Learning in Multi-agent Systems with Sparse Interactions by Knowledge Transfer and Game Abstraction
Autonomous Agents and Multi-Agent Systems(2015)
摘要
In many multi-agent systems, the interactions between agents are sparse and exploiting interaction sparseness in multi-agent reinforcement learning (MARL) can improve the learning performance. Also, agents may have already learnt some single-agent knowledge (e.g., local value function) before the multi-agent learning process. In this work, we investigate how such knowledge can be utilized to learn better policies in multi-agent systems with sparse interactions. We adopt game theory-based MARL as the basic learning approach since it can coordinate agents better. We contribute three knowledge transfer mechanisms. The first one is value function transfer, which directly transfers agents' local value functions to the learning algorithm. The second one is selective value function transfer, which only transfers the value functions in states where the environmental dynamics change slightly. The last mechanism is model transfer-based game abstraction, which further improves the former two mechanisms by abstracting the one-shot game in each state and reducing equilibrium computation. Experimental results in benchmarks show that with the three knowledge transfer mechanisms, all of the tested game theory-based MARL algorithms are drastically improved and also achieve better asymptotic performance than the state-of-the-art algorithm CQ-learning.
更多查看译文
关键词
Multi-agent Reinforcement Learning, Knowledge Transfer, Multi-agent Systems, Sparse Interactions
AI 理解论文
溯源树
样例
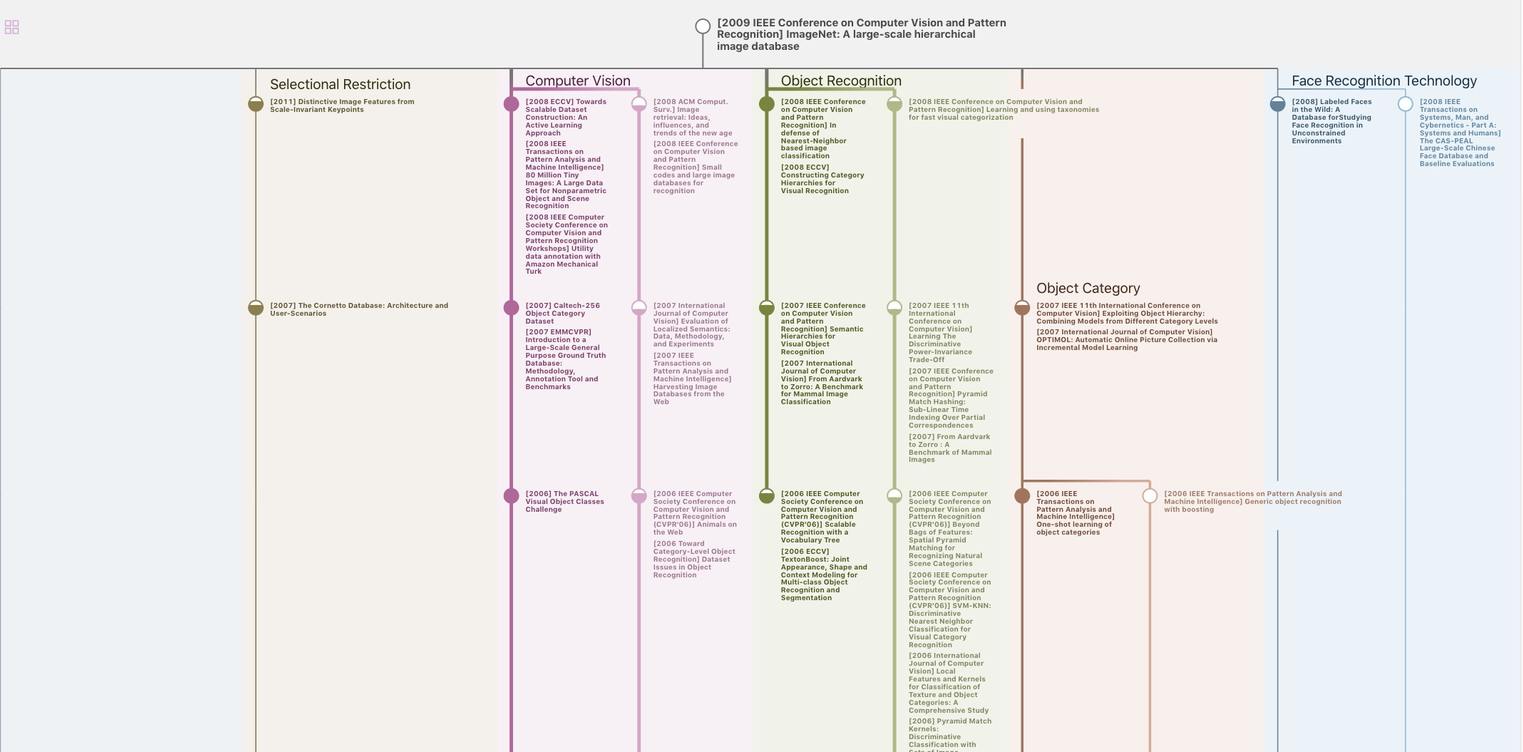
生成溯源树,研究论文发展脉络
Chat Paper
正在生成论文摘要