Let'S (Not) Stick Together: Pairwise Similarity Biases Cross-Validation In Activity Recognition
UbiComp '15: The 2015 ACM International Joint Conference on Pervasive and Ubiquitous Computing Osaka Japan September, 2015(2015)
摘要
The ability to generalise towards either new users or unforeseen behaviours is a key requirement for activity recognition systems in ubiquitous computing. Differences in recognition performance for the two application cases can be significant, and user-dependent performance is typically assumed to be an upper bound on performance. We demonstrate that this assumption does not hold for the widely used cross-validation evaluation scheme that is typically employed both during system bootstrapping and for reporting results. We describe how the characteristics of segmented time-series data render random cross-validation a poor fit, as adjacent segments are not statistically independent. We develop an alternative approach -meta-segmented cross validation - that explicitly circumvents this issue and evaluate it on two data-sets. Results indicate a significant drop in performance across a variety of feature extraction and classification methods if this bias is removed, and that prolonged, repetitive activities are particularly affected.
更多查看译文
关键词
Activity Recognition,Evaluation,Cross validation,Model selection
AI 理解论文
溯源树
样例
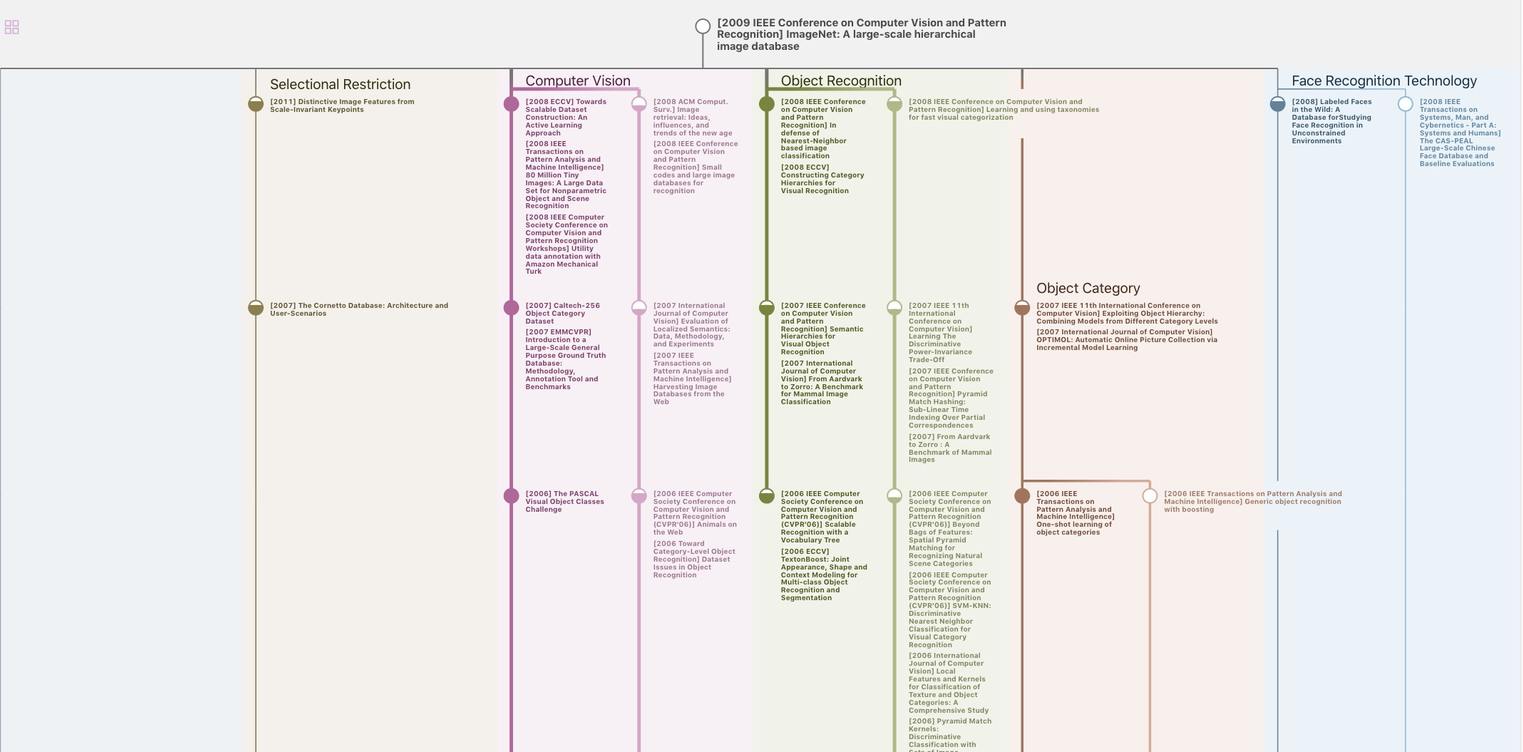
生成溯源树,研究论文发展脉络
Chat Paper
正在生成论文摘要