Wide-Area Image Geolocalization with Aerial Reference Imagery
ICCV(2015)
摘要
We propose to use deep convolutional neural networks to address the problem of cross-view image geolocalization, in which the geolocation of a ground-level query image is estimated by matching to georeferenced aerial images. We use state-of-the-art feature representations for ground-level images and introduce a cross-view training approach for learning a joint semantic feature representation for aerial images. We also propose a network architecture that fuses features extracted from aerial images at multiple spatial scales. To support training these networks, we introduce a massive database that contains pairs of aerial and ground-level images from across the United States. Our methods significantly out-perform the state of the art on two benchmark datasets. We also show, qualitatively, that the proposed feature representations are discriminative at both local and continental spatial scales.
更多查看译文
关键词
wide-area image geolocalization,aerial reference imagery,deep convolutional neural networks,cross-view image geolocalization,ground-level query image,georeferenced aerial image matching,state-of-the-art feature representations,cross-view training,joint semantic feature representation,network architecture,feature extraction,feature fusion,image database,United States,local spatial scales,continental spatial scales
AI 理解论文
溯源树
样例
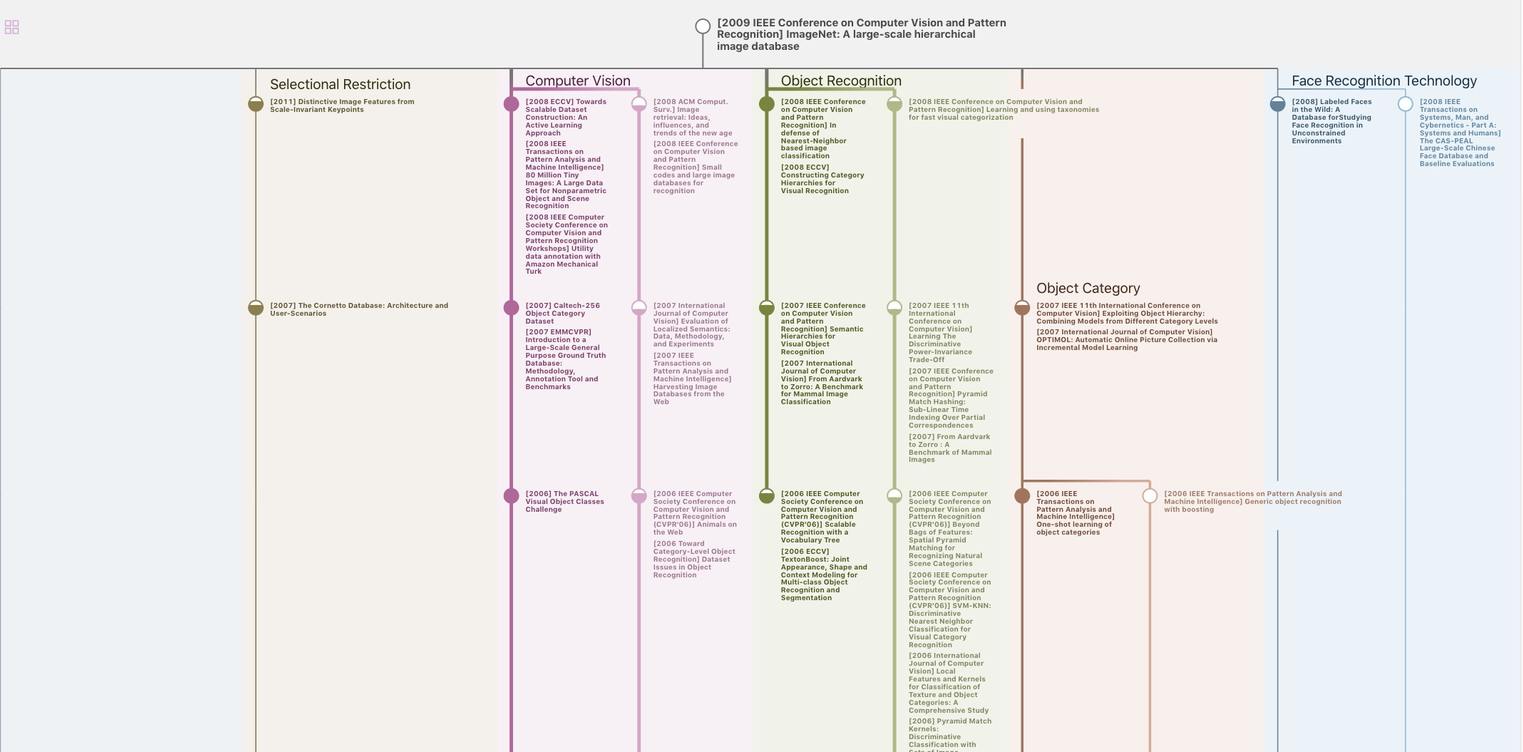
生成溯源树,研究论文发展脉络
Chat Paper
正在生成论文摘要