the heavily benchmarked MIT67 and Scene15 datasets, our results reduce the lowest previously-reported error by 23.9% and 9.5%, respectively.
更多查看译文
关键词
multiscale recognition,DAG-CNN,multiscale convolutional neural nets,CNN,image classification,feature extraction,multiscale architecture,feed-forward model,directed acyclic graph,fine grained classification
AI 理解论文
溯源树
样例
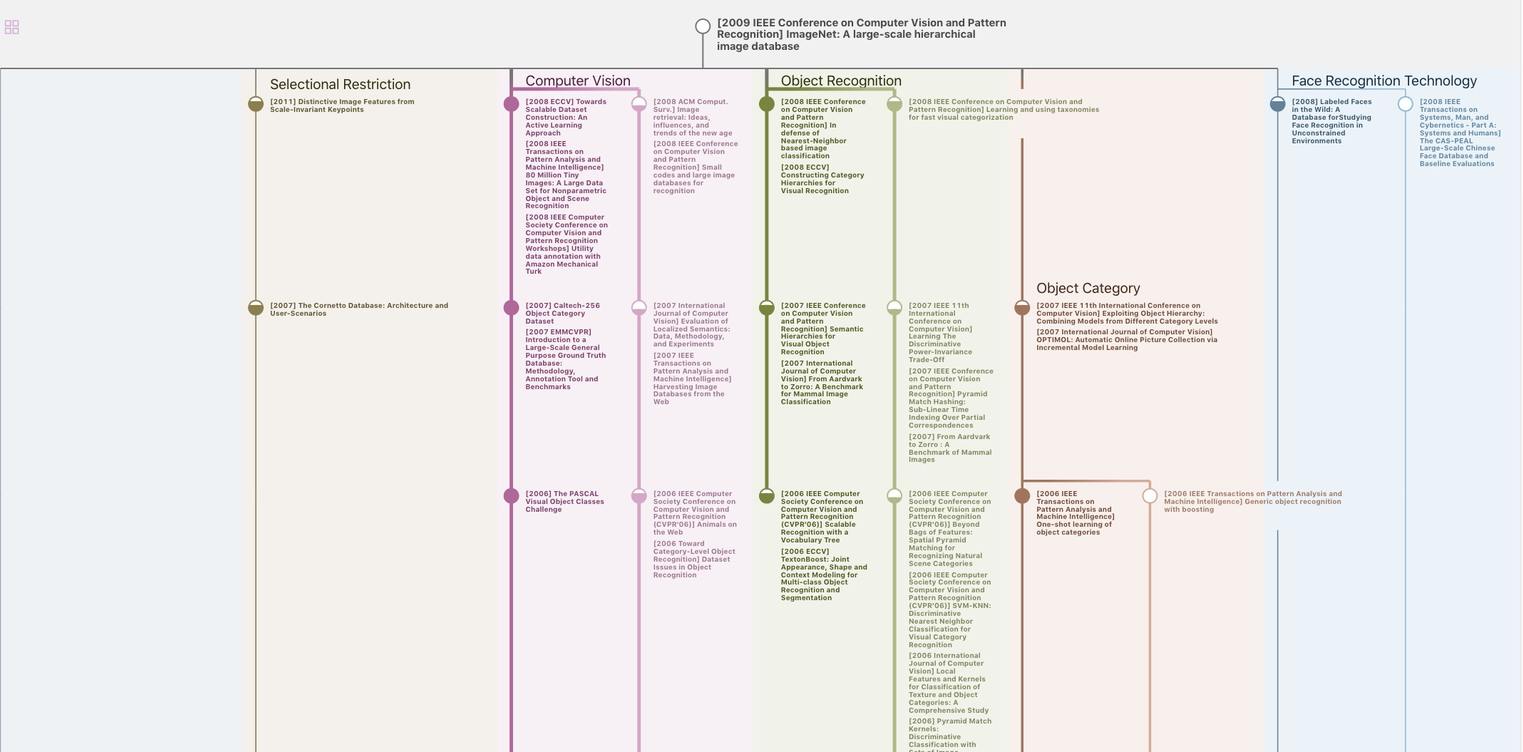
生成溯源树,研究论文发展脉络
Chat Paper
正在生成论文摘要