Multi-Label Cross-Modal Retrieval
2015 IEEE International Conference on Computer Vision (ICCV)(2015)
摘要
In this work, we address the problem of cross-modal retrieval in presence of multi-label annotations. In particular, we introduce multi-label Canonical Correlation Analysis (ml-CCA), an extension of CCA, for learning shared subspaces taking into account the high level semantic information in the form of multi-label annotations. Unlike CCA, ml-CCA does not rely on explicit pairings between the modalities, instead it uses the multi-label information to establish correspondences. This results in a discriminative subspace which is better suited for cross-modal retrieval tasks. We also present Fast ml-CCA, a computationally efficient version of ml-CCA, which is able to handle large scale datasets. We show the efficacy of our approach by conducting extensive cross-modal retrieval experiments on three standard benchmark datasets. The results show that the proposed approach achieves state-of-the-art retrieval performance on the three datasets.
更多查看译文
关键词
multilabel cross-modal retrieval,multilabel annotation,multilabel canonical correlation analysis,ml-CCA,subspace learning,semantic information
AI 理解论文
溯源树
样例
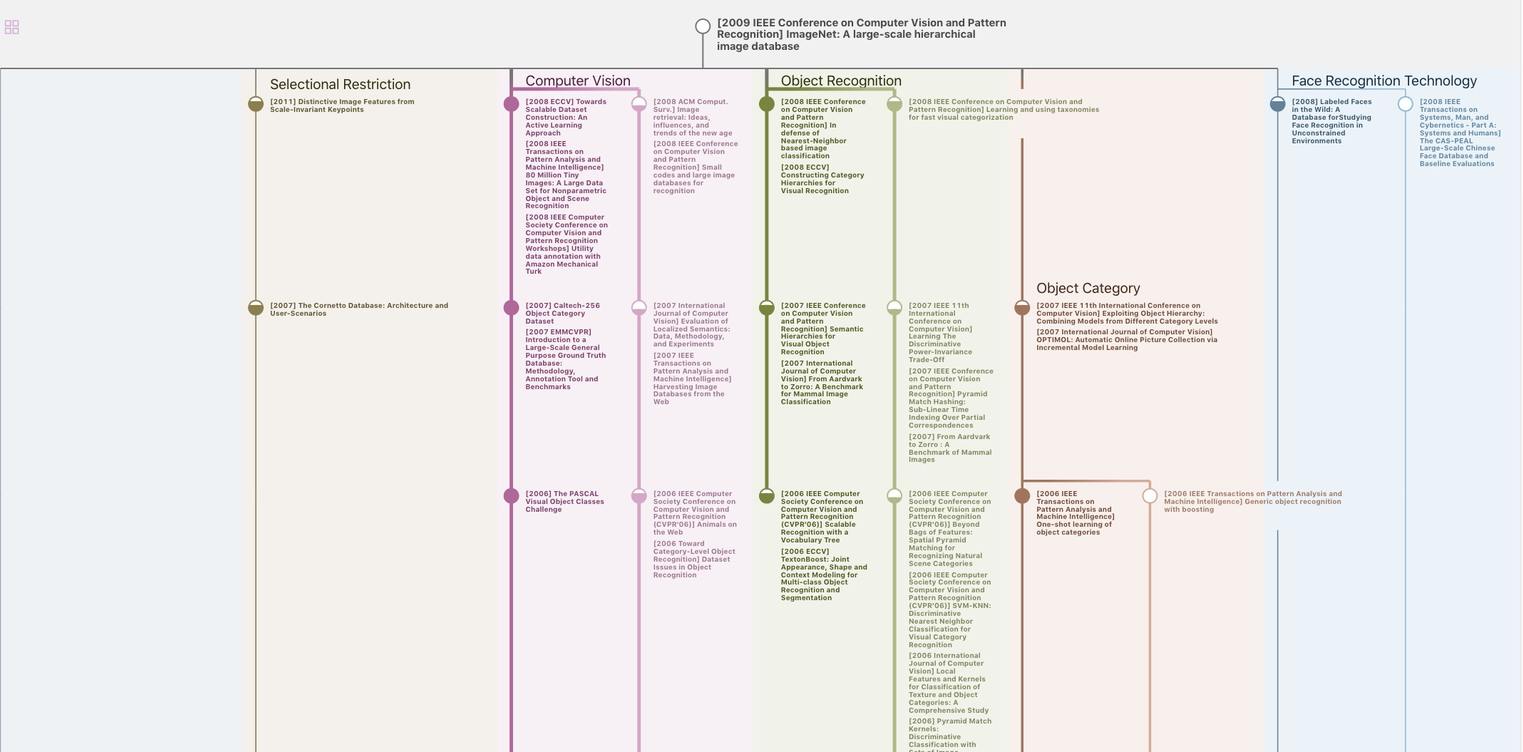
生成溯源树,研究论文发展脉络
Chat Paper
正在生成论文摘要