Using speaker group dependent modelling to improve fusion of fragmentary classifier decisions
CYBCONF(2013)
摘要
Current speech-controlled human computer interaction is purely based on spoken information. For a successful interaction, additional information such as the individual skills, preferences and actual affective state of the user are often mandatory. The most challenging of these additional inputs is the affective state, since affective cues are in general expressed very sparsely. The problem can be addressed in two ways. On the one hand, the recognition can be enhanced by making use of already available individual information. On the other hand, the recognition is aggravated by the fact that research is often limited to a single modality, which in real-life applications is critical since recognition may fail in case sensors do not perceive a signal. We address the problem by enhancing the acoustic recognition of the affective state by partitioning the user into groups. The assignment of a user to a group is performed at the beginning of the interaction, such that subsequently a specialized classifier model is utilized. Furthermore, we make use of several modalities, acoustics, facial expressions, and gesture information. The combination of decisions not affected by sensor failures from these multiple modalities is achieved by a Markov Fusion Network. The proposed approach is studied empirically using the LAST MINUTE corpus. We could show that compared to previous studies a significant improvement of the recognition rate can be obtained.
更多查看译文
关键词
Multimodal Pattern Recognition, Affect Recognition, Companion Systems, Human Computer Interaction
AI 理解论文
溯源树
样例
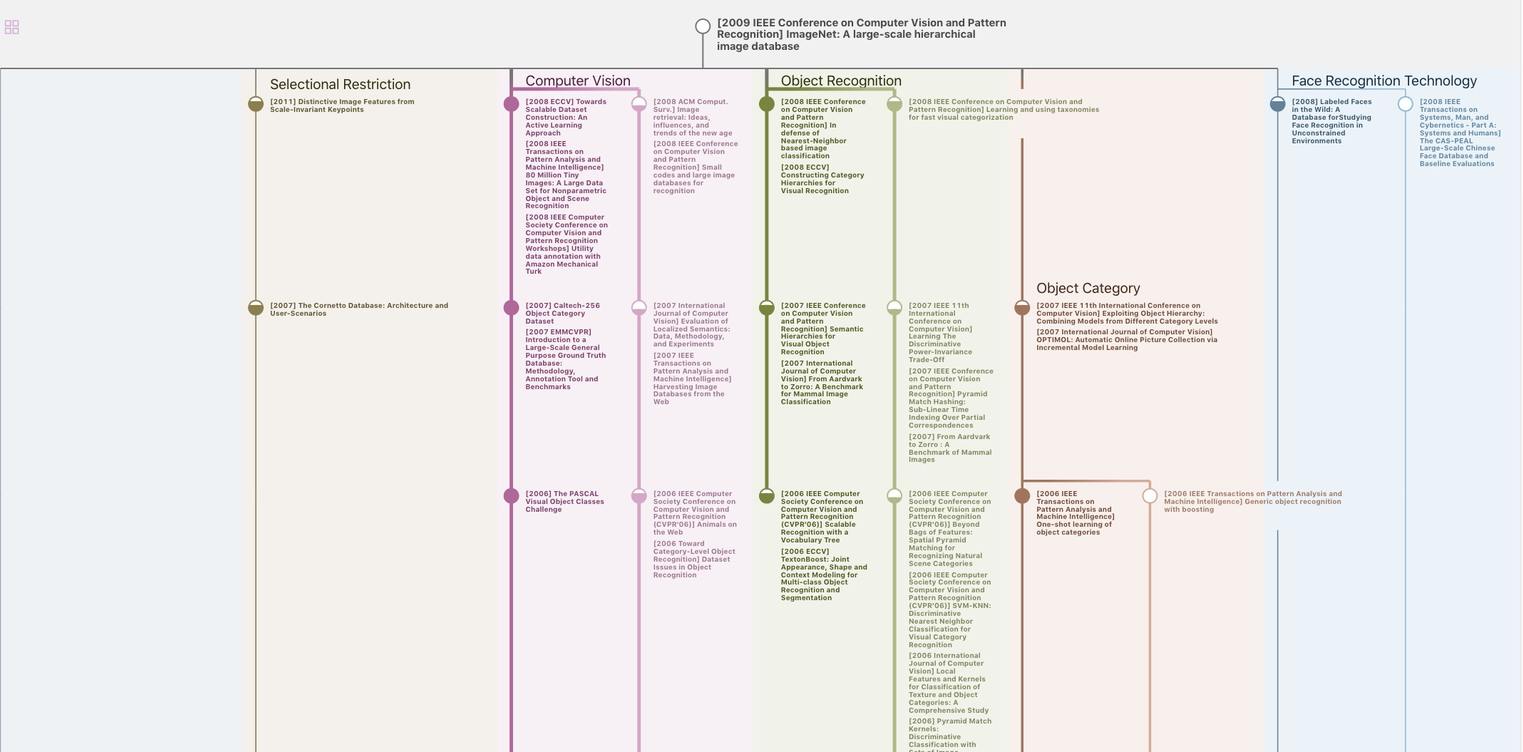
生成溯源树,研究论文发展脉络
Chat Paper
正在生成论文摘要