Att: Attention-Based Timbre Transfer
2020 INTERNATIONAL JOINT CONFERENCE ON NEURAL NETWORKS (IJCNN)(2020)
摘要
In this paper, we tackle the issue of timbre transfer on a given monophonic music sample. The objective is to change the timbre of source audio from one instrument to another while preserving features such as loudness, pitch, and rhythm. Existing approaches use image-to-image translation techniques on the entire region of time-frequency representations of the raw audio wave, which may lead to the addition of unwanted elements in the final audio waveform. We propose Attention-based Timbre Transfer (ATT), an attention-based pipeline for transferring timbre. To the best of our knowledge, ATT is the first approach which leverages attention for achieving timbre transfer. Further, ATT uses MelGAN for spectrogram inversion, which provides a fast and parallel alternative to other autoregressive music generation approaches, without compromising on the quality. ATT shows promising results, thus efficaciously transferring timbre with minimal offset to other physical characteristics.
更多查看译文
关键词
Generative Adversarial Networks, Timbre transfer, Style transfer, mel-frequency spectrogram
AI 理解论文
溯源树
样例
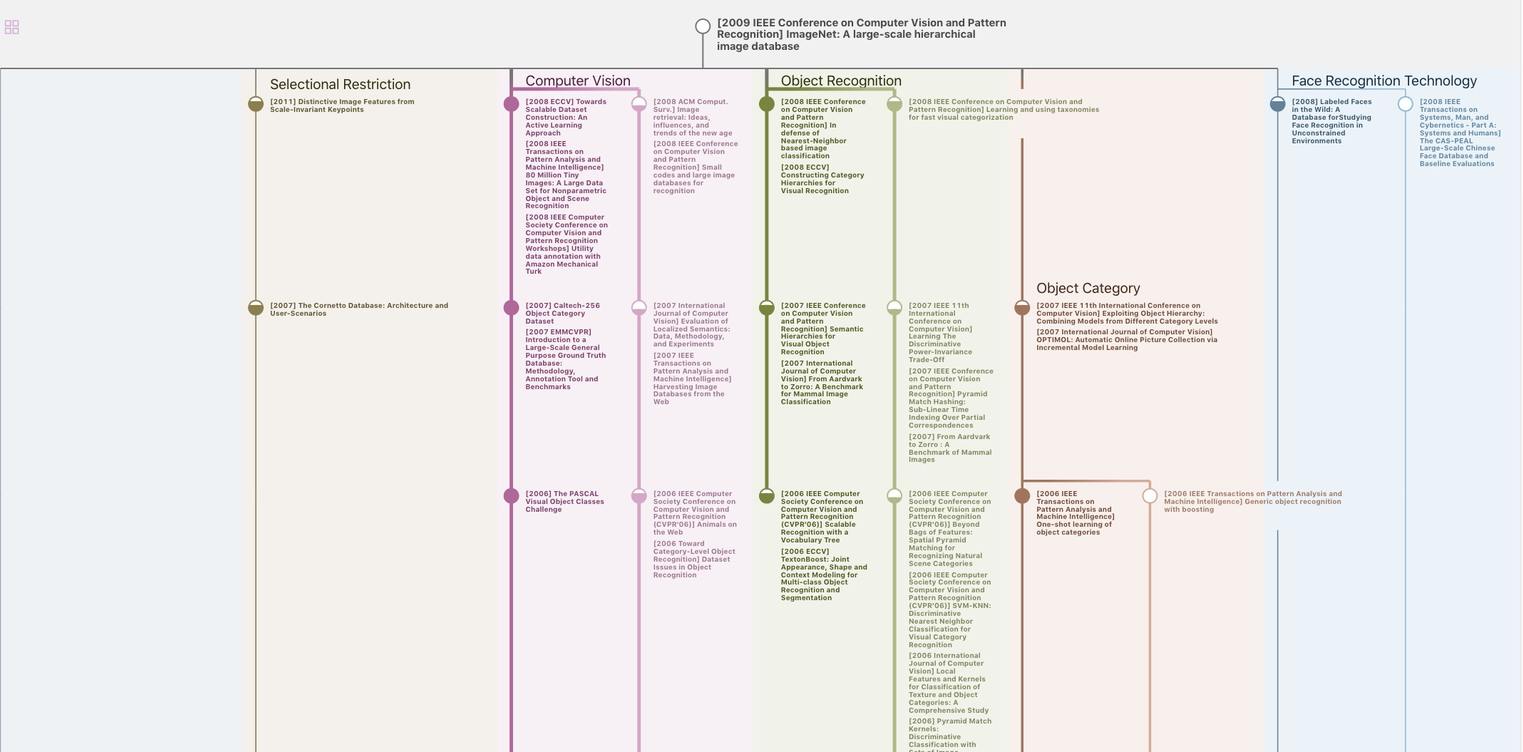
生成溯源树,研究论文发展脉络
Chat Paper
正在生成论文摘要