Combining Map-Based Inference and Crowd-Sensing for Detecting Traffic Regulators
IEEE International Conference on Mobile Ad hoc and Sensor Systems(2015)
摘要
Intelligent transportation systems serve as important technologies to improve traffic safety, mobility, cost and environmental sustainability. Towards that end, a variety of applications and driver advisory tools have been developed. To work efficiently, many require knowledge of not only street maps but also elements affecting traffic flow. The most obvious elements are traffic lights and stop signs, which we shall henceforth call traffic regulators. Unfortunately, information on traffic regulators is not widely available in public databases such as Open Street Map (OSM). Prior work described crowd-sourcing solutions to predict regulator type and locations. In this paper, we improve the prediction by offering a combination of map-based modeling and crowd-sensing solutions. The modeling component reverse engineers rules for placement of traffic regulators, allowing it to predict their locations and type based on map information. Where available, crowd-sourced vehicular GPS traces are incorporated into the prediction function to improve the results. The approach is evaluated across multiple cities and is shown to outperform both crowd-sourcing alone and map-based modeling alone. It achieves a prediction accuracy level above 97% in detecting the existence and determining the type of traffic regulators in the cities considered.
更多查看译文
关键词
intelligent transportation, traffic regulators, street maps, crowd-sensing
AI 理解论文
溯源树
样例
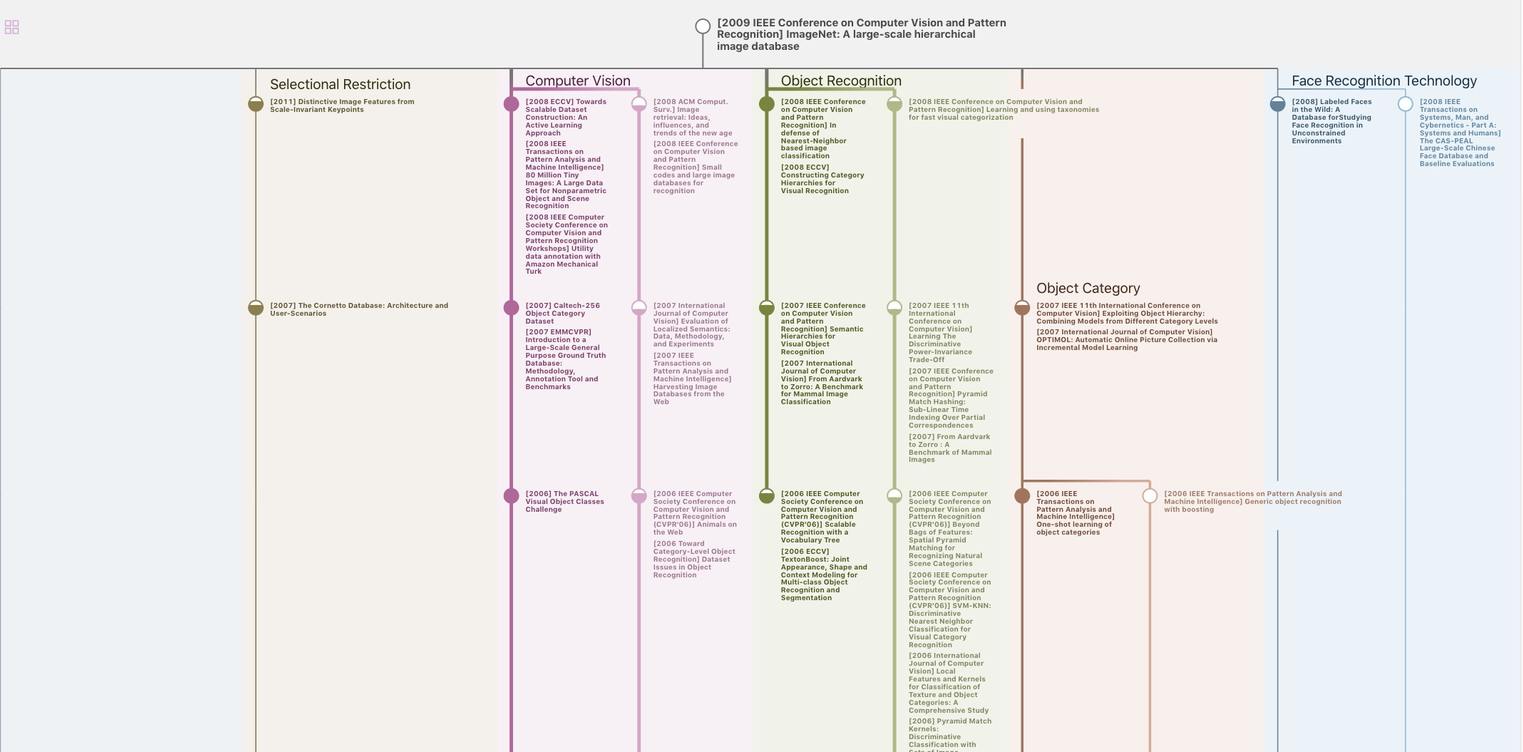
生成溯源树,研究论文发展脉络
Chat Paper
正在生成论文摘要