Model-Based Word Embeddings From Decompositions Of Count Matrices
PROCEEDINGS OF THE 53RD ANNUAL MEETING OF THE ASSOCIATION FOR COMPUTATIONAL LINGUISTICS AND THE 7TH INTERNATIONAL JOINT CONFERENCE ON NATURAL LANGUAGE PROCESSING, VOL 1(2015)
摘要
This work develops a new statistical understanding of word embeddings induced from transformed count data. Using the class of hidden Markov models (HMMs) underlying Brown clustering as a generative model, we demonstrate how canonical correlation analysis (CCA) and certain count transformations permit efficient and effective recovery of model parameters with lexical semantics. We further show in experiments that these techniques empirically outperform existing spectral methods on word similarity and analogy tasks, and are also competitive with other popular methods such as WORD2VEC and GLOVE.
更多查看译文
AI 理解论文
溯源树
样例
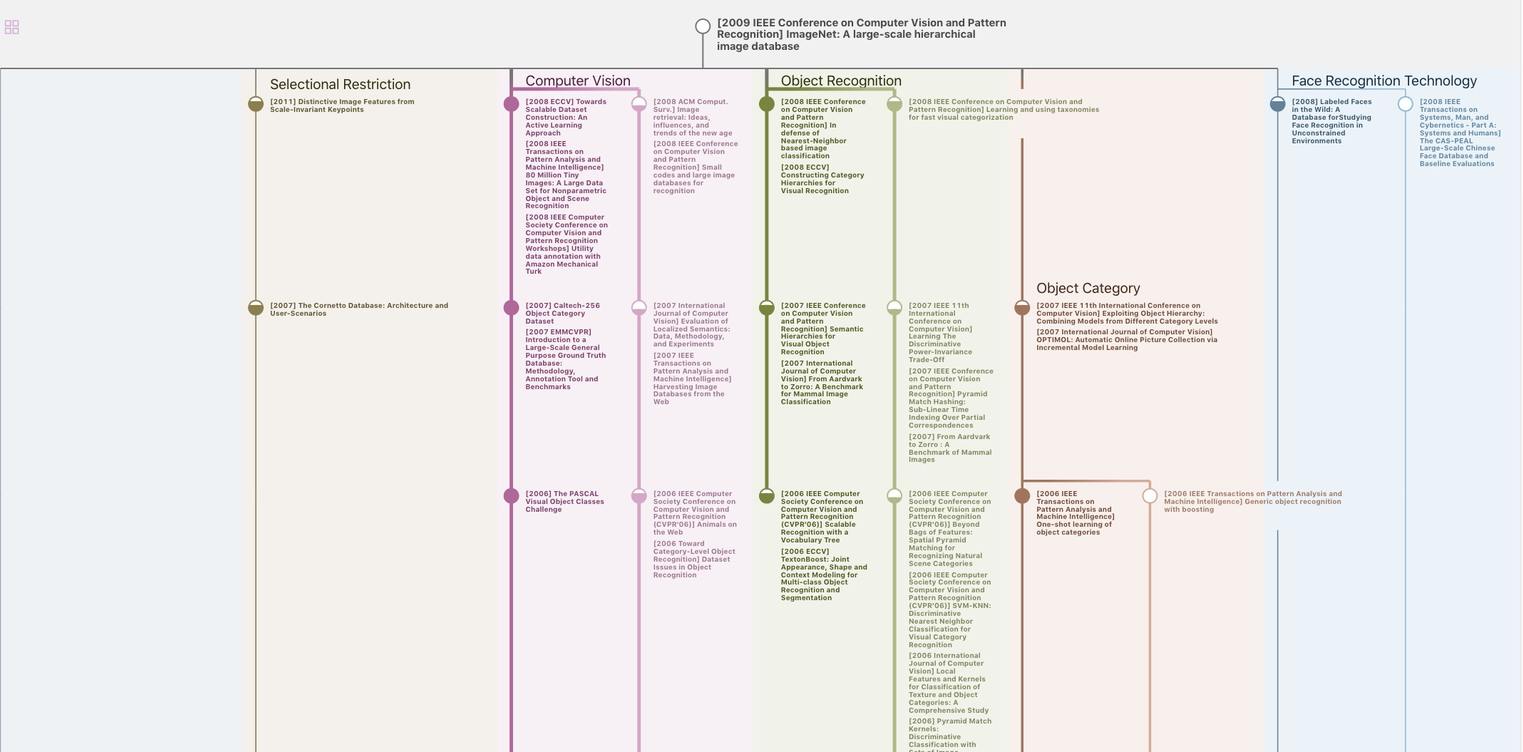
生成溯源树,研究论文发展脉络
Chat Paper
正在生成论文摘要